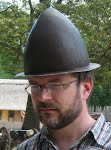
Brian Roark
Brian Roark is a computational linguist working on various topics in natural language processing. His research interests include: language modeling for automatic speech recognition, text entry and other applications; text normalization and transliteration; text entry, accessibility and augmentative and alternative communication (AAC).
Before joining Google, he was a faculty member for 9 years in the Center for Spoken Language Understanding (CSLU) at Oregon Health & Science University (OHSU) – part of what used to be the Oregon Graduate Institute (OGI). Before that, he was in the Speech Algorithms Department at AT&T Labs - Research from 2001–2004. He received his Ph.D. in the Department of Cognitive and Linguistic Sciences at Brown University in 2001.
More information, including publications, CV and other links, can be found at his external webpage here.
Before joining Google, he was a faculty member for 9 years in the Center for Spoken Language Understanding (CSLU) at Oregon Health & Science University (OHSU) – part of what used to be the Oregon Graduate Institute (OGI). Before that, he was in the Speech Algorithms Department at AT&T Labs - Research from 2001–2004. He received his Ph.D. in the Department of Cognitive and Linguistic Sciences at Brown University in 2001.
More information, including publications, CV and other links, can be found at his external webpage here.
Research Areas
Authored Publications
Sort By
Context-aware Transliteration of Romanized South Asian Languages
Christo Kirov
Computational Linguistics, 50 (2)(2024), 475–534
Preview abstract
While most transliteration research is focused on single tokens such as named entities -- e.g., transliteration of "અમદાવાદ" from the Gujarati script to the Latin script "Ahmedabad" -- the informal romanization prevalent in South Asia and elsewhere often requires transliteration of full sentences. The lack of large parallel text collections of full sentence (as opposed to single word) transliterations necessitates incorporation of contextual information into transliteration via non-parallel resources, such as via mono-script text collections. In this paper, we present a number of methods for improving transliteration in context for such a use scenario. Some of these methods in fact improve performance without making use of sentential context, allowing for better quantification of the degree to which contextual information in particular is responsible for system improvements. Our final systems, which ultimately rely upon ensembles including large pretrained language models finetuned on simulated parallel data, yield substantial improvements over the best previously reported results for full sentence transliteration from Latin to native script on all 12 languages in the Dakshina dataset (Roark et al. 2020), with an overall 4.8% absolute (27.1% relative) mean word-error rate reduction.
View details
Preview abstract
We examine whether large neural language models, trained on very large collections of varied English text, learn the potentially long-distance dependency of British versus American spelling conventions, i.e., whether spelling is consistently one or the other within model-generated strings. In contrast to long-distance dependencies in non-surface underlying structure (e.g., syntax), spelling consistency is easier to measure both in LMs and the text corpora used to train them, which can provide additional insight into certain observed model behaviors. Using a set of probe words unique to either British or American English, we first establish that training corpora exhibit substantial (though not total) consistency. A large T5 language model does appear to internalize this consistency, though only with respect to observed lexical items (not nonce words with British/American spelling patterns). We further experiment with correcting for biases in the training data by fine-tuning T5 on synthetic data that has been debiased, and find that finetuned T5 remains only somewhat sensitive to spelling consistency. Further experiments show GPT2 to be similarly limited.
View details
XTREME-UP: A User-Centric Scarce-Data Benchmark for Under-Represented Languages
Sebastian Ruder
Mihir Sanjay Kale
Shruti Rijhwani
Jean-Michel Sarr
Cindy Wang
John Wieting
Christo Kirov
Dana L. Dickinson
Bidisha Samanta
Connie Tao
David Adelani
Reeve Ingle
Dmitry Panteleev
Findings of the Association for Computational Linguistics: EMNLP 2023, Association for Computational Linguistics, Singapore, pp. 1856-1884
Preview abstract
Data scarcity is a crucial issue for the development of highly multilingual NLP systems. Yet for many under-represented languages (ULs) — languages for which NLP research is particularly far behind in meeting user needs — it is feasible to annotate small amounts of data. Motivated by this, we propose XTREME-UP, a benchmark defined by: its focus on the scarce-data scenario rather than zero-shot; its focus on user-centric tasks — tasks with broad adoption by speakers of high-resource languages; and its focus on under-represented languages where this scarce-data scenario tends to be most realistic. XTREME-UP evaluates the capabilities of language models across 88 under-represented languages over 9 key user-centric technologies including ASR, OCR, MT, and information access tasks that are of general utility. We create new datasets for OCR, autocomplete, semantic parsing, and transliteration, and build on and refine existing datasets for other tasks. XTREME-UP provides methodology for evaluating many modeling scenarios including text only, multi-modal (vision, audio, and text), supervised parameter tuning, and in-context learning. We evaluate commonly used models on the benchmark. We release all code and scripts to train and evaluate models.
View details
Beyond Arabic: Software for Perso-Arabic Script Manipulation
Raiomond Doctor
Proceedings of the 7th Arabic Natural Language Processing Workshop (WANLP2022) at EMNLP, Association for Computational Linguistics (ACL), Abu Dhabi, United Arab Emirates (Hybrid), pp. 381-387
Preview abstract
This paper presents an open-source software library that provides a set of finite-state transducer (FST) components and corresponding utilities for manipulating the writing systems of languages that use the Perso-Arabic script. The operations include various levels of script normalization, including visual invariance-preserving operations that subsume and go beyond the standard Unicode normalization forms, as well as transformations that modify the visual appearance of characters in accordance with the regional orthographies for ten contemporary languages from diverse language families. The library also provides simple FST-based romanization and transliteration. We additionally attempt to formalize the typology of Perso-Arabic characters by providing one-to-many mappings from Unicode code points to the languages that use them. While our work focuses on the Arabic script diaspora rather than Arabic itself, this approach could be adopted for any language that uses the Arabic script, thus providing a unified framework for treating a script family used by close to a billion people.
View details
Extensions to Brahmic script processing within the Nisaba library: new scripts, languages and utilities
Raiomond Doctor
Lawrence Wolf-Sonkin
Proceedings of the 13th Language Resources and Evaluation Conference.(LREC), European Language Resources Association (ELRA), 20-25 June, Marseille, France(2022), 6450‑6460
Preview abstract
The Brahmic family of scripts is used to record some of the most spoken languages in the world and is arguably the most diverse family of writing systems. In this work, we present several substantial extensions to Brahmic script functionality within the open-source Nisaba library of finite-state script normalization and processing utilities (Johny et. al. , 2021). First, we extend coverage from the original ten scripts to an additional ten scripts of South Asia and beyond, including some used to record endangered languages such as Dogri. Second, we augment the language layer so that scripts used by multiple languages in distinct ways can be processed correctly for more languages, such as the Bengali script when used for the low-resource language Santali. We document key changes to the finite-state engine required to support these new languages and scripts. Finally, we add new script processing utilities, including lightweight script-level reading normalization that (unlike existing visual normalization) does not preserve visual invariance, and a fixed-input transliteration mechanism specifically tailored to Brahmic text entry with ASCII characters.
View details
Criteria for Useful Automatic Romanization in South Asian Languages
Proceedings of the 13th Language Resources and Evaluation Conference.(LREC), European Language Resources Association (ELRA), 20-25 June, Marseille, France(2022), 6662‑6673
Preview abstract
This paper presents a number of possible criteria for systems that transliterate South Asian languages from their native scripts into the Latin script. This process is also known as romanization. These criteria are related to either fidelity to human linguistic behavior (pronunciation transparency, naturalness and conventionality) or processing utility for people (ease of input) as well as under-the-hood in systems (invertibility and stability across languages and scripts). When addressing these differing criteria several linguistic considerations, such as modeling of prominent phonological processes and their relation to orthography, need to be taken into account. We discuss these key linguistic details in the context of Brahmic scripts and languages that use them, such as Hindi and Malayalam. We then present the core features of several romanization algorithms, implemented in finite state transducer (FST) formalism, that address differing criteria. Implementation of these algorithms will be released as part of the Nisaba finite-state script processing library.
View details
Graphemic Normalization of the Perso-Arabic Script
Raiomond Doctor
Proceedings of Grapholinguistics in the 21st Century, 2022 (G21C, Grafematik), Paris, France
Preview abstract
Since its original appearance in 1991, the Perso-Arabic script representation in Unicode has grown from 169 to over 440 atomic isolated characters spread over several code pages representing standard letters, various diacritics and punctuation for the original Arabic and numerous other regional orthographic traditions (Unicode Consortium, 2021). This paper documents the challenges that Perso-Arabic presents beyond the best-documented languages, such as Arabic and Persian, building on earlier work by the expert community (ICANN, 2011, 2015). We particularly focus on the situation in natural language processing (NLP), which is affected by multiple, often neglected, issues such as the use of visually ambiguous yet canonically nonequivalent letters and the mixing of letters from different orthographies. Among the contributing conflating factors are the lack of input methods, the instability of modern orthographies (e.g., Aazim et al., 2009; Iyengar, 2018), insufficient literacy, and loss or lack of orthographic tradition (Jahani and Korn, 2013; Liljegren, 2018). We evaluate the effects of script normalization on eight languages from diverse language families in the Perso-Arabic script diaspora on machine translation and statistical language modeling tasks. Our results indicate statistically significant improvements in performance in most conditions for all the languages considered when normalization is applied. We argue that better understanding and representation of Perso-Arabic script variation within regional orthographic traditions, where those are present, is crucial for further progress of modern computational NLP techniques (Ponti et al., 2019; Conneau et al., 2020; Muller et al., 2021) especially for languages with a paucity of resources.
View details
Design principles of an open-source language modeling microservice package for AAC text-entry applications
9th Workshop on Speech and Language Processing for Assistive Technologies (SLPAT-2022), Association for Computational Linguistics (ACL), Dublin, Ireland, pp. 1-16
Preview abstract
We present MozoLM, an open-source language model microservice package intended for use in AAC text-entry applications, with a particular focus on the design principles of the library. The intent of the library is to allow the ensembling of multiple diverse language models without requiring the clients (user interface designers, system users or speech-language pathologists) to attend to the formats of the models. Issues around privacy, security, dynamic versus static models, and methods of model combination are explored and specific design choices motivated. Some simulation experiments demonstrating the benefits of personalized language model ensembling via the library are presented.
View details
Preview abstract
Psycholinguistic studies of human word processing and lexical access provide ample evidence of the preferred nature of word-initial versus word-final segments, e.g., in terms of attention paid by listeners (greater) or the likelihood of reduction by speakers (lower). This has led to the conjecture—as in Wedel et al. (2019b), but common elsewhere—that languages have evolved to provide more information earlier in words than later. Information-theoretic methods to establish such tendencies in lexicons have suffered from several methodological shortcomings that leave open the question of whether this high word-initial informativeness is actually a property of the lexicon or simply an artefact of the incremental nature of recognition. In this paper, we point out the confounds in existing methods for comparing the informativeness of segments early in the word versus later in the word, and present several new measures that avoid these confounds. When controlling for these confounds, we still find evidence across hundreds of languages that indeed there is a cross-linguistic tendency to front-load information in words.
View details
Approximating probabilistic models as weighted finite automata
Vlad Schogol
Computational Linguistics, 47(2021), pp. 221-254
Preview abstract
Weighted finite automata (WFA) are often used to represent probabilistic models, such as n-
gram language models, since they are efficient for recognition tasks in time and space. The
probabilistic source to be represented as a WFA, however, may come in many forms. Given
a generic probabilistic model over sequences, we propose an algorithm to approximate it as a
weighted finite automaton such that the Kullback-Leiber divergence between the source model
and the WFA target model is minimized. The proposed algorithm involves a counting step and a
difference of convex optimization step, both of which can be performed efficiently. We demonstrate
the usefulness of our approach on various tasks, including distilling n-gram models from neural
models, building compact language models, and building open-vocabulary character models. The
algorithms used for these experiments are available in an open-source software library.
View details