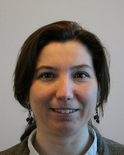
Yasemin Altun
Yasemin Altun is a Research Scientist at Google working on natural language understanding. She received her PhD from Brown University. Before joining Google, she was a faculty member in Toyota Technological Institute, Chicago and Max Planck Institute for Biological Cybernetics, Tuebingen.
Research Areas
Authored Publications
Google Publications
Other Publications
Sort By
DePlot: One-shot visual language understanding by plot-to-text translation
Chenxi Pang
Mandar Joshi
Nigel Collier
Under review (2022)
Preview abstract
Visual language such as charts and plots are ubiquitous in the human world. Comprehending plots and charts requires strong reasoning skills. Prior state-of-the-art models are end-to-end multimodal Transformers pretrained with dedicated plot derendering and numerical reasoning objectives. However, the models reasoning capabilities still fall short and will generally fail on complex queries.
In this paper, we decompose the multimodal reasoning problem into first, a modality conversion problem from image to text, then a purely textual reasoning problem. Through combining a pretrained image-to-text model and an LLM for the task of chart/figure reasoning. Compared with a SOTA model finetuned on >10k data points, our plug-and-play model DePlot-LLM achieves >20% improvement over finetuned SOTA with just one-shot prompting.
View details
Table-To-Text generation and pre-training with TabT5
Ewa Andrejczuk
Findings of EMNLP (2022)
Preview abstract
Encoder-only transformer models have been successfully applied to different table understanding tasks, as in TAPAS (Herzig et al., 2020). A major limitation of these architectures is that they are constrained to classification-like tasks such as cell selection or entailment detection. We present TABT5, an encoder-decoder model that generates natural language text based on tables and textual inputs. TABT5, overcomes the encoder-only limitation by incorporating a decoder component and leverages the input structure with table specific embeddings as well as pre-training. TABT5 achieves new state-of-the-art results on several domains, including spreadsheet formula prediction (15% increase in sequence accuracy), question answering (10% increase in sequence accuracy) and data-to-text generation (2% increas in BLEU).
View details
MatCha: Enhancing Visual Language Pretraining with Math Reasoning and Chart Derendering
Chenxi Pang
Mandar Joshi
Nigel Collier
Under review (2022)
Preview abstract
Visual language data such as plots, charts, and infographics are ubiquitous in the human world. However, state-of-the-art vision-language models do not perform well on these data. We propose a set of pretraining tasks to enhance visual language models' capabilities in jointly modeling charts/plots and language data. We initialize with Pix2Struct, a recently proposed image-to-text visual language model and continue pretraining with our proposed objectives. We argue that numerical reasoning and plot deconstruction enable a model with the key capabilities of (1) extracting key information and (2) reasoning on the extracted information. On standard benchmarks such as PlotQA and ChartQA, our continually pretrained MatCha model outperforms state-of-the-art methods by as much as ~20%. We also examine how well does MatCha pretraining transfer to domains such as screenshot, textbook, and poster figures. We observe improvement over the base Pix2Struct checkpoint by 1.2% on average, verifying the usefulness of MatCha pretraining on broader visual language tasks.
View details
Translate & Fill: Improving Zero-Shot Multilingual Semantic Parsing by Generating Synthetic Data
Zhongdi Qu
Proceedings of the 2021 Conference on Empirical Methods in Natural Language Processing (Findings), Association for Computational Linguistics (2021) (to appear)
Preview abstract
While multilingual pretrained language models (LMs) fine-tuned on a single language have shown substantial cross-lingual task transfer capabilities, there is still a wide performance gap in semantic parsing tasks when target language supervision is available. In this paper, we propose a novel Translate-and-Fill (TaF) method for producing silver training data for a multilingual semantic parser. This method simplifies the popular Translate-Align-Project (TAP) pipeline and consists of a sequence-to-sequence filler model that constructs a full parse conditioned on an utterance and a view of the same parse. Our filler is trained on English data only but can accurately complete instances in other languages (i.e., translations of the English training utterances), in a zero-shot fashion. Experimental results on multiple multilingual semantic parsing datasets show that high-capacity multilingual pretrained LMs have remarkable zero-shot performance and with the help of our synthetic data, they reach competitive accuracy compared to similar systems which rely on traditional alignment techniques.
View details
Preview abstract
Semantic parsing maps natural language utterances into structured meaning representations. We present an approach that uses a Graph Neural Network (GNN) architecture to incorporate information about relevant entities and their relations during parsing. Combined with a decoder copy mechanism, this approach also provides a conceptually simple mechanism to generate logical forms with entities. We demonstrate that this approach is competitive with state-of-the-art across several tasks without pre-training, and outperforms existing approaches when combined with BERT pre-training.
View details
Answering Conversational Questions on Structured Data without Logical Forms
Thomas Müller
Proceedings of the 2019 Conference on Empirical Methods in Natural Language Processing, Association for Computational Linguistics (2019)
Preview abstract
We present a novel approach to answering sequential questions based on structured objects such as knowledge bases or tables without using a logical form as an intermediate representation. We encode tables as graphs using a graph neural network model based on the Transformer architecture. The answers are then selected from the encoded graph using a pointer network. This model is appropriate for processing conversations around structured data, where the attention mechanism that selects the answer to a question can also be used to resolve conversational references. We demonstrate the validity of this approach with competitive results on the Sequential Question Answering task (SQA) (Iyyer et al., 2017).
View details
Overcoming the Lack of Parallel Data in Sentence Compression
Proceedings of the 2013 Conference on Empirical Methods in Natural Language Processing (EMNLP '13), pp. 1481-1491
Preview abstract
A subset of the described data (10,000 sentence & extracted headlines pairs, with source URL and annotations) is available for download.
View details
Exponential Families for Conditional Random Fields
Semi-Markov Models for Sequence Segmentation
Transductive Gaussian Process Regression with Automatic Model Selection
Unifying Divergence Minimization and Statistical Inference Via Convex Duality
Support vector machine learning for interdependent and structured output spaces
Exponential Families for Conditional Random Fields
Gaussian process classification for segmenting and annotating sequences
Hidden Markov Support Vector Machines
Discriminative Learning for Label Sequences via Boosting