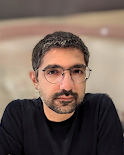
Bardia Doosti
My research interests include applying Deep Learning on vision and audio problems. Specifically I have worked on Graph-Convolutional and Convolutional Neural Networks for 3D Reconstruction and Hand and Object Pose Estimation for egocentric view images.
Authored Publications
Google Publications
Other Publications
Sort By
Spectral Graphormer: Spectral Graph-based Transformer for Egocentric Two-Hand Reconstruction using Multi-View Color Images
Danhang "Danny" Tang
Franziska Müller
Jonathan Taylor
Mingsong Dou
Sasa Petrovic
Thabo Beeler
Tze Ho Elden Tse
Zhengyang Shen
Proceedings of the IEEE/CVF International Conference on Computer Vision (ICCV) (2023), pp. 14666-14677
Preview abstract
We propose a novel transformer-based framework that reconstructs two high fidelity hands from multi-view RGB images. Unlike existing hand pose estimation methods, where one typically trains a deep network to regress hand model parameters from single RGB image, we consider a more challenging problem setting where we directly regress the absolute root poses of two-hands with extended forearm at high resolution from egocentric view. As existing datasets are either infeasible for egocentric viewpoints or lack background variations, we create a large-scale synthetic dataset with diverse scenarios and collect a real dataset from multi-calibrated camera setup to verify our proposed multi-view image feature fusion strategy. To make the reconstruction physically plausible, we propose two strategies: (i) a coarse-to-fine spectral graph convolution decoder to smoothen the meshes during upsampling and (ii) an optimisation-based refinement stage at inference to prevent self-penetrations. Through extensive quantitative and qualitative evaluations, we show that our framework is able to produce realistic two-hand reconstructions and demonstrate the generalisation of synthetic-trained models to real data, as well as real-time AR/VR applications.
View details
An Early Rico Retrospective: Three Years Of Uses For A Mobile App Dataset
Biplab Deka
Forrest Huang
Jeffrey Nichols
Artificial Intelligence for Human Computer Interaction: A Modern Approach (2021)
Preview abstract
The Rico dataset, containing design data from more than9.7k Android apps spanning 27categories, was released in 2017. It exposes visual, textual, structural, and interactive design properties of more than72k unique UI screens. Over the years since its release, the original paper has been cited nearly 100 times according to Google Scholar and the dataset has been used as the basis for numerous research projects. In this chapter, we describe the creation of Rico using a system that combined crowdsourcing and automation to scalably mine design and interaction data from Android apps at runtime. We then describe two projects that we conducted using the dataset: the training of an autoencoder to identify similarity between UI designs, and an exploration of the use of Google’s Material Design within the dataset using machine learned models. We conclude with an overview of other work that has used Rico to understand our mobile UI world and build data-driven models that assist users, designers, and developers.
View details
No Results Found