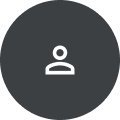
Ekin Dogus Cubuk
Authored Publications
Sort By
Preview abstract
Human-like perceptual similarity is an emergent property in the intermediate feature space of ImageNet-pretrained classifiers. Perceptual distances between images, as measured in the space of pre-trained image embeddings, have outperformed prior low-level metrics significantly on assessing image similarity. This has led to the wide adoption of perceptual distances as both an evaluation metric and an auxiliary training objective for image synthesis tasks. While image classification has improved by leaps and bounds, the de facto standard for computing perceptual distances uses older, less accurate models such as VGG and AlexNet. Motivated by this, we evaluate the perceptual scores of modern networks: ResNets, EfficientNets and VisionTransformers. Surprisingly, we observe an inverse correlation between ImageNet accuracy and perceptual scores: better classifiers achieve worse perceptual scores. We dive deeper into this, studying the ImageNet accuracy/perceptual score relationship under different hyperparameter configurations. Improving accuracy improves perceptual scores up to a certain point, but beyond this point we uncover a Pareto frontier between accuracies and perceptual scores. We explore this relationship further using distortion invariance, spatial frequency sensitivity and alternative perceptual functions. Based on our study, we find a ImageNet trained ResNet-6 network whose emergent perceptual score matches the best prior score obtained with networks trained explicitly on a perceptual similarity task.
View details
Revisiting ResNets: Improved Training Methodologies and Scaling Principles
Irwan Bello
Liam B. Fedus
Xianzhi Du
Aravind Srinivas
Tsung-Yi Lin
Jon Shlens
Barret Richard Zoph
ICML 2021(2021) (to appear)
Preview abstract
Novel ImageNet architectures monopolize the limelight when advancing the state-of-the-art, but progress is often muddled by simultaneous changes to training methodology and scaling strategies.
Our work disentangles these factors by revisiting the ResNet architecture using modern training and scaling techniques and, in doing so, we show ResNets match recent state-of-the-art models.
A ResNet trained to 79.0 top-1 ImageNet accuracy is increased to 82.2 through improved training methodology alone; two small popular architecture changes further improve this to 83.4.
We next offer new perspectives on the scaling strategy which we summarize by two key principles: (1) increase model depth and image size, but not model width (2) increase image size far more slowly than previously recommended.
Using improved training methodology and our scaling principles, we design a family of ResNet architectures, ResNet-RS, which are 1.9x - 2.3x faster than the EfficientNets in supervised learning on ImageNet.
And though EfficientNet has significantly fewer FLOPs and parameters -- training ResNet-RS is both faster and less memory-intensive, serving as a strong baseline for researchers and practitioners.
View details
Preview abstract
Though data augmentation has become a standard component of deep neural network training, the underlying mechanism behind the effectiveness of these techniques remains poorly understood. In practice, augmentation policies are often chosen using heuristics of distribution shift or augmentation diversity. Inspired by these, we conduct an empirical study to quantify how data augmentation improves model generalization. We introduce two interpretable and easy-to-compute measures: Affinity and Diversity. We find that augmentation performance is predicted not by either of these alone but by jointly optimizing the two.
View details
Kohn-Sham equations as regularizer: building prior knowledge into machine-learned physics
Li Li
Ryan Pederson
Patrick Francis Riley
Kieron Burke
Phys. Rev. Lett., 126(2021), pp. 036401
Preview abstract
Including prior knowledge is important for effective machine learning models in physics and is usually achieved by explicitly adding loss terms or constraints on model architectures. Prior knowledge embedded in the physics computation itself rarely draws attention. We show that solving the Kohn-Sham equations when training neural networks for the exchange-correlation functional provides an implicit regularization that greatly improves generalization. Two separations suffice for learning the entire one-dimensional H$_2$ dissociation curve within chemical accuracy, including the strongly correlated region. Our models also generalize to unseen types of molecules and overcome self-interaction error.
View details
ReMixMatch: Semi-Supervised Learning with Distribution Matching and Augmentation Anchoring
Colin Raffel
David Berthelot
Han Zhang
Kihyuk Sohn
ICLR, ICLR(2020)
Preview abstract
We improve the recently-proposed ``MixMatch'' semi-supervised learning algorithm by introducing two new techniques: distribution alignment and augmentation anchoring. Distribution alignment encourages the marginal distribution of predictions on unlabeled data to be close to the marginal distribution of groundtruth labels. Augmentation anchoring feeds multiple strongly augmented versions of an input into the model and encourages each output to be close to the prediction for a weakly-augmented version of the same input. To produce strong augmentations, we propose a variant of AutoAugment which learns the augmentation policy while the model is being trained. Our new algorithm, dubbed ReMixMatch, is significantly more data-efficient than prior work, requiring between 5x and 16x less data to reach the same accuracy. For example, on CIFAR10 with 250 labeled examples we reach 93.73% accuracy (compared to MixMatch’s accuracy of 93.58% with 4,000 examples) and a median accuracy of 84.92% with just four labels per class. We make our code and data open-source at https://github.com/google-research/remixmatch.
View details
Improving 3D Object Detection through Progressive Population Based Augmentation
Shuyang Cheng
Zhaoqi Leng
Barret Richard Zoph
Chunyan Bai
Jiquan Ngiam
Vijay Vasudevan
Jon Shlens
Drago Anguelov
ECCV'2020
Preview abstract
Data augmentation has been widely adopted for object detection in 3-D point clouds. All efforts have focused on manually designing specific data augmentation methods for individual architectures, however no work has attempted to automate the design of data augmentation in 3-D detection problems -- as is common in 2-D camera-based computer vision. In this work, we present a first attempt to automate the design of data augmentation policies for 3-D object detection. We describe an algorithm termed Progressive Population Based Augmentation (PPBA). PPBA learns to optimize augmentation strategies by narrowing down the search space, and adopting the best parameters discovered in previous iterations. On the KITTI test set, PPBA improves the StarNet by substantial margins on the moderate difficulty category of cars, pedestrians, and cyclists, outperforming all current state-of-the-art single-stage detection models. Additional experiments on the Waymo Open Dataset, a 20x larger dataset compared to KITTI, indicate that PPBA continues to effectively improve 3D object detection. The magnitude of the improvements may be comparable to advances in 3-D perception architectures, yet data augmentation incurs no cost at inference time. In subsequent experiments, we find that PPBA may be up to 10x more data efficient on baseline 3D detection models without augmentation, highlighting that 3D detection models may achieve competitive accuracy with far fewer labeled examples.
View details
Preview abstract
Materials design enables technologies critical to humanity, including combating climate change with solar cells and batteries. Many properties of a material are determined by its atomic crystal structure. However, prediction of the atomic crystal structure for a given material's chemical formula is a long-standing grand challenge that remains a barrier in materials design. We investigate a data-driven approach to accelerating ab initio random structure search (AIRSS), a state-of-the-art method for crystal structure search. We build a novel dataset of random structure relaxations of Li-Si battery anode materials using high-throughput density functional theory calculations. We train graph neural networks to simulate relaxations of random structures. Our model is able to find an experimentally verified structure of Li15Si4 it was not trained on, and has potential for orders of magnitude speedup over AIRSS when searching large unit cells and searching over multiple chemical stoichiometries. Surprisingly, we find that data augmentation of adding Gaussian noise improves both the accuracy and out of domain generalization of our models.
View details
Naive-Student: Leveraging semi-supervised learning in video sequences for urban scene segmentation
Liang-Chieh Chen
Rapha Gontijo Lopes
Bowen Cheng
Maxwell D. Collins
Barret Richard Zoph
Jon Shlens
European Conference on Computer Vision (ECCV)(2020)
Preview abstract
Supervised learning in large discriminative models is a mainstay for modern computer vision. Such an approach necessitates investing in large-scale, human annotated datasets for achieving state-of-the-art results. In turn, the efficacy of supervised learning may be limited by the size of the human annotated dataset. This limitation is particularly notable for image segmentation tasks where the expense of human annotation may be especially large, yet large amounts of unlabeled data may exist. In this work, we ask if we may leverage unlabeled video sequences to improve the performance on urban scene segmentation using semi-supervised learning. The goal of this work is to avoid the construction of sophisticated, learned architectures specific to label propagation (e.g., patch matching and optical flow). Instead, we simply predict pseudo-labels for the unlabeled data and train subsequent models with a mix of human-annotated and pseudo-labeled data. The procedure is iterated for several times. As a result, our model, trained with such simple yet effective iterative semi-supervised learning, attains state-of-the-art results at all three Cityscapes benchmarks, reaching the performance of 67.6% PQ, 42.4% AP, and 85.1% mIOU on the test set. We view this work as a notable step for building a simple procedure to harness unlabeled video sequences to surpass state-of-the-art performance on core computer vision tasks.
View details
FixMatch: Simplifying Semi-Supervised Learning with Consistency and Confidence
Chun-Liang Li
Colin Raffel
David Berthelot
Han Zhang
Kihyuk Sohn
NeurIPS(2020) (to appear)
Preview abstract
Semi-supervised learning (SSL) provides an effective means of leveraging unlabeled data to improve a model’s performance. This domain has seen fast progress recently, at the cost of requiring more complex methods. In this paper we proposeFixMatch, an algorithm that is a significant simplification of existing SSL methods.FixMatch first generates pseudo-labels using the model’s predictions on weakly-augmented unlabeled images. For a given image, the pseudo-label is only retained if the model produces a high-confidence prediction. The model is then trained to predict the pseudo-label when fed a strongly-augmented version of the same image. Despite its simplicity, we show that FixMatch achieves state-of-the-art performance across a variety of standard semi-supervised learning benchmarks, including 94.93% accuracy on CIFAR-10 with 250 labels and 88.61% accuracy with 40 – just 4 labels per class. We carry out an extensive ablation study to tease apart the experimental factors that are most important to FixMatch’s success
View details
SpecAugment: A Simple Augmentation Method for Automatic Speech Recognition
Daniel S. Park
William Chan
Yu Zhang
Chung-Cheng Chiu
Barret Zoph
INTERSPEECH(2019) (to appear)
Preview abstract
We present SpecAugment, a simple data augmentation method for speech recognition. SpecAugment is applied directly to the feature inputs of a neural network (i.e., filterbanks). The augmentation policy consists of warping the features, masking blocks of frequencies, and masking blocks of time steps. We apply SpecAugment on Listen, Attend and Spell networks for end-to-end speech recognition tasks. We achieve state-of-the-art performance on the Librispeech 960h and Swichboard 300h tasks, outperforming all prior work. On LibriSpeech, we achieve 6.8% WER on test-other without the use of a language model, and 5.8% WER with language model rescoring. This compares to the previous state-of-the-art hybrid system of 7.5% WER. For Switchboard, we achieve 7.2%/15.4% on the Switchboard/CallHome portion of the Hub5'00 test set without the use of a language model, which compares to the previous state-of-the-art hybrid system at 8.3%/17.3% WER.
View details