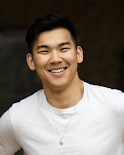
Alexander Ku
Research Areas
Authored Publications
Google Publications
Other Publications
Sort By
A New Path: Scaling Vision-and-Language Navigation with Synthetic Instructions and Imitation Learning
Aishwarya Kamath
Peter Anderson
Jing Yu Koh
Yinfei Yang
Zarana Parekh
CVPR (2023)
Preview abstract
Recent studies in Vision-and-Language Navigation (VLN) train RL agents to execute natural-language navigation instructions in photorealistic environments, as a step towards robots that can follow human instructions. However, given the scarcity of human instruction data and limited diversity in the training environments, these agents still struggle with complex language grounding and spatial language understanding. Pre-training on large text and image-text datasets from the web has been extensively explored but the improvements are limited. We investigate large-scale augmentation with synthetic instructions. We take 500+ indoor environments captured in densely-sampled 360 degree panoramas, construct navigation trajectories through these panoramas, and generate a visually-grounded instruction for each trajectory using Marky, a high-quality multilingual navigation instruction generator. We also synthesize image observations from novel viewpoints using an image-to-image GAN. The resulting dataset of 4.2M instruction-trajectory pairs is two orders of magnitude larger than existing human-annotated datasets, and contains a wider variety of environments and viewpoints. To efficiently leverage data at this scale, we train a simple transformer agent with imitation learning. On the challenging RxR dataset, our approach outperforms all existing RL agents, improving the state-of-the-art NDTW from 71.1 to 79.1 in seen environments, and from 64.6 to 66.8 in unseen test environments. Our work points to a new path to improving instruction-following agents, emphasizing large-scale training on near-human quality synthetic instructions.
View details
On the Evaluation of Vision-and-Language Navigation Instructions
Ming Zhao
Peter Anderson
Vihan Jain
Conference of the European Chapter of the Association for Computational Linguistics (EACL) (2021)
PanGEA: The Panoramic Graph Environment Annotation Toolkit
Peter Anderson
2nd Workshop on Advances in Language and Vision Research (ALVR) (2021)
Preview abstract
PanGEA, the Panoramic Graph Environment Annotation toolkit, is a lightweight toolkit for collecting speech and text annotations in photo-realistic 3D environments. PanGEA immerses annotators in a web-based simulation and allows them to move around easily as they speak and/or listen. It includes database and cloud storage integration, plus utilities for automatically aligning recorded speech with manual transcriptions and the virtual pose of the annotators. Out of the box, PanGEA supports two tasks -- collecting navigation instructions and navigation instruction following -- and it could be easily adapted for annotating walking tours, finding and labeling landmarks or objects, and similar tasks. We share best practices learned from using PanGEA in a 20,000 hour annotation effort to collect the Room-Across-Room (RxR) dataset. We hope that our open-source annotation toolkit and insights will both expedite future data collection efforts and spur innovation on the kinds of grounded language tasks such environments can support.
View details
Preview abstract
We introduce Room-Across-Room (RxR), a new Vision-and-Language Navigation (VLN) dataset. RxR is multilingual (English, Hindi, and Telugu) and larger (more paths and instructions) than other VLN datasets. It emphasizes the role of language in VLN by addressing known biases in paths and eliciting more references to visible entities. Furthermore, each word in an instruction is time-aligned to the virtual poses of instruction creators and validators. We establish baseline scores for monolingual and multilingual settings and multitask learning when including Room-to-Room annotations. We also provide results for a model that learns from synchronized pose traces by focusing only on portions of the panorama attended to in human demonstrations. The size, scope and detail of RxR dramatically expands the frontier for research on embodied language agents in simulated, photo-realistic environments.
View details
Stay on the Path: Instruction Fidelity in Vision-and-Language Navigation
Vihan Jain
Gabriel Magalhaes
Ashish Vaswani
Association for Computational Linguistics (2019)
Preview abstract
Advances in learning and representations have reinvigorated work that connects language to other modalities. A particularly exciting direction is Vision-and-Language Navigation (VLN), in which agents interpret natural language instructions and visual scenes to move through environments and reach goals. Despite recent progress, current research leaves unclear how much of a role language understanding plays in this task, especially because dominant evaluation metrics have focused on goal completion rather than the sequence of actions corresponding to the instructions. Here, we highlight shortcomings of current metrics for the Room-to-Room dataset (Anderson et al. 2018b) and propose a new metric, Coverage weighted by Length Score (CLS). We also show that the existing paths in the dataset are not ideal for evaluating instruction following because they are direct-to-goal shortest paths. We join existing short paths to form more challenging extended paths to create a new data set, Room-for-Room (R4R). Using R4R and CLS, we show that agents that receive rewards for instruction fidelity outperform agents that focus on goal completion.
View details
General Evaluation for Instruction Conditioned Navigation using Dynamic Time Warping
Gabriel Ilharco Magalhaes
Vihan Jain
NeurIPS Visually Grounded Interaction and Language (ViGIL) Workshop (2019)
Transferable Representation Learning in Vision-and-Language Navigation
Haoshuo Huang
Vihan Jain
Gabriel Ilharco Magalhaes
ICCV 2019 (2019)
Image Transformer
Niki J. Parmar
Ashish Vaswani
Jakob Uszkoreit
Lukasz Kaiser
Noam Shazeer
International Conference on Machine Learning (ICML) (2018)
Preview abstract
Recent work demonstrated significant progress towards modeling the distribution of natural images with tractable likelihood using deep neural networks. This was achieved by modeling the joint distribution of pixels in the image as the product of conditional distributions, thereby turning it into a sequence modeling problem, and applying recurrent or convolutional neural networks to it.
In this work we instead build on the Transformer, a recently proposed network architecture based on self-attention, to model the conditional distributions in similar factorizations. We present two extensions of the network architecture, allowing it to scale to images and to take advantage of their two-dimensional structure.
While conceptually simple, our generative models trained on two image data sets are competitive with or outperform the current state of the art on two different data sets, CIFAR-10 and ImageNet, as measured by log-likelihood.
We also present results on image super-resolution with large magnification ratio with an encoder-decoder configuration of our architecture. In a human evaluation study, we show that our super-resolution models improve over previously published autoregressive super-resolution models in how often they fool a naive human observer by a factor of three.
Lastly, we provide examples of images generated or completed by our various models which, following previous work, we also believe to look pretty cool.
View details
A universal SNP and small-indel variant caller using deep neural networks
Scott Schwartz
Dan Newburger
Jojo Dijamco
Nam Nguyen
Pegah T. Afshar
Sam S. Gross
Lizzie Dorfman
Mark A. DePristo
Nature Biotechnology (2018)
Preview abstract
Despite rapid advances in sequencing technologies, accurately calling genetic variants present in an individual genome from billions of short, errorful sequence reads remains challenging. Here we show that a deep convolutional neural network can call genetic variation in aligned next-generation sequencing read data by learning statistical relationships between images of read pileups around putative variant and true genotype calls. The approach, called DeepVariant, outperforms existing state-of-the-art tools. The learned model generalizes across genome builds and mammalian species, allowing nonhuman sequencing projects to benefit from the wealth of human ground-truth data. We further show that DeepVariant can learn to call variants in a variety of sequencing technologies and experimental designs, including deep whole genomes from 10X Genomics and Ion Ampliseq exomes, highlighting the benefits of using more automated and generalizable techniques for variant calling.
View details
No Results Found