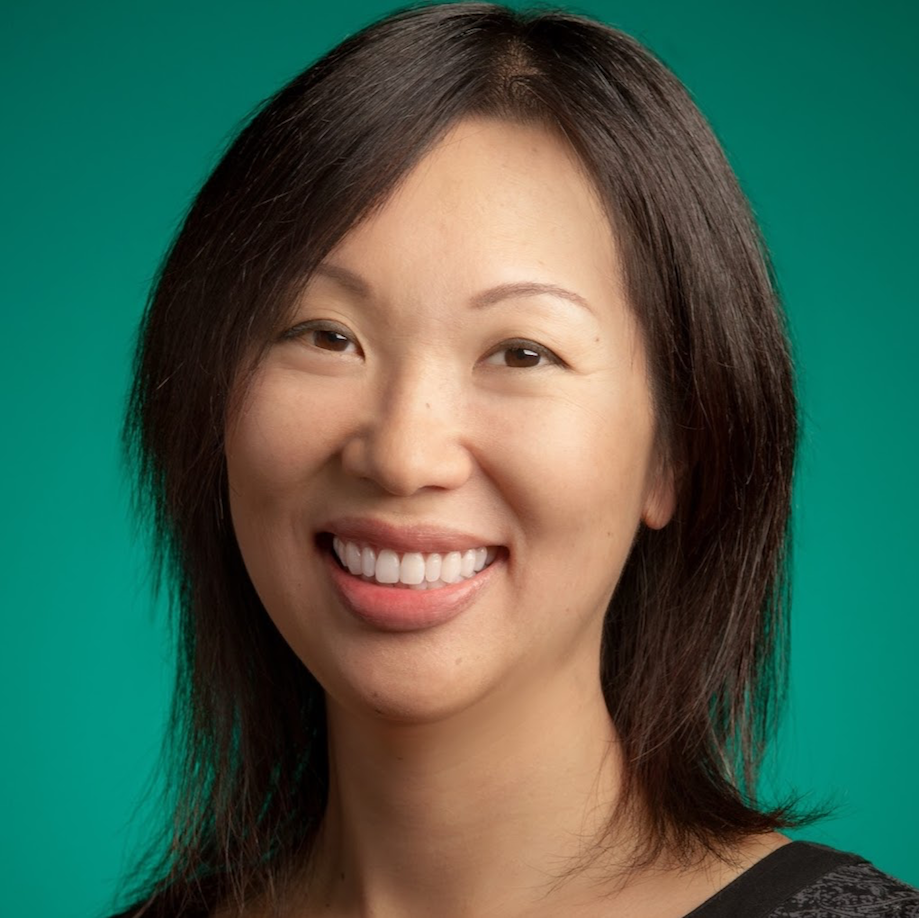
Kasumi Widner
Research Areas
Authored Publications
Sort By
Lessons learned from translating AI from development to deployment in healthcare
Sunny Virmani
Jay Nayar
Elin Rønby Pedersen
Divleen Jeji
Lily Peng
Nature Medicine (2023)
Preview abstract
The application of an artificial intelligence (AI)-based screening tool for retinal disease in India and Thailand highlighted the myths and reality of introducing medical AI, which may form a framework for subsequent tools.
View details
Real-time diabetic retinopathy screening by deep learning in a multisite national screening programme: a prospective interventional cohort study
Dr. Paisan Raumviboonsuk
Variya Nganthavee
Kornwipa Hemarat
Apinpat Kongprayoon
Rajiv Raman
Brian Levinstein
Roy Lee
Sunny Virmani
John Chambers
Fred Hersch
Lily Hao Yi Peng
The Lancet Digital Health (2022)
Preview abstract
Background:
Diabetic retinopathy is a leading cause of preventable blindness, especially in low-income and middle-income countries (LMICs). Deep-learning systems have the potential to enhance diabetic retinopathy screenings in these settings, yet prospective studies assessing their usability and performance are scarce.
Methods:
We did a prospective interventional cohort study to evaluate the real-world performance and feasibility of deploying a deep-learning system into the health-care system of Thailand. Patients with diabetes and listed on the national diabetes registry, aged 18 years or older, able to have their fundus photograph taken for at least one eye, and due for screening as per the Thai Ministry of Public Health guidelines were eligible for inclusion. Eligible patients were screened with the deep-learning system at nine primary care sites under Thailand's national diabetic retinopathy screening programme. Patients with a previous diagnosis of diabetic macular oedema, severe non-proliferative diabetic retinopathy, or proliferative diabetic retinopathy; previous laser treatment of the retina or retinal surgery; other non-diabetic retinopathy eye disease requiring referral to an ophthalmologist; or inability to have fundus photograph taken of both eyes for any reason were excluded. Deep-learning system-based interpretations of patient fundus images and referral recommendations were provided in real time. As a safety mechanism, regional retina specialists over-read each image. Performance of the deep-learning system (accuracy, sensitivity, specificity, positive predictive value [PPV], and negative predictive value [NPV]) were measured against an adjudicated reference standard, provided by fellowship-trained retina specialists. This study is registered with the Thai national clinical trials registry, TCRT20190902002.
Findings:
Between Dec 12, 2018, and March 29, 2020, 7940 patients were screened for inclusion. 7651 (96·3%) patients were eligible for study analysis, and 2412 (31·5%) patients were referred for diabetic retinopathy, diabetic macular oedema, ungradable images, or low visual acuity. For vision-threatening diabetic retinopathy, the deep-learning system had an accuracy of 94·7% (95% CI 93·0–96·2), sensitivity of 91·4% (87·1–95·0), and specificity of 95·4% (94·1–96·7). The retina specialist over-readers had an accuracy of 93·5 (91·7–95·0; p=0·17), a sensitivity of 84·8% (79·4–90·0; p=0·024), and specificity of 95·5% (94·1–96·7; p=0·98). The PPV for the deep-learning system was 79·2 (95% CI 73·8–84·3) compared with 75·6 (69·8–81·1) for the over-readers. The NPV for the deep-learning system was 95·5 (92·8–97·9) compared with 92·4 (89·3–95·5) for the over-readers.
Interpretation:
A deep-learning system can deliver real-time diabetic retinopathy detection capability similar to retina specialists in community-based screening settings. Socioenvironmental factors and workflows must be taken into consideration when implementing a deep-learning system within a large-scale screening programme in LMICs.
Funding:
Google and Rajavithi Hospital, Bangkok, Thailand.
View details
Performance of a Deep-Learning Algorithm vs Manual Grading for Detecting Diabetic Retinopathy in India
Renu P. Rajan
Derek Wu
Peter Wubbels
Tyler Rhodes
Kira Whitehouse
Ramasamy Kim
Rajiv Raman
Lily Peng
JAMA Ophthalmology (2019)
Preview abstract
Importance More than 60 million people in India have diabetes and are at risk for diabetic retinopathy (DR), a vision-threatening disease. Automated interpretation of retinal fundus photographs can help support and scale a robust screening program to detect DR.
Objective To prospectively validate the performance of an automated DR system across 2 sites in India.
Design, Setting, and Participants This prospective observational study was conducted at 2 eye care centers in India (Aravind Eye Hospital and Sankara Nethralaya) and included 3049 patients with diabetes. Data collection and patient enrollment took place between April 2016 and July 2016 at Aravind and May 2016 and April 2017 at Sankara Nethralaya. The model was trained and fixed in March 2016.
Interventions Automated DR grading system compared with manual grading by 1 trained grader and 1 retina specialist from each site. Adjudication by a panel of 3 retinal specialists served as the reference standard in the cases of disagreement.
Main Outcomes and Measures Sensitivity and specificity for moderate or worse DR or referable diabetic macula edema.
Results Of 3049 patients, 1091 (35.8%) were women and the mean (SD) age for patients at Aravind and Sankara Nethralaya was 56.6 (9.0) years and 56.0 (10.0) years, respectively. For moderate or worse DR, the sensitivity and specificity for manual grading by individual nonadjudicator graders ranged from 73.4% to 89.8% and from 83.5% to 98.7%, respectively. The automated DR system’s performance was equal to or exceeded manual grading, with an 88.9% sensitivity (95% CI, 85.8-91.5), 92.2% specificity (95% CI, 90.3-93.8), and an area under the curve of 0.963 on the data set from Aravind Eye Hospital and 92.1% sensitivity (95% CI, 90.1-93.8), 95.2% specificity (95% CI, 94.2-96.1), and an area under the curve of 0.980 on the data set from Sankara Nethralaya.
Conclusions and Relevance This study shows that the automated DR system generalizes to this population of Indian patients in a prospective setting and demonstrates the feasibility of using an automated DR grading system to expand screening programs.
View details
Deep learning versus human graders for classifying diabetic retinopathy severity in a nationwide screening program
Dr. Paisan Raumviboonsuk
Dr. Peranut Chotcomwongse
Rajiv Raman
Sonia Phene
Kornwipa Hemarat
Mongkol Tadarati
Sukhum Silpa-Archa
Jirawut Limwattanayingyong
Chetan Rao
Oscar Kuruvilla
Jesse Jung
Jeffrey Tan
Surapong Orprayoon
Chawawat Kangwanwongpaisan
Ramase Sukumalpaiboon
Chainarong Luengchaichawang
Jitumporn Fuangkaew
Pipat Kongsap
Lamyong Chualinpha
Sarawuth Saree
Srirut Kawinpanitan
Korntip Mitvongsa
Siriporn Lawanasakol
Chaiyasit Thepchatri
Lalita Wongpichedchai
Lily Peng
Nature Partner Journal (npj) Digital Medicine (2019)
Preview abstract
Deep learning algorithms have been used to detect diabetic retinopathy (DR) with specialist-level accuracy. This study aims to validate one such algorithm on a large-scale clinical population, and compare the algorithm performance with that of human graders. A total of 25,326 gradable retinal images of patients with diabetes from the community-based, nationwide screening program of DR in Thailand were analyzed for DR severity and referable diabetic macular edema (DME). Grades adjudicated by a panel of international retinal specialists served as the reference standard. Relative to human graders, for detecting referable DR (moderate NPDR or worse), the deep learning algorithm had significantly higher sensitivity (0.97 vs. 0.74, p < 0.001), and a slightly lower specificity (0.96 vs. 0.98, p < 0.001). Higher sensitivity of the algorithm was also observed for each of the categories of severe or worse NPDR, PDR, and DME (p < 0.001 for all comparisons). The quadratic-weighted kappa for determination of DR severity levels by the algorithm and human graders was 0.85 and 0.78 respectively (p < 0.001 for the difference). Across different severity levels of DR for determining referable disease, deep learning significantly reduced the false negative rate (by 23%) at the cost of slightly higher false positive rates (2%). Deep learning algorithms may serve as a valuable tool for DR screening.
View details
Grader variability and the importance of reference standards for evaluating machine learning models for diabetic retinopathy
Ehsan Rahimy
Peter Karth
Lily Peng
Ophthalmology (2018)
Preview abstract
Purpose
Use adjudication to quantify errors in diabetic retinopathy (DR) grading based on individual graders and majority decision, and to train an improved automated algorithm for DR grading.
Design
Retrospective analysis.
Participants
Retinal fundus images from DR screening programs.
Methods
Images were each graded by the algorithm, U.S. board-certified ophthalmologists, and retinal specialists. The adjudicated consensus of the retinal specialists served as the reference standard.
Main Outcome Measures
For agreement between different graders as well as between the graders and the algorithm, we measured the (quadratic-weighted) kappa score. To compare the performance of different forms of manual grading and the algorithm for various DR severity cutoffs (e.g., mild or worse DR, moderate or worse DR), we measured area under the curve (AUC), sensitivity, and specificity.
Results
Of the 193 discrepancies between adjudication by retinal specialists and majority decision of ophthalmologists, the most common were missing microaneurysm (MAs) (36%), artifacts (20%), and misclassified hemorrhages (16%). Relative to the reference standard, the kappa for individual retinal specialists, ophthalmologists, and algorithm ranged from 0.82 to 0.91, 0.80 to 0.84, and 0.84, respectively. For moderate or worse DR, the majority decision of ophthalmologists had a sensitivity of 0.838 and specificity of 0.981. The algorithm had a sensitivity of 0.971, specificity of 0.923, and AUC of 0.986. For mild or worse DR, the algorithm had a sensitivity of 0.970, specificity of 0.917, and AUC of 0.986. By using a small number of adjudicated consensus grades as a tuning dataset and higher-resolution images as input, the algorithm improved in AUC from 0.934 to 0.986 for moderate or worse DR.
Conclusions
Adjudication reduces the errors in DR grading. A small set of adjudicated DR grades allows substantial improvements in algorithm performance. The resulting algorithm's performance was on par with that of individual U.S. Board-Certified ophthalmologists and retinal specialists.
View details
Development and Validation of a Deep Learning Algorithm for Detection of Diabetic Retinopathy in Retinal Fundus Photographs
Lily Peng
Martin C Stumpe
Derek Wu
Arunachalam Narayanaswamy
Subhashini Venugopalan
Tom Madams
Jorge Cuadros
Ramasamy Kim
Rajiv Raman
Jessica Mega
JAMA (2016)
Preview abstract
Importance: Deep learning is a family of computational methods that allow an algorithm to program itself by learning from a large set of examples that demonstrate the desired behavior, removing the need to specify rules explicitly. Application of these methods to medical imaging requires further assessment and validation.
Objective: To apply deep learning to create an algorithm for automated detection of diabetic retinopathy and diabetic macular edema in retinal fundus photographs.
Design and Setting: A specific type of neural network optimized for image classification called a deep convolutional neural network was trained using a retrospective development data set of 128 175 retinal images, which were graded 3 to 7 times for diabetic retinopathy, diabetic macular edema, and image gradability by a panel of 54 US licensed ophthalmologists and ophthalmology senior residents between May and December 2015. The resultant algorithm was validated in January and February 2016 using 2 separate data sets, both graded by at least 7 US board-certified ophthalmologists with high intragrader consistency.
Exposure: Deep learning–trained algorithm.
Main Outcomes and Measures: The sensitivity and specificity of the algorithm for detecting referable diabetic retinopathy (RDR), defined as moderate and worse diabetic retinopathy, referable diabetic macular edema, or both, were generated based on the reference standard of the majority decision of the ophthalmologist panel. The algorithm was evaluated at 2 operating points selected from the development set, one selected for high specificity and another for high sensitivity.
Results: The EyePACS-1 data set consisted of 9963 images from 4997 patients (mean age, 54.4 years; 62.2% women; prevalence of RDR, 683/8878 fully gradable images [7.8%]); the Messidor-2 data set had 1748 images from 874 patients (mean age, 57.6 years; 42.6% women; prevalence of RDR, 254/1745 fully gradable images [14.6%]). For detecting RDR, the algorithm had an area under the receiver operating curve of 0.991 (95% CI, 0.988-0.993) for EyePACS-1 and 0.990 (95% CI, 0.986-0.995) for Messidor-2. Using the first operating cut point with high specificity, for EyePACS-1, the sensitivity was 90.3% (95% CI, 87.5%-92.7%) and the specificity was 98.1% (95% CI, 97.8%-98.5%). For Messidor-2, the sensitivity was 87.0% (95% CI, 81.1%-91.0%) and the specificity was 98.5% (95% CI, 97.7%-99.1%). Using a second operating point with high sensitivity in the development set, for EyePACS-1 the sensitivity was 97.5% and specificity was 93.4% and for Messidor-2 the sensitivity was 96.1% and specificity was 93.9%.
Conclusions and Relevance: In this evaluation of retinal fundus photographs from adults with diabetes, an algorithm based on deep machine learning had high sensitivity and specificity for detecting referable diabetic retinopathy. Further research is necessary to determine the feasibility of applying this algorithm in the clinical setting and to determine whether use of the algorithm could lead to improved care and outcomes compared with current ophthalmologic assessment.
View details