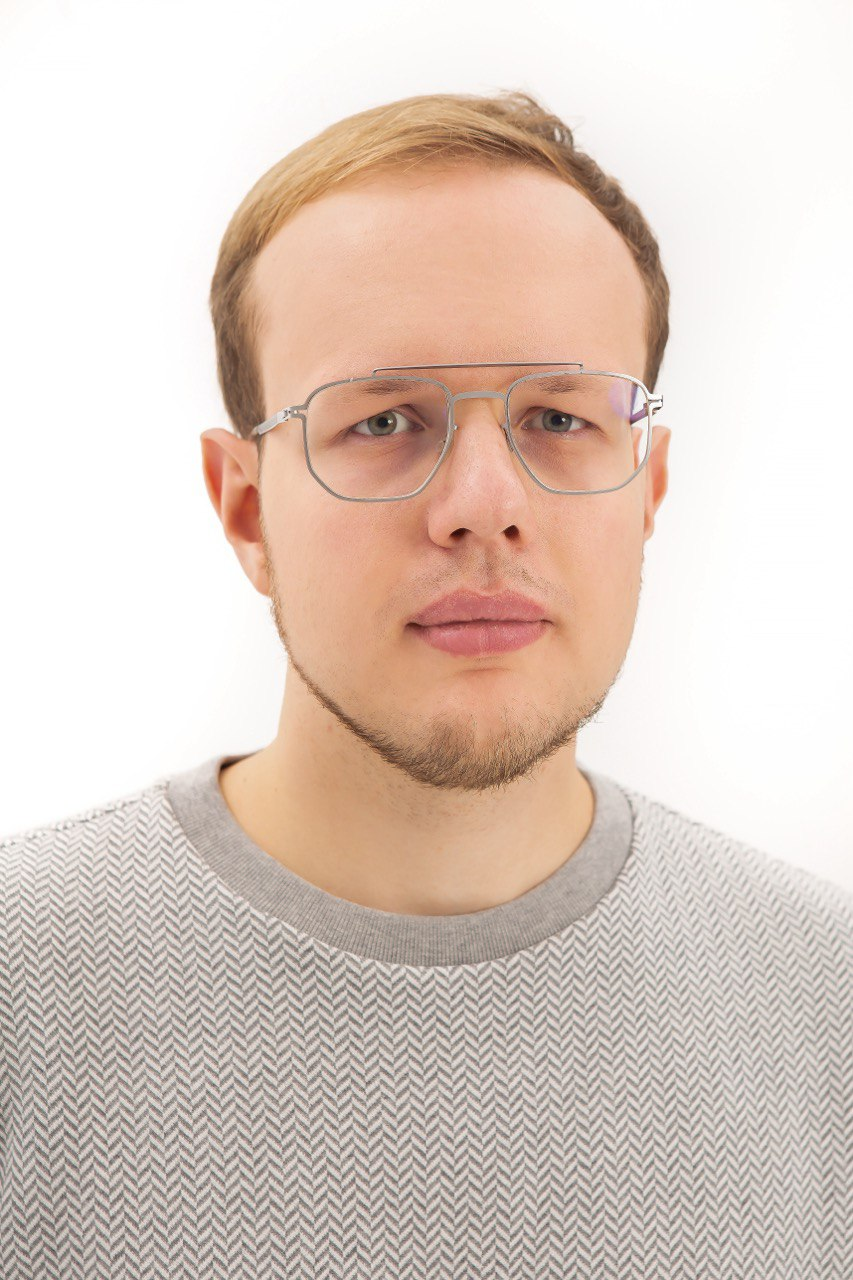
Anton Tsitsulin
Graphs, ML, math, data.
Research Areas
Authored Publications
Sort By
Tackling Provably Hard Representative Selection via Graph Neural Networks
Transactions on Machine Learning Research(2023)
Preview abstract
Representative Selection (RS) is the problem of finding a small subset of exemplars from a dataset that is representative of the dataset. In this paper, we study RS for attributed graphs, and focus on finding representative nodes that optimize the accuracy of a model trained on the selected representatives. Theoretically, we establish a new hardness result for RS (in the absence of a graph structure) by proving that a particular, highly practical variant of it (RS for Learning) is hard to approximate in polynomial time within any reasonable factor, which implies a significant potential gap between the optimum solution of widely-used surrogate functions and the actual accuracy of the model. We then study the setting where a (homophilous) graph structure is available, or can be constructed, between the data points. We show that with an appropriate modeling approach, the presence of such a structure can turn a hard RS (for learning) problem into one that can be effectively solved. To this end, we develop RS-GNN, a representation learning-based RS model based on Graph Neural Networks. Empirically, we demonstrate the effectiveness of RS-GNN on problems with predefined graph structures as well as problems with graphs induced from node feature similarities, by showing that RS-GNN achieves significant improvements over established baselines on a suite of eight benchmarks.
View details
Preview abstract
Graph Neural Networks (GNNs) have achieved state-of-the-art results on many graph analysis tasks such as node classification and link prediction. However, important unsupervised problems on graphs, such as graph clustering, have proved more resistant to advances in GNNs. Graph clustering has the same overall goal as node pooling in GNNs - does this mean that GNN pooling methods do a good job at clustering graphs?
Surprisingly, the answer is no - current GNN pooling methods often fail to recover the cluster structure in cases where simple baselines, such as k-means applied on learned representations, work well. We investigate further by carefully designing a set of experiments to study different signal-to-noise scenarios both in graph structure and attribute data. To address these methods' poor performance in clustering, we introduce Deep Modularity Networks (DMoN), an unsupervised pooling method inspired by the modularity measure of clustering quality, and show how it tackles recovery of the challenging clustering structure of real-world graphs. Similarly, on real-world data, we show that DMoN produces high quality clusters which correlate strongly with ground truth labels, achieving state-of-the-art results with over 40% improvement over other pooling methods across different metrics.
View details
HUGE: Huge Unsupervised Graph Embeddings with TPUs
SIGKDD Conference on Knowledge Discovery and Data Mining, ACM(2023) (to appear)
Preview abstract
Graphs are a representation of structured data that captures the relationships between sets of objects. With the ubiquity of available network data, there is increasing industrial and academic need to quickly analyze graphs with billions of nodes and trillions of edges. A common first step for network understanding is Graph Embedding, the process of creating a continuous representation of nodes in a graph. A continuous representation is often more amenable, especially at scale, for solving downstream machine learning tasks such as classification, link prediction, and clustering. A high-performance graph embedding architecture leveraging Tensor Processing Units (TPUs) with configurable amounts of high-bandwidth memory is presented that simplifies the graph embedding problem and can scale to graphs with billions of nodes and trillions of edges. We verify the embedding space quality on real and synthetic large-scale datasets.
View details
Unsupervised Embedding Quality Evaluation
Marina Munkhoeva
Topology, Algebra, and Geometry in Machine Learning(2023)
Preview abstract
Unsupervised learning has recently significantly gained in popularity, especially with deep learning-based approaches. Despite numerous successes and approaching supervised-level performance on a variety of academic benchmarks, it is still hard to train and evaluate SSL models in practice due to the unsupervised nature of the problem. Even with networks trained in a supervised fashion, it is often unclear whether they will perform well when transferred to another domain.
Past works have focused on assessing the amount of information contained in the embeddings. This works chooses to follow a different approach: can we quantify how easy it is to linearly separate the data in a stable way? We survey the literature and uncover three methods that could be potentially used for evaluating quality of representations. We also introduce one novel method based on recent advances in understanding the high-dimensional geometric structure self-supervised learning.
We conduct extensive experiments and study the properties of these metrics and ones introduced in the previous work. Our results suggest that while there is no free lunch, there are metrics that can robustly estimate embedding quality in an unsupervised way.
View details
GraphWorld: Fake Graphs Bring Real Insights for GNNs
Proceedings of the 28th ACM SIGKDD Conference on Knowledge Discovery and Data Mining(2022)
Preview abstract
The continuing maturity of the deep learning subfield of graph neural networks (GNNs) has motivated recent studies into the standard datasets used to benchmark GNNs. While important improvements have been made to GNN datasets and experimental design, any one dataset provides only a singular, potentially spurious insight into the performance of any GNN being tested. We show that state-of-the-art GNN task datasets do not cover the distribution of graphs in a much larger real-data graph repository, with respect to several key graph metrics. Motivated by this finding, we introduce GraphWorld, a novel distributed framework and software package for testing GNN models on an arbitrarily-large population of \emph{synthetic} task datasets.
GraphWorld allows a user to efficiently generate a \emph{world} of millions of graph datasets, with fine-grained control over graph generator parameters, and benchmark arbitrary GNN models, with built-in hyperparameter tuning. Using GraphWorld to generate diverse graph worlds corresponding to node classification, graph classification, and link prediction tasks, we provide insight into the sensitivity of 10,000+ GNN models to various parameters of graphs and node features and} show comparisons between models that have not been possible to make in any previous work. We also introduce a novel metric with which to explore each models' performance on the graph world, conditioning on graph metrics and graph generator parameters.
View details
Differentially Private Graph Learning via Sensitivity-Bounded Personalized PageRank
Advances in Neural Information Processing Systems(2022)
Preview abstract
Personalized PageRank (PPR) is a fundamental tool in unsupervised learning of graph representations such as node ranking, labeling, and graph embedding. However, while data privacy is one of the most important recent concerns, existing PPR algorithms are not designed to protect user privacy. PPR is highly sensitive to the input graph edges: the difference of only one edge may cause a big change in the PPR vector, potentially leaking private user data.
In this work, we propose an algorithm which outputs an approximate PPR and has provably bounded sensitivity to input edges. In addition, we prove that our algorithm achieves similar accuracy to non-private algorithms when the input graph has large degrees. Our sensitivity-bounded PPR directly implies private algorithms for several tools of graph learning, such as, differentially private (DP) PPR ranking, DP node classification, and DP node embedding. To complement our theoretical analysis, we also empirically verify the practical performances of our algorithms.
View details
Preview abstract
Graph Neural Networks (GNNs) have achieved state-of-the-art results on many graph analysis tasks such as node classification and link prediction. However, important unsupervised problems on graphs, such as graph clustering, have proved more resistant to advances in GNNs. In this paper, we study unsupervised training of GNN pooling in terms of their clustering capabilities.
We start by drawing a connection between graph clustering and graph pooling: intuitively, a good graph clustering is what one would expect from a GNN pooling layer. Counterintuitively, we show that this is not true for state-of-the-art pooling methods, such as MinCut pooling. To address these deficiencies, we introduce Deep Modularity Networks (DMoN), an unsupervised pooling method inspired by the modularity measure of clustering quality, and show how it tackles recovery of the challenging clustering structure of real-world graphs. In order to clarify the regimes where existing methods fail, we carefully design a set of experiments on synthetic data which show that DMoN is able to jointly leverage the signal from the graph structure and node attributes. Similarly, on real-world data, we show that DMoN produces high quality clusters which correlate strongly with ground truth labels, achieving state-of-the-art results.
View details