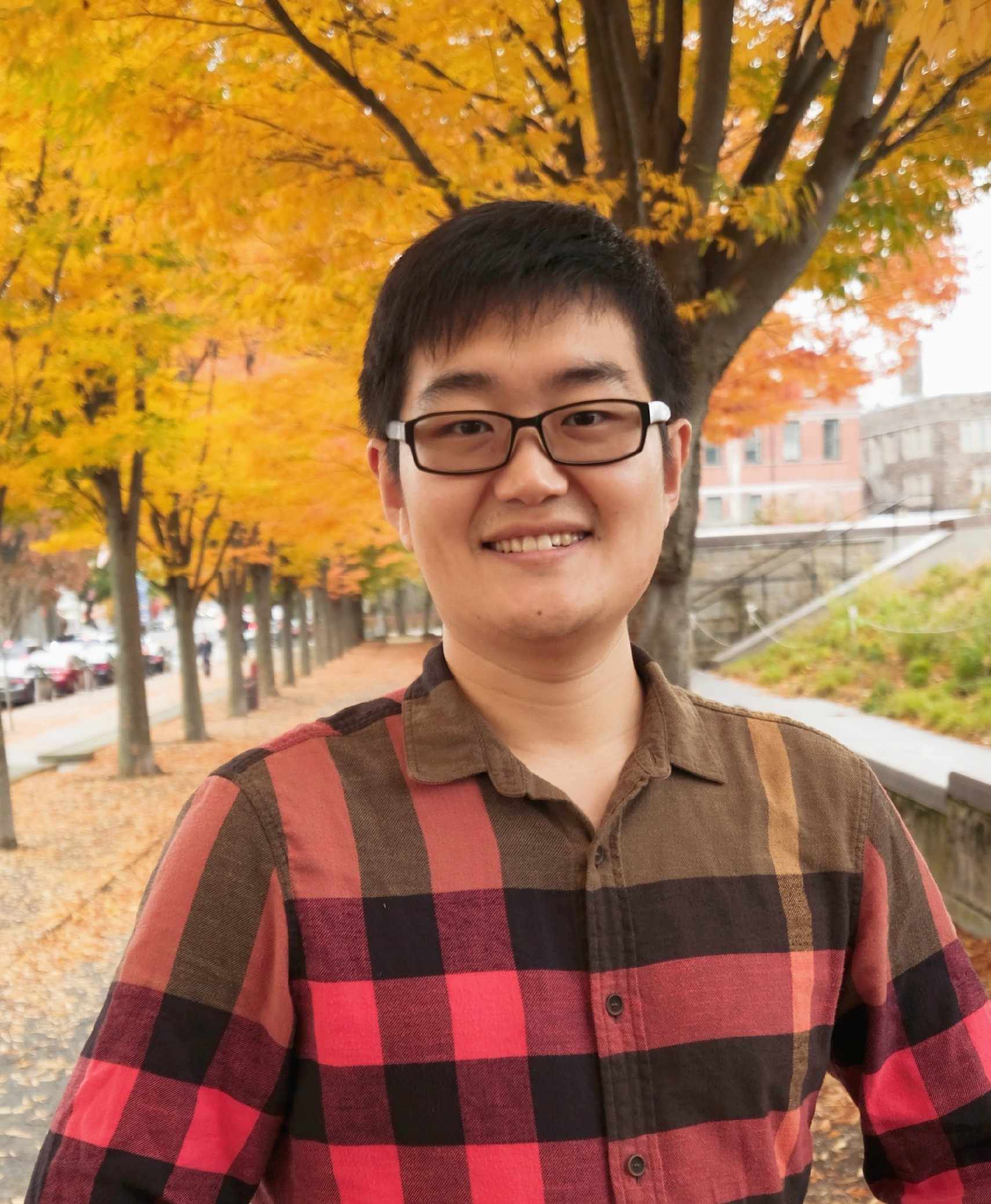
Yinda Zhang
I am a Researsh Scientist at Google.
My research interests are mostly around computer vision and computer graphics. Recently, I focus on empowering 3D vision and percetion via machine learning, including dense depth estimation, 3D shape analysis, and 3D scene understanding.
I received my Ph.D. in Computer Science from Princeton University, advised by Professor Thomas Funkhouser. Before that, I received a Bachelor degree from Dept. Automation in Tsinghua University, and a Master degree from Dept. ECE in National University of Singapore co-supervised by Prof. Ping Tan and Prof. Shuicheng Yan.
Please check my personal webpage for more.
Research Areas
Authored Publications
Sort By
ChatDirector: Enhancing Video Conferencing with Space-Aware Scene Rendering and Speech-Driven Layout Transition
Brian Moreno Collins
Karthik Ramani
Proceedings of the 2024 CHI Conference on Human Factors in Computing Systems, ACM, pp. 16 (to appear)
Preview abstract
Remote video conferencing systems (RVCS) are widely adopted in personal and professional communication. However, they often lack the co-presence experience of in-person meetings. This is largely due to the absence of intuitive visual cues and clear spatial relationships among remote participants, which can lead to speech interruptions and loss of attention. This paper presents ChatDirector, a novel RVCS that overcomes these limitations by incorporating space-aware visual presence and speech-aware attention transition assistance. ChatDirector employs a real-time pipeline that converts participants' RGB video streams into 3D portrait avatars and renders them in a virtual 3D scene. We also contribute a decision tree algorithm that directs the avatar layouts and behaviors based on participants' speech states. We report on results from a user study (N=16) where we evaluated ChatDirector. The satisfactory algorithm performance and complimentary subject user feedback imply that ChatDirector significantly enhances communication efficacy and user engagement.
View details
Experiencing InstructPipe: Building Multi-modal AI Pipelines via Prompting LLMs and Visual Programming
Zhongyi Zhou
Jing Jin
Xiuxiu Yuan
Jun Jiang
Jingtao Zhou
Yiyi Huang
Kristen Wright
Jason Mayes
Mark Sherwood
Ram Iyengar
Na Li
Extended Abstracts of the 2024 CHI Conference on Human Factors in Computing Systems, ACM, pp. 5
Preview abstract
Foundational multi-modal models have democratized AI access, yet the construction of complex, customizable machine learning pipelines by novice users remains a grand challenge. This paper demonstrates a visual programming system that allows novices to rapidly prototype multimodal AI pipelines. We first conducted a formative study with 58 contributors and collected 236 proposals of multimodal AI pipelines that served various practical needs. We then distilled our findings into a design matrix of primitive nodes for prototyping multimodal AI visual programming pipelines, and implemented a system with 65 nodes. To support users' rapid prototyping experience, we built InstructPipe, an AI assistant based on large language models (LLMs) that allows users to generate a pipeline by writing text-based instructions. We believe InstructPipe enhances novice users onboarding experience of visual programming and the controllability of LLMs by offering non-experts a platform to easily update the generation.
View details
Spectral Graphormer: Spectral Graph-based Transformer for Egocentric Two-Hand Reconstruction using Multi-View Color Images
Danhang "Danny" Tang
Franziska Müller
Jonathan Taylor
Mingsong Dou
Sasa Petrovic
Thabo Beeler
Tze Ho Elden Tse
Zhengyang Shen
Proceedings of the IEEE/CVF International Conference on Computer Vision (ICCV)(2023), pp. 14666-14677
Preview abstract
We propose a novel transformer-based framework that reconstructs two high fidelity hands from multi-view RGB images. Unlike existing hand pose estimation methods, where one typically trains a deep network to regress hand model parameters from single RGB image, we consider a more challenging problem setting where we directly regress the absolute root poses of two-hands with extended forearm at high resolution from egocentric view. As existing datasets are either infeasible for egocentric viewpoints or lack background variations, we create a large-scale synthetic dataset with diverse scenarios and collect a real dataset from multi-calibrated camera setup to verify our proposed multi-view image feature fusion strategy. To make the reconstruction physically plausible, we propose two strategies: (i) a coarse-to-fine spectral graph convolution decoder to smoothen the meshes during upsampling and (ii) an optimisation-based refinement stage at inference to prevent self-penetrations. Through extensive quantitative and qualitative evaluations, we show that our framework is able to produce realistic two-hand reconstructions and demonstrate the generalisation of synthetic-trained models to real data, as well as real-time AR/VR applications.
View details
InstructPipe: Building Visual Programming Pipelines with Human Instructions
Zhongyi Zhou
Jing Jin
Xiuxiu Yuan
Jun Jiang
Jingtao Zhou
Yiyi Huang
Kristen Wright
Jason Mayes
Mark Sherwood
Ram Iyengar
Na Li
arXiv, 2312.09672(2023)
Preview abstract
Visual programming provides beginner-level programmers with a coding-free experience to build their customized pipelines. Existing systems require users to build a pipeline entirely from scratch, implying that novice users need to set up and link appropriate nodes all by themselves, starting from a blank workspace. We present InstructPipe, an AI assistant that enables users to start prototyping machine learning (ML) pipelines with text instructions. We designed two LLM modules and a code interpreter to execute our solution. LLM modules generate pseudocode of a target pipeline, and the interpreter renders a pipeline in the node-graph editor for further human-AI collaboration. Technical evaluations reveal that InstructPipe reduces user interactions by 81.1% compared to traditional methods. Our user study (N=16) showed that InstructPipe empowers novice users to streamline their workflow in creating desired ML pipelines, reduce their learning curve, and spark innovative ideas with open-ended commands.
View details
Learning Personalized High Quality Volumetric Head Avatars from Monocular RGB Videos
Ziqian Bai
Danhang "Danny" Tang
Di Qiu
Abhimitra Meka
Mingsong Dou
Ping Tan
Thabo Beeler
2023 IEEE/CVF Conference on Computer Vision and Pattern Recognition (CVPR), IEEE
Preview abstract
We propose a method to learn a high-quality implicit 3D head avatar from a monocular RGB video captured in the wild. The learnt avatar is driven by a parametric face model to achieve user-controlled facial expressions and head poses. Our hybrid pipeline combines the geometry prior and dynamic tracking of a 3DMM with a neural radiance field to achieve fine-grained control and photorealism. To reduce over-smoothing and improve out-of-model expressions synthesis, we propose to predict local features anchored on the 3DMM geometry. These learnt features are driven by 3DMM deformation and interpolated in 3D space to yield the volumetric radiance at a designated query point. We further show that using a Convolutional Neural Network in the UV space is critical in incorporating spatial context and producing representative local features. Extensive experiments show that we are able to reconstruct high-quality avatars, with more accurate expression-dependent details, good generalization to out-of-training expressions, and quantitatively superior renderings compared to other state-of-the-art approaches.
View details
Rapsai: Accelerating Machine Learning Prototyping of Multimedia Applications through Visual Programming
Na Li
Jing Jin
Michelle Carney
Scott Joseph Miles
Maria Kleiner
Xiuxiu Yuan
Anuva Kulkarni
Xingyu “Bruce” Liu
Ahmed K Sabie
Ping Yu
Ram Iyengar
Proceedings of the 2023 CHI Conference on Human Factors in Computing Systems (CHI), ACM
Preview abstract
In recent years, there has been a proliferation of multimedia applications that leverage machine learning (ML) for interactive experiences. Prototyping ML-based applications is, however, still challenging, given complex workflows that are not ideal for design and experimentation. To better understand these challenges, we conducted a formative study with seven ML practitioners to gather insights about common ML evaluation workflows.
This study helped us derive six design goals, which informed Rapsai, a visual programming platform for rapid and iterative development of end-to-end ML-based multimedia applications. Rapsai is based on a node-graph editor to facilitate interactive characterization and visualization of ML model performance. Rapsai streamlines end-to-end prototyping with interactive data augmentation and model comparison capabilities in its no-coding environment. Our evaluation of Rapsai in four real-world case studies (N=15) suggests that practitioners can accelerate their workflow, make more informed decisions, analyze strengths and weaknesses, and holistically evaluate model behavior with real-world input.
View details
OmniSyn: Synthesizing 360 Videos with Wide-baseline Panoramas
Christian Haene
Danhang "Danny" Tang
Amitabh Varshney
2022 IEEE Conference on Virtual Reality and 3D User Interfaces (VR), IEEE
Preview abstract
Immersive maps such as Google Street View and Bing Streetside provide true-to-life views with a massive collection of panoramas. However, these panoramas are only available at sparse intervals along the path they are taken, resulting in visual discontinuities during navigation. Prior art in view synthesis is usually built upon a set of perspective images, a pair of stereoscopic images, or a monocular image, but barely examines wide-baseline panoramas, which are widely adopted in commercial platforms to optimize bandwidth and storage usage. In this paper, we leverage the unique characteristics of wide-baseline panoramas and present OmniSyn, a novel pipeline for 360° view synthesis between wide-baseline panoramas. OmniSyn predicts omnidirectional depth maps using a spherical cost volume and a monocular skip connection, renders meshes in 360° images, and synthesizes intermediate views with a fusion network. We demonstrate the effectiveness of OmniSyn via comprehensive experimental results including comparison with the state-of-the-art methods on CARLA and Matterport datasets, ablation studies, and generalization studies on street views. We envision our work may inspire future research for this unheeded real-world task and eventually produce a smoother experience for navigating immersive maps.
View details
PRIF: Primary Ray-based Implicit Function
Brandon Yushan Feng
Danhang "Danny" Tang
Amitabh Varshney
European Conference on Computer Vision (ECCV)(2022)
Preview abstract
We introduce a new implicit shape representation called Primary Ray-based Implicit Function (PRIF). In contrast to most existing approaches based on the signed distance function (SDF) which handles spatial locations, our representation operates on oriented rays. Specifically, PRIF is formulated to directly produce the surface hit point of a given input ray, without the expensive sphere-tracing operations, hence enabling efficient shape extraction and differentiable rendering. We demonstrate that neural networks trained to encode PRIF achieve successes in various tasks including single shape representation, category-wise shape generation, shape completion from sparse or noisy observations, inverse rendering for camera pose estimation, and neural rendering with color.
View details
Opportunistic Interfaces for Augmented Reality: Transforming Everyday Objects into Tangible 6DoF Interfaces Using Ad hoc UI
Mathieu Le Goc
Shengzhi Wu
Danhang "Danny" Tang
Jun Zhang
David Joseph New Tan
Extended Abstracts of the 2022 CHI Conference on Human Factors in Computing Systems, ACM
Preview abstract
Real-time environmental tracking has become a fundamental capability in modern mobile phones and AR/VR devices. However, it only allows user interfaces to be anchored at a static location. Although fiducial and natural-feature tracking overlays interfaces with specific visual features, they typically require developers to define the pattern before deployment. In this paper, we introduce opportunistic interfaces to grant users complete freedom to summon virtual interfaces on everyday objects via voice commands or tapping gestures. We present the workflow and technical details of Ad hoc UI (AhUI), a prototyping toolkit to empower users to turn everyday objects into opportunistic interfaces on the fly. We showcase a set of demos with real-time tracking, voice activation, 6DoF interactions, and mid-air gestures and prospect the future of opportunistic interfaces.
View details
HumanGPS: Geodesic PreServing Feature for Dense Human Correspondence
Danhang "Danny" Tang
Mingsong Dou
Kaiwen Guo
Cem Keskin
Sofien Bouaziz
Ping Tan
Computer Vision and Pattern Recognition 2021(2021), pp. 8
Preview abstract
In this paper, we address the problem of building dense correspondences between human images under arbitrary camera viewpoints and body poses. Prior art either assumes small motion between frames or relies on local descriptors, which cannot handle large motion or visually ambiguous body parts, e.g. left v.s. right hand. In contrast, we propose a deep learning framework that maps each pixel to a feature space, where the feature distances reflect the geodesic distances among pixels as if they were projected onto the surface of a 3D human scan. To this end, we introduce novel loss functions to push features apart according to their geodesic distances on the surface. Without any semantic annotation, the proposed embeddings automatically learn to differentiate visually similar parts and align different subjects into an unified feature space. Extensive experiments show that the learned embeddings can produce accurate correspondences between images with remarkable generalization capabilities on both intra and inter subjects.
View details