Publications
Our teams aspire to make discoveries that impact everyone, and core to our approach is sharing our research and tools to fuel progress in the field.
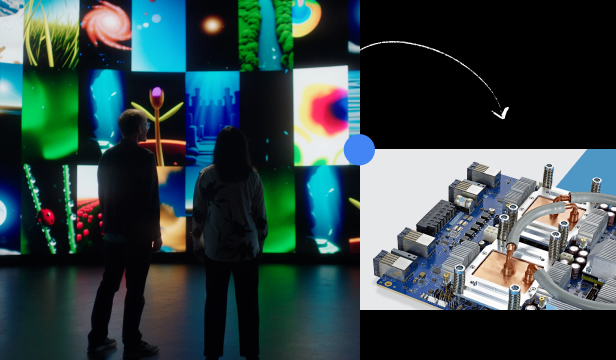
Our teams aspire to make discoveries that impact everyone, and core to our approach is sharing our research and tools to fuel progress in the field.
Sort By
1 - 15 of 10129 publications
Rich Human Feedback for Text to Image Generation
Katherine Collins
Nicholas Carolan
Youwei Liang
Peizhao Li
Dj Dvijotham
Gang Li
Sarah Young
Jiao Sun
Arseniy Klimovskiy
Preview abstract
Recent Text-to-Image (T2I) generation models such as Stable Diffusion and Imagen have made significant progress in generating high-resolution images based on text descriptions. However, many generated images still suffer from issues such as artifacts/implausibility, misalignment with text descriptions, and low aesthetic quality.
Inspired by the success of Reinforcement Learning with Human Feedback (RLHF) for large language models, prior work collected human-provided scores as feedback on generated images and trained a reward model to improve the T2I generation.
In this paper, we enrich the feedback signal by (i) marking image regions that are implausible or misaligned with the text, and (ii) annotating which keywords in the text prompt are not represented in the image.
We collect such rich human feedback on 18K generated images and train a multimodal transformer to predict these rich feedback automatically.
We show that the predicted rich human feedback can be leveraged to improve image generation, for example, by selecting high-quality training data to finetune and improve the generative models, or by creating masks with predicted heatmaps to inpaint the problematic regions.
Notably, the improvements generalize to models (Muse) beyond those used to generate the images on which human feedback data were collected (Stable Diffusion variants).
View details
Recent Books and Journal Articles in Public Opinion, Survey Methods, Survey Statistics, Big Data, Data Science, and User Experience Research. 2023 Update
Mario Callegaro
Survey Practice, 17 (2024)
Preview abstract
Welcome to the 16th edition of this column on recent books and journal articles in the field of public opinion, survey methods, survey statistics, Big Data, data science, and user experience research.
Special issues of journals have a space in this article because, in our view, they are like edited books. We also added review papers from the journal series of Annual Reviews because these papers are seminal state of the art write ups, a mini book, if you wish on a specific subject.
This article is an update of the books and journals published in the 2022 article. Like the previous year, the books are organized by topic; this should help the readers to focus on their interests.
You will note that we use very broad definitions of public opinion, survey methods, survey statistics, Big Data, data science, and user experience research. This is because there are many books published in different outlets that can be very useful to the readers of Survey Practice, even if they do not come from traditional sources of survey content.
It is unlikely we have exhaustively listed all new books in each subcategory; we did our best scouting different resources and websites, but we take full responsibility for any omissions. The list is also focused only on books published in the English language and available for purchase (as an ebook or in print) at the time of this review (April 2024) and with the printed copyright year of 2023. Books are listed based on the relevance to the topic, and no judgment is made in terms of quality of the content. We let the readers do so.
If you want to send information for the next issue, please send it to surveypractice.new.books@gmail.com.
View details
Experiencing Thing2Reality: Transforming 2D Content into Conditioned Multiviews and 3D Gaussian Objects for XR Communication
Erzhen Hu
Mingyi Li
Seongkook Heo
Adjunct Proceedings of the 33rd Annual ACM Symposium on User Interface Software and Technology, ACM (2024)
Preview abstract
During remote communication, participants share both digital and physical content, such as product designs, digital assets, and environments, to enhance mutual understanding. Recent advances in augmented communication have facilitated users to swiftly create and share digital 2D copies of physical objects from video feeds into a shared space. However, the conventional 2D representation of digital objects restricts users’ ability to spatially reference items in a shared immersive environment. To address these challenges, we propose Thing2Reality, an Extended Reality (XR) communication platform designed to enhance spontaneous discussions regard-ing both digital and physical items during remote sessions. WithThing2Reality, users can quickly materialize ideas or physical objects in immersive environments and share them as conditioned multiview renderings or 3D Gaussians. Our system enables users to interact with remote objects or discuss concepts in a collaborative manner.
View details
PaLI-X: On Scaling up a Multilingual Vision and Language Model
Josip Djolonga
Piotr Padlewski
Basil Mustafa
Carlos Riquelme
Sebastian Goodman
Yi Tay
Siamak Shakeri
Daniel Salz
Michael Tschannen
Mandar Joshi
Filip Pavetić
Gang Li
Anurag Arnab
Yuanzhong Xu
Keran Rong
Neil Houlsby
Computer Vision and Pattern Recognition Conference (CVPR) (2024)
Preview abstract
We explore the boundaries of scaling up a multilingual vision and language model, both in terms of size of the components and the breadth of its training task mixture. Our model achieves new levels of performance on a wide-range of varied and complex tasks, including multiple image-based captioning and question-answering tasks, image-based document understanding and few-shot (in-context) learning, as well as object detection, video question answering, and video captioning. Our model advances the state-of-the-art on most vision-and-language benchmarks considered (20+ of them). Finally, we observe emerging capabilities, such as complex counting and multilingual object detection, tasks that are not explicitly in the training mix.
View details
Preview abstract
Given a training data-set $\mathcal{S}$, and a reference data-set $\mathcal{T}$, we design a simple and efficient algorithm to reweigh the loss function such that the limiting distribution of the neural network weights that result from training on $\mathcal{S}$ approaches the limiting distribution that would have resulted by training on $\mathcal{T}$. Such reweighing can be used to correct for Train-Test distribution shift, when we don't have access to the labels of $\mathcal{T}$. It can also be used to perform (soft) multi-criteria optimization on neural nets, when we have access to the labels of $\mathcal{T}$, but $\mathcal{S}$ and $\mathcal{T}$ have few common points.
As a motivating application, we train a graph neural net to recognize small molecule binders to MNK2 (a MAP Kinase, responsible for cell signaling) which are non-binders to MNK1 (a very similar protein), even in the absence of training data common to both data-sets. We are able to tune the reweighing parameters so that overall change in holdout loss is negligible, but the selectivity, i.e., the fraction of top 100 MNK2 binders that are MNK1 non-binders, increases from 54\% to 95\%, as a result of our reweighing.
We expect the algorithm to be applicable in other settings as well, since we prove that when the metric entropy of the input data-sets is bounded, our random sampling based greedy algorithm outputs a close to optimal reweighing, i.e., the two invariant distributions of network weights will be provably close in total variation distance.
View details
Preview abstract
In this paper we study users' opinions about the privacy of their mobile health apps. We look at what they write in app reviews in the 'Health & Fitness' category on the Google Play store. We identified 2832 apps in this category (based on 1K minimum installs). Using NLP/LLM analyses, we find that 76% of these apps have at least some privacy reviews. In total this yields over 164,000 reviews about privacy, from over 150 countries and in 25 languages. Our analyses identifies top themes and offers an approximation of how widespread these issues are around the world. We show that the top 4 themes - Data Sharing and Exposure, Permission Requests, Location Tracking and Data Collection - are issues of concern in over 70 countries. Our automatically generated thematic summaries reveal interesting aspects that deserve further research around user suspicions (unneeded data collection), user requests (more fine-grained control over data collection and data access), as well as user behavior (uninstalling apps).
View details
Preview abstract
Machine learning has a pseudoscience problem. An abundance of ethical issues arising from the use of machine learning (ML)-based technologies—by now, well documented—is inextricably entwined with the systematic epistemic misuse of these tools. We take a recent resurgence of deep learning-assisted physiognomic research as a case study in the relationship between ML-based pseudoscience and attendant social harms—the standard purview of “AI ethics.” In practice, the epistemic and ethical dimensions of ML misuse often arise from shared underlying reasons and are resolvable by the same pathways. Recent use of ML toward the ends of predicting protected attributes from photographs highlights the need for philosophical, historical, and domain-specific perspectives of particular sciences in the prevention and remediation of misused ML.
View details
Learning to Rewrite Prompts for Personalized Text Generation
Qiaozhu Mei
Proceedings of the ACM Web Conference 2024
Preview abstract
Facilitated by large language models (LLMs), personalized text generation has become a rapidly growing research direction. Most existing studies focus on designing specialized models for a particular domain, or they require fine-tuning the LLMs to generate personalized text. We consider a typical scenario in which the large language model, which generates personalized output, is frozen and can only be accessed through APIs. Under this constraint, all one can do is to improve the input text (i.e., text prompts) sent to the LLM, a procedure that is usually done manually. In this paper, we propose a novel method to automatically revise prompts for personalized text generation. The proposed method takes the initial prompts generated by a state-of-the-art, multistage framework for personalized generation and rewrites a few critical components that summarize and synthesize the personal context. The prompt rewriter employs a training paradigm that chains together supervised learning (SL) and reinforcement learning (RL), where SL reduces the search space of RL and RL facilitates end-to-end training of the rewriter. Using datasets from three representative domains, we demonstrate that the rewritten prompts outperform both the original prompts and the prompts optimized via supervised learning or reinforcement learning alone. In-depth analysis of the rewritten prompts shows that they are not only human readable, but also able to guide manual revision of prompts when there is limited resource to employ reinforcement learning to train the prompt rewriter, or when it is costly to deploy an automatic prompt rewriter for inference.
View details
PRewrite: Prompt Rewriting with Reinforcement Learning
Qiaozhu Mei
Proceedings of the 62nd Annual Meeting of the Association for Computational Linguistics (2024) (to appear)
Preview abstract
Prompt engineering is critical for the development of LLM-based applications. However, it is usually done manually in a "trial and error" fashion that can be time consuming, ineffective, and sub-optimal. Even for the prompts which seemingly work well, there is always a lingering question: can the prompts be made better with further modifications?
To address these problems, we investigate automated prompt engineering in this paper. Specifically, we propose PRewrite, an automated method to rewrite an under-optimized prompt to a more effective prompt. We instantiate the prompt rewriter using an LLM. The rewriter LLM is trained using reinforcement learning to optimize the performance on a given downstream task. We conduct experiments on diverse benchmark datasets, which demonstrates the effectiveness of PRewrite.
View details
PRISM: A New Lens for Improved Color Understanding
Garima Pruthi
Inderjit Dhillon
Varun Jampani
EMNLP (2024)
Preview abstract
While image-text pre-trained models, such as CLIP, have demonstrated impressive capabilities in learning robust text and image representations, a critical area for substantial improvement remains—precise color understanding. In this paper, we address this limitation by introducing PRISM, a simple yet highly effective method that extends CLIP's capability to grasp the nuances of precise colors. PRISM seamlessly adapts to both recognized HTML colors and out-of-vocabulary RGB inputs through the utilization of our curated dataset of 100 image-text pairs, which can be effortlessly repurposed for fine-tuning with any desired color. Importantly, PRISM achieves these enhancements without compromising CLIP's performance on established benchmarks. During the fine-tuning process, PRISM encourages the disentanglement of color-relevant information from color-irrelevant details. Furthermore, we introduce a novel evaluation framework, ColorLens, featuring both seen and unseen test sets that can be readily repurposed to assess a model's precision in understanding precise colors. Our comprehensive evaluation and results demonstrate significant improvements over baseline models.
View details
LFM-3D: Learnable Feature Matching Across Wide Baselines Using 3D Signals
Arjun Karpur
Guilherme Perrotta
Ricardo Martin-Brualla
Proc. 3DV'24 (2024) (to appear)
Preview abstract
Finding localized correspondences across different images of the same object is crucial to understand its geometry. In recent years, this problem has seen remarkable progress with the advent of deep learning-based local image features and learnable matchers. Still, learnable matchers often underperform when there exists only small regions of co-visibility between image pairs (i.e. wide camera baselines). To address this problem, we leverage recent progress in coarse single-view geometry estimation methods. We propose LFM-3D, a Learnable Feature Matching framework that uses models based on graph neural networks and enhances their capabilities by integrating noisy, estimated 3D signals to boost correspondence estimation. When integrating 3D signals into the matcher model, we show that a suitable positional encoding is critical to effectively make use of the low-dimensional 3D information. We experiment with two different 3D signals - normalized object coordinates and monocular depth estimates - and evaluate our method on large-scale (synthetic and real) datasets containing object-centric image pairs across wide baselines. We observe strong feature matching improvements compared to 2D-only methods, with up to +6% total recall and +28% precision at fixed recall. Additionally, we demonstrate that the resulting improved correspondences lead to much higher relative posing accuracy for in-the-wild image pairs - up to 8.6% compared to the 2D-only approach.
View details
Preview abstract
Private Everlasting Prediction (PEP), recently introduced by Naor et al. [2023], is a model for differentially private learning in which the learner never publicly releases a hypothesis. Instead, it provides a black-box access to a ``prediction oracle'' that can predict the labels of an endless stream of unlabeled examples drawn from the underlying distribution. Importantly, PEP provides privacy both for the initial training set and for the endless stream of classification queries. We present two conceptual modifications to the definition of PEP, as well as new constructions exhibiting significant improvements over prior work. Specifically, our contributions include:
(1) Robustness: PEP only guarantees accuracy provided that all the classification queries are drawn from the correct underlying distribution. A few out-of-distribution queries might break the validity of the prediction oracle for future queries, even for future queries which are sampled from the correct distribution. We incorporate robustness against such poisoning attacks into the definition of PEP, and show how to obtain it.
(2) Dependence of the privacy parameter delta in the time horizon: We present a relaxed privacy definition, suitable for PEP, that allows us to disconnect the privacy parameter delta from the number of total time steps T. This allows us to obtain algorithms for PEP whose sample complexity is independent from T, thereby making them "truly everlasting". This is in contrast to prior work where the sample complexity grows with polylog(T).
(3) New constructions: Prior constructions for PEP exhibit sample complexity that is quadratic in the VC dimension of the target class. We present new constructions of PEP for axis-aligned rectangles and for decision-stumps, that exhibit sample complexity linear in the dimension (instead of quadratic). We show that our constructions satisfy very strong robustness properties.
View details
Preview abstract
The effect of regularizers such as weight decay when training deep neural networks is not well understood. We study the influence of weight decay as well as $L2$-regularization when training neural network models in which parameter matrices interact multiplicatively. This combination is of particular interest as this parametrization is common in attention layers, the workhorse of transformers. Here, key-query, as well as value-projection parameter matrices, are multiplied directly with each other: $W_K^TW_Q$ and $PW_V$.
We extend previous results and show on one hand that any local minimum of a $L2$-regularized loss of the form $L(AB^\top) + \lambda (\|A\|^2 + \|B\|^2)$ coincides with a minimum of the nuclear norm-regularized loss $L(AB^\top) + \lambda\|AB^\top\|_*$, and on the other hand that the 2 losses become identical exponentially quickly during training. We thus complement existing works linking $L2$-regularization with low-rank regularization, and in particular, explain why such regularization on the matrix product affects early stages of training.
Based on these theoretical insights, we verify empirically that the key-query and value-projection matrix products $W_K^TW_Q, PW_V$ within attention layers, when optimized with weight decay, as usually done in vision tasks and language modelling, indeed induce a significant reduction in the rank of $W_K^TW_Q$ and $PW_V$, even in fully online training.
We find that, in accordance with existing work, inducing low rank in attention matrix products can damage language model performance, and observe advantages when decoupling weight decay in attention layers from the rest of the parameters.
View details
DiffHuman: Probabilistic Photorealistic 3D Reconstruction of Humans
Akash Sengupta
Enric Corona
Andrei Zanfir
Proceedings of the IEEE/CVF Conference on Computer Vision and Pattern Recognition (CVPR) (2024)
Preview abstract
We present DiffHuman, a probabilistic method for photorealistic 3D human reconstruction from a single RGB image. Despite the ill-posed nature of this problem, most methods are deterministic and output a single solution, often resulting in a lack of geometric detail and blurriness in unseen or uncertain regions. In contrast, DiffHuman predicts a distribution over 3D reconstructions conditioned on an image, which allows us to sample multiple detailed 3D avatars that are consistent with the input image. DiffHuman is implemented as a conditional diffusion model that denoises partial observations of an underlying pixel-aligned 3D representation. In testing, we can sample a 3D shape by iteratively denoising renderings of the predicted intermediate representation. Further, we introduce an additional generator neural network that approximates rendering with considerably reduced runtime (55x speed up), resulting in a novel dual-branch diffusion framework. We evaluate the effectiveness of our approach through various experiments. Our method can produce diverse, more detailed reconstructions for the parts of the person not observed in the image, and has competitive performance for the surface reconstruction of visible parts.
View details
Preview abstract
Motivated by recent advances in large language models for NLP, we design a time-series foundation model for forecasting whose out-of-the-box zero-shot performance on a variety of datasets, matches the accuracy of state-of-the-art supervised forecasting models for each individual dataset. Our model is based on pretraining a patched-decoder style attention model on a large time series dataset, and can work well across different forecasting history lengths, prediction lengths and temporal granularities.
View details