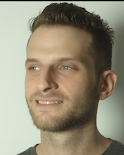
Julian Martin Eisenschlos
NLP Researcher based in Zurich. I work on natural language understanding, with a focus on text generation, semantic parsing and multilinguality. Co-founded botmaker, a leading conversational AI platform in Latin America. Previously worked on NLP research at ASAPP and knowledge base fusion at Facebook.
Research Areas
Authored Publications
Google Publications
Other Publications
Sort By
Chain-of-Table: Evolves Tables in the LLM Reasoning Chain for Table Understanding
Zilong Wang
Hao Zhang
Chun-Liang Li
Jingbo Shang
ICLR (2024)
Preview abstract
Table-based reasoning with large language models (LLMs) is a promising direction to tackle many table understanding tasks, such as table-based question answering and fact verification. Compared with generic reasoning, table-based reasoning requires the extraction of underlying semantics from both free-form questions and semi-structured tabular data. Chain-of-Thought and its similar approaches incorporate the reasoning chain in the form of textual context, but it is still an open question how to effectively leverage tabular data in the reasoning chain. We propose the Chain-of-Table framework, where tabular data is explicitly used in the reasoning chain as a proxy for intermediate thoughts. Specifically, we guide LLMs using in-context learning to iteratively generate operations and update the table to represent a tabular reasoning chain. LLMs can therefore dynamically plan the next operation based on the results of the previous ones. This continuous evolution of the table forms a chain, showing the reasoning process for a given tabular problem. The chain carries structured information of the intermediate results, enabling more accurate and reliable predictions. Chain-of-Table achieves new state-of-the-art performance on WikiTQ, FeTaQA, and TabFact benchmarks across multiple LLM choices.
View details
Preview abstract
A hallmark of modern large language models (LLMs) is their impressive general zero-shot and few-shot abilities, often elicited through in-context learning (ICL) via prompting. However, while highly coveted and being the most general, zero-shot performances in LLMs are still typically weaker due to the lack of guidance and the difficulty of applying existing automatic prompt design methods in general tasks when ground-truth labels are unavailable. In this study, we address this by presenting Universal Self-Adaptive Prompting (USP), an automatic prompt design approach specifically tailored for zero-shot learning (while compatible with few-shot). Requiring only a small amount of unlabeled data and an inference-only LLM, USP is highly versatile: to achieve universal prompting, USP categorizes a possible NLP task into one of the three possible task types and then uses a corresponding selector to select the most suitable queries and zero-shot model-generated responses as pseudo-demonstrations, thereby generalizing ICL to the zero-shot setup in a fully automated way. We evaluate USP with PaLM and PaLM 2 models and demonstrate performances that are considerably stronger than standard zero-shot baselines and often comparable to or even superior to few-shot baselines across more than 40 natural language understanding, natural language generation, and reasoning tasks.
View details
DIFFQG: Generating Questions to Summarize Factual Changes
Palak Jain
Michael Zhang
Eunsol Choi
Bhuwan Dhingra
European Association for Computational Linguistics EACL (2023)
Preview abstract
Question Generation has been emerging as new method to improve QA systems and represent factual information in text.
However, despite the rash of new work on the topic, there is still no obvious method to evaluate such systems.
Here we present DiffQG, a method to evaluate the precision and recall of question generation systems.
DiffQG consists of expert labeled annotations, focusing on the particularly challenging task of generating questions from similar pieces of text.
Given an edit to a Wikipedia passage and a noun phrase, annotators wrote questions that are answered by one passage but answered differently or not at all by the other.
These questions are intended to be both unambiguous and information-seeking, pushing the bounds of current question generation systems' capabilities.
Moreover, as annotators also marked when no such question exists, it serves as a new evaluation for difference detection, which also lacks evaluations with as much diversity as DiffQG.
We hope that this dataset will be of value to researchers as they seek to improve such systems for a variety of purposes.
View details
Preview abstract
Prior work on constructing challenging tabular inference data centered primarily on human annotation or automatic synthetic generation. Both techniques have their own set of issues. Human annotation, despite its diversity and superior reasoning, struggles from scaling concerns. Synthetic data, on the other hand, despite its scalability, suffers from lack of linguistic and reasoning diversity. In this paper, we address both of these concerns by presenting a recasting approach that semi-automatically generates tabular NLI instances. We transform the table2text dataset ToTTo (Parikh et al., 2020) into a tabular NLI dataset using our proposed framework. We demonstrate the use of our recasted data as an evaluation benchmark as well as augmentation data to improve performance on TabFact (Chen et al., 2020b). Furthermore, we test the effectiveness of models trained on our data on the TabFact benchmark in the zero-shot scenario.
View details
Preview abstract
By nature of the cost and time required to train Large Language Models (LLMs), the embedded knowledge within is usually frozen at the moment their training data is collected. As a result, LLMs have been shown to suffer from diachronic degradation. The in-context learning paradigm can provide a workaround for this limitation by supplying relevant information at inference time. We introduce a new benchmark to evaluate LLMs for one particular but critical aspect of diachronic change: language acquisition. To that end, we rewrite Winograd-style co-reference resolution problems by replacing a word for a new synthetic but plausible English word. The meaning of the word is given to the model in the prompt via a dictionary definition. We show that the accuracy of LLMs compared to the original Winograd tasks decreases radically in our benchmark and we believe this serves as a measure of progress for future models.
View details
Preview abstract
Language models (LMs) trained on raw texts have no access to the real physical world environment. Gordon and Van Durme (2013) argue that they thus suffer from reporting bias, meaning that texts rarely report commonsensical facts about the world but more frequently talk about non-commonsenscical facts/events. If LMs naively overfit to the co-occurrence statistics of training corpora, they learn a biased view of the physical world. While prior studies have repeatedly verified that LMs of smaller scales (e.g. RoBERTa, GTP-2) amplifies reporting bias, it remains unknown whether such trends continue when models are scaled up. We investigate reporting bias in larger language models (LLMs) such as PaLM and GPT-3. Specifically, we query LLMs for the colour of objects, using colour as a representative property for visual commonsense. Surprisingly, we found that LLMs significantly outperform smaller LMs on answering queries about an object's typical colour. We find that LLMs' predictions deviate from corpus co-occurrence statistics induced from resources such as Google Books Ngram and are closer to human judgement. We believe this serves as evidence that larger LMs can overcome reporting bias, rather than showing an inverse scaling function as previously suggested.
View details
Table-To-Text generation and pre-training with TabT5
Ewa Andrejczuk
Findings of EMNLP (2022)
Preview abstract
Encoder-only transformer models have been successfully applied to different table understanding tasks, as in TAPAS (Herzig et al., 2020). A major limitation of these architectures is that they are constrained to classification-like tasks such as cell selection or entailment detection. We present TABT5, an encoder-decoder model that generates natural language text based on tables and textual inputs. TABT5, overcomes the encoder-only limitation by incorporating a decoder component and leverages the input structure with table specific embeddings as well as pre-training. TABT5 achieves new state-of-the-art results on several domains, including spreadsheet formula prediction (15% increase in sequence accuracy), question answering (10% increase in sequence accuracy) and data-to-text generation (2% increas in BLEU).
View details
Preview abstract
We propose a benchmark to assess the capability of large language models to reason with metaphor.
Our benchmark combines the previously isolated topics of metaphor detection and commonsense reasoning into a single task that requires a model to make inferences by accurately selecting between the literal and metaphorical register. We examine the performance of state-of-the-art pretrained models on forced-choice tasks and find a large discrepancy between small and very large models, going from chance- to human-level performance. However, upon examining the generative performance of the largest model, we find that there is still a gap to bridge before human performance is reached in a more natural conversational setting.
View details
MatCha: Enhancing Visual Language Pretraining with Math Reasoning and Chart Derendering
Chenxi Pang
Mandar Joshi
Nigel Collier
Under review (2022)
Preview abstract
Visual language data such as plots, charts, and infographics are ubiquitous in the human world. However, state-of-the-art vision-language models do not perform well on these data. We propose a set of pretraining tasks to enhance visual language models' capabilities in jointly modeling charts/plots and language data. We initialize with Pix2Struct, a recently proposed image-to-text visual language model and continue pretraining with our proposed objectives. We argue that numerical reasoning and plot deconstruction enable a model with the key capabilities of (1) extracting key information and (2) reasoning on the extracted information. On standard benchmarks such as PlotQA and ChartQA, our continually pretrained MatCha model outperforms state-of-the-art methods by as much as ~20%. We also examine how well does MatCha pretraining transfer to domains such as screenshot, textbook, and poster figures. We observe improvement over the base Pix2Struct checkpoint by 1.2% on average, verifying the usefulness of MatCha pretraining on broader visual language tasks.
View details
DePlot: One-shot visual language understanding by plot-to-text translation
Chenxi Pang
Mandar Joshi
Nigel Collier
Under review (2022)
Preview abstract
Visual language such as charts and plots are ubiquitous in the human world. Comprehending plots and charts requires strong reasoning skills. Prior state-of-the-art models are end-to-end multimodal Transformers pretrained with dedicated plot derendering and numerical reasoning objectives. However, the models reasoning capabilities still fall short and will generally fail on complex queries.
In this paper, we decompose the multimodal reasoning problem into first, a modality conversion problem from image to text, then a purely textual reasoning problem. Through combining a pretrained image-to-text model and an LLM for the task of chart/figure reasoning. Compared with a SOTA model finetuned on >10k data points, our plug-and-play model DePlot-LLM achieves >20% improvement over finetuned SOTA with just one-shot prompting.
View details
Preview abstract
Recent advances in open-domain QA have led to strong models based on dense retrieval, but only focused on retrieving textual passages. In this work, we tackle open-domain QA over tables for the first time, and show that retrieval can be improved by a retriever designed to handle tabular context. We present an effective pre-training procedure for our retriever and improve retrieval quality with mined hard negatives. As relevant datasets are missing, we extract a subset of NATURAL QUESTIONS (Kwiatkowski et al., 2019) into a Table QA dataset. We find that our retriever improves retrieval results from 72.0 to 81.1 recall@10 and end-to-end QA results from 33.8 to 37.7 exact match, over a BERT based retriever
View details
MATE: Multi-view Attention for Table Transformer Efficiency
Maharshi Gor
Thomas Müller
Proceedings of the 2021 Conference on Empirical Methods in Natural Language Processing (EMNLP), Association for Computational Linguistics
Preview abstract
This work presents a sparse-attention Transformer architecture for modeling documents that contain large tables. Tables are ubiquitous on the web, and are rich in information. However, more than 20% of relational tables on the web have 20 or more rows (Cafarella et al., 2008), and these large tables present a challenge for current Transformer models, which are typically limited to 512 tokens. Here we propose MATE, a novel Transformer architecture designed to model the structure of web tables. MATE uses sparse attention in a way that allows heads to efficiently attend to either rows or columns in a table. This architecture scales linearly with respect to speed and memory, and can handle documents containing more than 8000 tokens with current accelerators. MATE also has a more appropriate inductive bias for tabular data, and sets a new state-of-the-art for three table reasoning datasets. For HybridQA (Chen et al., 2020b), a dataset that involves large documents containing tables, we improve the best prior result by 19 points.
View details
DoT: An efficient Double Transformer for NLP tasks with tables
Thomas Müller
Findings of the Association for Computational Linguistics: ACL 2021
Preview abstract
Transformer-based approaches have been successfully used to obtain state-of-the-art accuracy on natural language processing (NLP) tasks with semi-structured tables. These model architectures are typically deep, resulting in slow training and inference, especially for long inputs. To improve efficiency while maintaining a high accuracy, we propose a new architecture, DoT, a double transformer model, that decomposes the problem into two sub-tasks: A shallow pruning transformer that selects the top-K tokens, followed by a deep task-specific transformer that takes as input those K tokens. Additionally, we modify the task-specific attention to incorporate the pruning scores. The two transformers are jointly trained by optimizing the task-specific loss. We run experiments on three benchmarks, including entailment and question-answering. We show that for a small drop of accuracy, DoT improves training and inference time by at least 50%. We also show that the pruning transformer effectively selects relevant tokens enabling the end-to-end model to maintain similar accuracy as slower baseline models. Finally, we analyse the pruning and give some insight into its impact on the task model.
View details
Adapting Language Models to Temporal Knowledge
Bhuwan Dhingra
Transactions of the ACL (2021)
Preview abstract
It is only a matter of time before facts become out of date: from the name of \abr{POTUS} to the basketball team Lebron James plays for.
This continuously limits the usefulness of previously collected datasets and language models (LMs) trained on them.
This problem is exacerbated as LMs are used in the closed book question answering setting,
where the pretraining data must contain the facts for the model to remember within its fixed parameters.
A frequent paradigm is to update or refresh the dataset every so often, then retrain models with the new data: this is costly, but does it work?
In this paper, we introduce a diagnostic dataset for probing LMs for factual knowledge that changes over time.
Using it we show that models trained only on the most recent slice of data perform
worse on questions about the past than models trained on uniform data across time,
while being better on current and future questions.
Moreover, we propose jointly modeling text with the time it was created
and show that this improves memorization of previous facts,
as well as reasoning about the uncertainty around future facts.
We also show that models trained with temporal context allow for efficient refreshes as
new data arrives without the need of retraining from scratch.
View details
TAPAS at SemEval-2021 Task 9: Reasoning over tables with intermediate pre-training
Thomas Müller
Proceedings of the Fifteenth Workshop on Semantic Evaluation (2021)
Preview abstract
We present the TAPAS contribution to the Shared Task on Statement Verification and Evidence Finding with Tables (SemEval 2021 Task 9, Wang et al. (2021)). SEM TAB FACT Task A is a classification task of recognizing if a statement is entailed, neutral or refuted by the content of a given table. We adopt the binary TAPAS model of Eisenschlos et al. (2020) to this task. We learn two binary classification models: A first model to predict if a statement is neutral or non-neutral and a second one to predict if it is entailed or refuted. As the shared task training set contains only entailed or refuted examples, we generate artificial neutral examples to train the first model. Both models are pre-trained using a MASKLM objective, intermediate counter-factual and synthetic data (Eisenschlos et al., 2020) and TABFACT (Chen et al., 2020), a large table entailment dataset. We find that the artificial neutral examples are somewhat effective at training the first model, achieving 68.03 test F1 versus the 60.47 of a majority baseline. For the second stage, we find that the pre-training on the intermediate data and TABFACT improves the results over MASKLM pre-training (68.03 vs 57.01).
View details
Fool Me Twice: Entailment from Wikipedia Gamification
Bhuwan Dhingra
Jordan Boyd-Graber
NAACL (2021)
Preview abstract
We release FoolMeTwice (FM2 for short), a large dataset of challenging entailment pairs collected through a fun multi-player game. Gamification encourages adversarial examples, drastically lowering the number of examples that can be solved using "shortcuts" compared to other popular entailment datasets. Players are presented with two tasks. The first task asks the player to write a plausible claim based on the evidence from a Wikipedia page. The second one shows two plausible claims written by other players, one of which is false, and the goal is to identify it before the time runs out. Players "pay" to see clues retrieved from the evidence pool: the more evidence the player needs, the harder the claim. Game-play between motivated players leads to diverse strategies for crafting claims, such as temporal inference and diverting to unrelated evidence, and results in higher quality data for the entailment and evidence retrieval tasks. We open source the dataset and the game code.
View details
SoftSort: A Continuous Relaxation for the argsort Operator
Sebastian Prillo
Proceedings of the 37th International Conference on Machine Learning, PMLR (2020)
Preview abstract
While sorting is an important procedure in computer science, the argsort operator - which takes as input a vector and returns its sorting permutation - has a discrete image and thus zero gradients almost everywhere. This prohibits end-to-end, gradient-based learning of models that rely on the argsort operator. A natural way to overcome this problem is to replace the argsort operator with a continuous relaxation. Recent work has shown a number of ways to do this, but the relaxations proposed so far are computationally complex. In this work we propose a simple continuous relaxation for the argsort operator which has the following qualities: it can be implemented in three lines of code, achieves state-of-the-art performance, is easy to reason about mathematically - substantially simplifying proofs - and is faster than competing approaches. We open source the code to reproduce all of the experiments and results.
View details
Preview abstract
Table entailment, the binary classification task of finding if a sentence is supported or refuted by the content of a table, requires understanding language and table structure as well as numerical and discrete reasoning. While there is extensive work on textual entailment, table entailment is less well studied. We adapt TAPAS (Herzig et al., 2020), a table-based BERT model, to recognize entailment.
Motivated by the benefits of data augmentation, we create a balanced dataset of millions of automatically created training examples which are learned in an intermediate step prior to fine-tuning. This new data is not only useful for table entailment, but also for SQA (Iyyer et al., 2017), a sequential table QA task. To be able to use long examples as input of BERT models, we evaluate table pruning techniques as a pre-processing step to drastically improve the training and prediction efficiency at a moderate drop in accuracy. The different methods set the new state0o-of-the-art on the TabFact (Chen et al., 2020) and SQA datasets.
View details
Tapas: Weakly Supervised Table Parsing via Pre-training
Thomas Müller
Proceedings of the 58th Annual Meeting of the Association for Computational Linguistics (Volume 1: Long Papers), Association for Computational Linguistics, Seattle, Washington, United States (2020)
Preview abstract
Answering natural language questions over tables is usually seen as a semantic parsing task. To alleviate the collection cost of full logical forms, one popular approach focuses on weak supervision consisting of denotations instead of logical forms. However, training semantic parsers from weak supervision poses difficulties, and in addition, the generated logical forms are only used as an intermediate step prior to retrieving the denotation. In this paper, we present TAPAS, an approach to question answering over tables without generating logical forms. TAPAS trains from weak supervision, and predicts the denotation by selecting table cells and optionally applying a corresponding aggregation operator to such selection. TAPAS extends BERT's architecture to encode tables as input, initializes from an effective joint pre-training of text segments and tables crawled from Wikipedia, and is trained end-to-end. We experiment with three different semantic parsing datasets, and find that TAPAS outperforms or rivals semantic parsing models by improving state-of-the-art accuracy on SQA from 55.1 to 67.2 and performing on par with the state-of-the-art on WIKISQL and WIKITQ, but with a simpler model architecture. We additionally find that transfer learning, which is trivial in our setting, from WIKISQL to WIKITQ, yields 48.7 accuracy, 4.2 points above the state-of-the-art.
View details
No Results Found