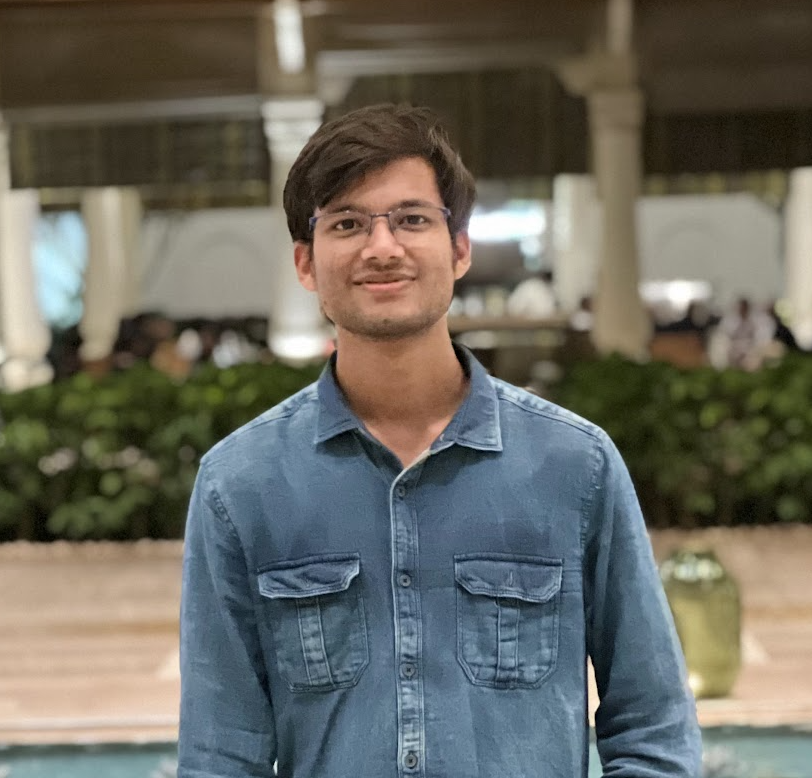
Rishabh Tiwari
Rishabh Tiwari is a Pre-Doctoral Researcher at Google Research, India. His research interest lies in the broad field of Efficient Deep Learning. Recent work involves mitigating simplicity bias without using bias labels, network architecture optimization by developing novel network pruning algorithm. Rishabh is also a founding member and advisor of Transmute AI Labs, a non profit research lab, where he guides undergrad students to pursue research.
Research Areas
Authored Publications
Sort By
Using Early Readouts to Mediate Featural Bias in Distillation
Durga Sivasubramanian
Anmol Mekala
Ganesh Ramakrishnan
WACV 2024(2024)
Preview abstract
Deep networks tend to learn spurious feature-label correlations in real-world supervised learning tasks. This vulnerability is aggravated in distillation, where a (student) model may have less representational capacity than the corresponding teacher model. Often, knowledge of specific problem features is used to reweight instances & rebalance the learning process. We propose a novel early readout mechanism whereby we attempt to predict the label using representations from earlier network layers. We show that these early readouts automatically identify problem instances or groups in the form of confident, incorrect predictions. We improve group fairness measures across benchmark datasets by leveraging these signals to mediate between teacher logits and supervised label. We extend our results to the closely related but distinct problem of domain generalization, which also critically depends on the quality of learned features. We provide secondary analyses that bring insight into the role of feature learning in supervision and distillation.
View details
Preview abstract
Simplicity bias is the concerning tendency of deep networks to over-depend on simple, weakly predictive features, to the exclusion of stronger, more complex features. This causes biased, incorrect model predictions in many real-world applications when trained on incomplete data containing spurious feature-label correlations. We propose a direct, interventional method for addressing simplicity bias in DNNs, called the feature sieve. We aim to automatically identify and suppress easily-computable features in lower layers of the network, thereby allowing the higher network levels to extract and utilize richer, more meaningful representations. We provide concrete evidence of this differential suppression & enhancement of features using controlled datasets, and report substantial gains on many real-world debiasing benchmarks (11.4\% relative gain on ImageNet-A; 3.2% on BAR, etc). Crucially, we outperform many baselines that incorporate knowledge about ``simple'' features, or known spurious attributes, despite our method not using any such information. We believe that our feature sieve work opens up exciting new research directions in automatic adversarial feature extraction techniques for deep networks.
View details
Preview abstract
Concept bottleneck models (CBMs) (Koh et al. 2020) are interpretable neural networks that first predict labels for human-interpretable concepts relevant to the prediction task, and then predict the final label based on the concept label predictions. We extend CBMs to interactive prediction settings where the model can query a human collaborator for the label to some concepts. We develop an interaction policy that, at prediction time, chooses which concepts to request a label for so as to maximally improve the final prediction. We demonstrate that a simple policy combining concept prediction uncertainty and influence of the concept on the final prediction achieves strong performance and outperforms a static approach proposed in Koh et al. (2020) as well as active feature acquisition methods proposed in the literature. We show that the interactive CBM can achieve accuracy gains of 5-10% with only 5 interactions over competitive baselines on the Caltech UCSB Birds dataset and the Chexpert dataset.
View details
GCR: Gradient coreset based replay buffer selection for continual learning
Krishnateja Killamsetty
Rishabh Iyer
Proceedings of the IEEE/CVF Conference on Computer Vision and Pattern Recognition(2022), pp. 99-108
Preview abstract
Continual learning (CL) aims to develop techniques by which a single model adapts to an increasing number of tasks encountered sequentially, thereby potentially leveraging learnings across tasks in a resource-efficient manner. A major challenge for CL systems is catastrophic forgetting, where earlier tasks are forgotten while learning a new task. To address this, replay-based CL approaches maintain and repeatedly retrain on a small buffer of data selected across encountered tasks. We propose Gradient Coreset Replay, a novel strategy for replay buffer selection and update using a carefully designed optimization criterion. Specifically, we select and maintain a “coreset” that closely approximates the gradient of all the data seen so far with respect to current model parameters, and discuss key strategies needed for its effective application to the continual learning setting. We show significant gains (2%-4%) over the state-of-the-art in the well-studied offline continual learning setting. Our findings also effectively transfer to online / streaming CL settings, showing up to 5% gains over existing approaches. Finally, we demonstrate the value of supervised contrastive loss for continual learning, which yields a cumulative gain of up to 5% accuracy when combined with our subset selection strategy.
View details