Publications
Our teams aspire to make discoveries that impact everyone, and core to our approach is sharing our research and tools to fuel progress in the field.
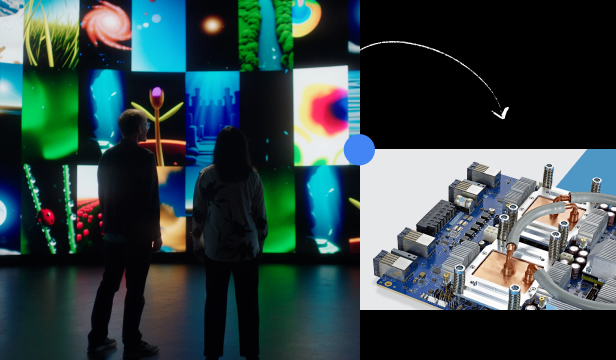
Our teams aspire to make discoveries that impact everyone, and core to our approach is sharing our research and tools to fuel progress in the field.
Sort By
1 - 15 of 10129 publications
Batch Calibration: Rethinking Calibration For In-Context Learning And Prompt Engineering
Lev Proleev
Diana Mincu
International Conference on Learning Representations (ICLR) (2024)
Preview abstract
Prompting and in-context learning (ICL) have become efficient learning paradigms for large language models (LLMs). However, LLMs suffer from prompt brittleness and various bias factors in the prompt, including but not limited to the formatting, the choice verbalizers, and the ICL examples. To address this problem that results in unexpected performance degradation, calibration methods have been developed to mitigate the effects of these biases while recovering LLM performance. In this work, we first conduct a systematic analysis of the existing calibration methods, where we both provide a unified view and reveal the failure cases. Inspired by these analyses, we propose Batch Calibration (BC), a simple yet intuitive method that controls the contextual bias from the batched input, unifies various prior approaches, and effectively addresses the aforementioned issues. BC is zero-shot, inference-only, and incurs negligible additional costs. In the few-shot setup, we further extend BC to allow it to learn the contextual bias from labeled data. We validate the effectiveness of BC with PaLM 2-(S, M, L) and CLIP models and demonstrate state-of-the-art performance over previous calibration baselines across more than 10 natural language understanding and image classification tasks.
View details
Computational Methodologies for Understanding, Automating, and Evaluating User Interfaces
Yuwen Lu
Yue Jiang
Christof Lutteroth
Toby Jia-Jun Li
Jeffery Nichols
Wolfgang Stuerzlinger
Preview abstract
Building on the success of the first two workshops on user interfaces (UIs) at CHI 2022 and CHI 2023, this workshop aims to advance the research field by further exploring current research trends, such as applying large language models and visual language models. Previous work has explored computational approaches to understanding and adapting UIs using constraint-based optimization models and machine learning-based data-driven approaches. In addition to further delving into these established UI research areas, we aim to trigger the exploration into the application of the latest advancements in general-purpose large language and vision-language models within the UI domain. We will encourage participants to explore novel methods for understanding, automating, and evaluating UIs. The proposed workshop seeks to bring together academic researchers and industry practitioners interested in computational approaches for UIs to discuss the needs and opportunities for future user interface algorithms, models, and applications.
View details
Preview abstract
Simple, sufficient explanations
furnished by short decision lists
can be useful for guiding stakeholder actions.
Unfortunately, this transparency can come at the expense
of the higher accuracy enjoyed by black box methods,
like deep nets.
To date, practitioners typically either (i) insist on the simpler model, forsaking accuracy; or (ii) insist on maximizing accuracy, settling for post-hoc explanations of dubious faithfulness.
In this paper, we propose a hybrid \emph{partially interpretable model} that represents a compromise between the two extremes.
In our setup, each input is first processed by a decision list that can either execute a decision or abstain,
handing off authority to the opaque model.
The key to optimizing the decision list is to optimally
trade off the accuracy of the composite system
against coverage (the fraction of the population
that receives explanations).
We contribute a new principled algorithm for constructing partially interpretable decision lists,
providing theoretical guarantees
addressing both interpretability and accuracy.
As an instance of our result, we prove
that when the optimal decision list has length $k$, coverage $c$, and $b$ mistakes,
our algorithm will generate a decision list
that has length no greater than $4k$,
coverage at least $c/2$,
and makes at most $4b$ mistakes.
Finally, we empirically validate the effectiveness of the new model.
View details
Preview abstract
One of the most basic problems for studying the "price of privacy over time" is the so called private counter problem, introduced by Dwork et al. (2010) and Chan et al. (2011). In this problem, we aim to track the number of events that occur over time, while hiding the existence of every single event. More specifically, in every time step $t\in[T]$ we learn (in an online fashion) that $\Delta_t\geq 0$ new events have occurred, and must respond with an estimate $n_t\approx\sum_{j=1}^t \Delta_j$. The privacy requirement is that all of the outputs together, across all time steps, satisfy event level differential privacy.
The main question here is how our error needs to depend on the total number of time steps $T$ and the total number of events $n$. Dwork et al. (2015) showed an upper bound of $O\left(\log(T)+\log^2(n)\right)$, and Henzinger et al. (2023) showed a lower bound of $\Omega\left(\min\{\log n, \log T\}\right)$. We show a new lower bound of $\Omega\left(\min\{n,\log T\}\right)$, which is tight w.r.t. the dependence on $T$, and is tight in the sparse case where $\log^2 n=O(\log T)$. Our lower bound has the following implications:
* We show that our lower bound extends to the online thresholds problem, where the goal is to privately answer many "quantile queries" when these queries are presented one-by-one. This resolves an open question of Bun et al. (2017).
* Our lower bound implies, for the first time, a separation between the number of mistakes obtainable by a private online learner and a non-private online learner. This partially resolves a COLT'22 open question published by Sanyal and Ramponi.
* Our lower bound also yields the first separation between the standard model of private online learning and a recently proposed relaxed variant of it, called private online prediction.
View details
Rambler: Supporting Writing With Speech via LLM-Assisted Gist Manipulation
Susan Lin
Jeremy Warner
J.D. Zamfirescu-Pereira
Matthew G Lee
Sauhard Jain
Michael Xuelin Huang
Bjoern Hartmann
Can Liu
Proceedings of the 2024 CHI Conference on Human Factors in Computing Systems, Association for Computing Machinery, New York, NY, USA
Preview abstract
Dictation enables efficient text input on mobile devices. However, writing with speech can produce disfluent, wordy, and incoherent text and thus requires heavy post-processing. This paper presents Rambler, an LLM-powered graphical user interface that supports gist-level manipulation of dictated text with two main sets of functions: gist extraction and macro revision. Gist extraction generates keywords and summaries as anchors to support the review and interaction with spoken text. LLM-assisted macro revisions allow users to respeak, split, merge, and transform dictated text without specifying precise editing locations. Together they pave the way for interactive dictation and revision that help close gaps between spontaneously spoken words and well-structured writing. In a comparative study with 12 participants performing verbal composition tasks, Rambler outperformed the baseline of a speech-to-text editor + ChatGPT, as it better facilitates iterative revisions with enhanced user control over the content while supporting surprisingly diverse user strategies.
View details
Preview abstract
This paper presents NOMAD (Non-Matching Audio Distance), a differentiable perceptual similarity metric that measures the distance of a degraded signal against non-matching references. The proposed method is based on learning deep feature embeddings via a triplet loss guided by the Neurogram Similarity Index Measure (NSIM) to capture degradation intensity. During inference, the similarity score between any two audio samples is computed through Euclidean distance of their embeddings. NOMAD is fully unsupervised and can be used in general perceptual audio tasks for audio analysis e.g. quality assessment and generative tasks such as speech enhancement and speech synthesis. The proposed method is evaluated with 3 tasks. Ranking degradation intensity, predicting speech quality, and as a loss function for speech enhancement. Results indicate NOMAD outperforms other non-matching reference approaches in both ranking degradation intensity and quality assessment, exhibiting competitive performance with full-reference audio metrics. NOMAD demonstrates a promising technique that mimics human capabilities in assessing audio quality with non-matching references to learn perceptual embeddings without the need for human-generated labels.
View details
Preview abstract
A vast amount of human discussion, storytelling, content creation,
and reporting now occurs on social media platforms. As such, social
media posts are often quoted on web pages as context. In this
paper, we argue that these quotations and their surrounding page
context provide a rich, platform-independent source of data for
studying the intersection of natural language and social media.
We introduce a taxonomy of quotation roles that categorizes how
social media posts are used within content. We release a dataset
of 38M social quotes derived from the Common Crawl, and role
labels for a subset assessed by human raters. We show that the
interplay of accounts, roles, and topics across the web graph reveal
valuable social diffusion patterns, and that roles can be predicted
with fine-tuned large language models from web context.
View details
Exploring the Feasibility of Remote Cardiac Auscultation Using Earphones
Tao Chen
Yongjie Yang
Xiuzhen Guo
Jie Xiong
Shangguan Longfei
MobiCom 2024: The 30th Annual International Conference On Mobile Computing And Networking
Preview abstract
The elderly over 65 accounts for 80% of COVID deaths in the United States. In response to the pandemic, the federal, state governments, and commercial insurers are promoting video visits, through which the elderly can access specialists at home over the Internet, without the risk of COVID exposure. However, the current video visit practice barely relies on video observation and talking. The specialist could not assess the patient's health conditions by performing auscultations.
This paper tries to address this key missing component in video visits by proposing Asclepius, a hardware-software solution that turns the patient's earphones into a stethoscope, allowing the specialist to hear the patient's fine-grained heart sound (i.e., PCG signals) in video visits. To achieve this goal, we contribute a low-cost plug-in peripheral that repurposes the earphone's speaker into a microphone and uses it to capture the patient's minute PCG signals from her ear canal. As the PCG signals suffer from strong attenuation and multi-path effects when propagating from the heart to ear canals, we then propose efficient signal processing algorithms coupled with a data-driven approach to de-reverberate and further correct the amplitude and frequency distortion in raw PCG receptions. We implement Asclepius on a 2-layer PCB board and follow the IRB protocol to evaluate its performance with 30 volunteers. Our extensive experiments show that Asclepius can effectively recover Phonocardiogram (PCG) signals with different types of earphones. The feedback from cardiologists also confirms the efficacy and efficiency of our system. PCG signal samples and benchmark results can be found at an anonymous link https://asclepius-system.github.io/
View details
TRINDs: Assessing the Diagnostic Capabilities of Large Language Models for Tropical and Infectious Diseases
Steve Adudans
Oluwatosin Akande
Chintan Ghate
Sylvanus Aitkins
Geoffrey Siwo
Lynda Osadebe
Nenad Tomašev
Eric Ndombi
Preview abstract
Neglected tropical diseases (NTDs) and infectious diseases disproportionately affect the poorest regions of the world. While large language models (LLMs) have shown promise for medical question answering, there is limited work focused on tropical and infectious disease-specific explorations. We introduce TRINDs, a dataset of 52 tropical and infectious diseases with demographic and semantic clinical and consumer augmentations. We evaluate various context and counterfactual locations to understand their influence on LLM performance. Results show that LLMs perform best when provided with contextual information such as demographics, location, and symptoms. We also develop TRINDs-LM, a tool that enables users to enter symptoms and contextual information to receive a most likely diagnosis. In addition to the LLM evaluations, we also conducted a human expert baseline study to assess the accuracy of human experts in diagnosing tropical and infectious diseases with 7 medical and public health experts. This work demonstrates methods for creating and evaluating datasets for testing and optimizing LLMs, and the use of a tool that could improve digital diagnosis and tracking of NTDs.
View details
Community search signatures as foundation features for human-centered geospatial modeling
Chaitanya Kamath
Mohit Agarwal
David Schottlander
Shailesh Bavadekar
Niv Efron
Shravya Shetty
ICML 2024 Workshop on Data-Centric Machine Learning Research
Preview abstract
Aggregated relative search frequencies offer a unique composite signal reflecting people's habits, concerns, interests, intents, and general information needs, which are not found in other readily available datasets. Temporal search trends have been successfully used to perform nowcasting across a variety of domains such as infectious diseases, unemployment rates, and retail sales. However, most existing applications require curating specialized datasets of individual keywords, queries, or query clusters, and the search data need to be temporally aligned with the outcome variable of interest. We propose a novel approach for generating an aggregated and anonymized representation of search interest as foundation features at the community level for geospatial modeling. We benchmark these features using spatial datasets across multiple domains. In regions with a population greater than 3000 that cover over 95% of the contiguous US population, our models achieve an average R-squared score of 0.74 across 21 health variables, and 0.80 across 6 demographic and environmental variables. Our results demonstrate that these search features can be used for spatial predictions without strict temporal alignment, and that the resulting models outperform spatial interpolation and state of the art methods using satellite imagery features.
View details
Now You See Me, Now You Don't: 'Poverty of the Stimulus' Problems and Arbitrary Correspondences in End-to-End Speech Models
Proceedings of the Second Workshop on Computation and Written Language (CAWL) 2024
Preview abstract
End-to-end models for speech recognition and speech synthesis have many benefits, but we argue they also face a unique set of challenges not encountered in conventional multi-stage hybrid systems, which relied on the explicit injection of linguistic knowledge through resources such as phonemic dictionaries and verbalization grammars. These challenges include handling words with unusual grapheme-to-phoneme correspondences, converting between written forms like ‘12’ and spoken forms such as ‘twelve’, and contextual disambiguation of homophones or homographs. We describe the mitigation strategies that have been used for these problems in end-to-end systems, either implicitly or explicitly, and call out that the most commonly used mitigation techniques are likely incompatible with newly emerging approaches that use minimal amounts of supervised audio training data. We review best-of-both-world approaches that allow the use of end-to-end models combined with traditional linguistic resources, which we show are increasingly straightforward to create at scale, and close with an optimistic outlook for bringing speech technologies to many more languages by combining these strands of research.
View details
Preview abstract
For Extended Reality (XR) headsets, a key aim is the natural interaction in 3D space beyond what traditional methods of keyboard, mouse, and touchscreen can offer. With the release of the Apple Vision Pro, a novel interaction paradigm is now widely available where users seamlessly navigate content through the combined use of their eyes and hands. However, blending these modalities poses unique design challenges due to their dynamic nature and the absence of established principles and standards.
In this article, we present five design principles and issues for the Gaze + Pinch interaction technique, informed by eye-hand research in the human-computer interaction field. The design principles encompass mechanisms like division of labor and minimalistic timing, which are crucial for usability, alongside enhancements for the manipulation of objects, indirect interactions, and drag & drop. Whether in design, technology, or research domains, this exploration offers valuable perspectives for navigating the evolving landscape of 3D interaction.
View details
Preview abstract
We describe a quantum algorithm for the Planted Noisy kXOR problem (also known as sparse Learning Parity with Noise) that achieves a nearly quartic (4th power) speedup over the best known classical algorithm while also only using logarithmically many qubits. Our work generalizes and simplifies prior work of Hastings, by building on his quantum algorithm for the Tensor Principal Component Analysis (PCA) problem. We achieve our quantum speedup using a general framework based on the Kikuchi Method (recovering the quartic speedup for Tensor PCA), and we anticipate it will yield similar speedups for further planted inference problems. These speedups rely on the fact that planted inference problems naturally instantiate the Guided Sparse Hamiltonian problem. Since the Planted Noisy kXOR problem has been used as a component of certain cryptographic constructions, our work suggests that some of these are susceptible to super-quadratic quantum attacks.
View details
Meta Lifelong-Learning With Selective and Task-Aware Adaptation
Thanapapas Horsuwan
Kasidis Kanwatchara
Boonserm Kijsirikul
Peerapon Vateekul
IEEE Access, 12 (2024), pp. 34099-34115
Preview abstract
Meta-learning has been applied to lifelong language learning due to its ability to find an optimal model for efficient adaptation to any learned tasks. Generally, meta lifelong-learning partially stores samples from seen tasks in a memory and selects some of them to train the model, refresh the knowledge, and adapt the model for inference. However, the sample selection for these steps was usually done in a sub-optimal manner in existing work. Hence, we propose MeLSTA (Meta Lifelong-Learning with Selective and Task-Aware Adaptation) to effectively select the samples based on task identifiers and the adaptation scores reflecting the model behavior after adaptation. The results show that MeLSTA enhances the accuracy by 1.2% over the state-of-the-art while significantly shrinking the training duration by over 6 times. Additionally, our in-depth analysis reveals the strengths and limitations of MeLSTA and existing work, providing useful insights for future designs of meta lifelong-learning for NLP.
View details
Bridging the Gap: Unpacking the Hidden Challenges in Knowledge Distillation for Online Ranking Systems
Shuo Yang
Aniruddh Nath
Yang Liu
Li Wei
Shawn Andrews
Maciej Kula
Jarrod Kahn
Zhe Zhao
Lichan Hong
Preview abstract
Knowledge Distillation (KD) is a powerful approach for compressing large models into smaller, more efficient models, particularly beneficial for latency-sensitive applications like recommender systems. However, current KD research predominantly focuses on Computer Vision (CV) and NLP tasks, overlooking unique data characteristics and challenges inherent to recommender systems. This paper addresses these overlooked challenges, specifically: (1) mitigating data distribution shifts between teacher and student models, (2) efficiently identifying optimal teacher configurations within time and budgetary constraints, and (3) enabling computationally efficient and rapid sharing of teacher labels to support multiple students. We present a robust KD system developed and rigorously evaluated on multiple large-scale personalized video recommendation systems within Google. Our live experiment results demonstrate significant improvements in student model performance while ensuring the consistent and reliable generation of high-quality teacher labels from continuous data streams.
View details