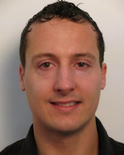
Richard Zens
Authored Publications
Google Publications
Other Publications
Sort By
Content Explorer: Recommending Novel Entities for a Document Writer
Proceedings of Empirical Methods of Natural Language Processing, EMNLP, 2018.
Preview abstract
Background research is an inseparable part of document writing. Search engines are great for retrieving information once we know what to look for. However, the bigger challenge is often identifying topics for further research.
Automated tools could help significantly in this discovery process and increase the productivity of the writer.
In this paper, we formulate the problem of recommending topics to a writer.
We formulate this as a supervised learning problem and run a user study to validate this approach.
We propose an evaluation metric and perform an empirical comparison of state-of-the-art models for extreme multi-label classification on a large data set.
We demonstrate how a simple modification of the cross-entropy loss function leads to improved results of the deep learning models.
View details
A Systematic Comparison of Phrase Table Pruning Techniques
Peng Xu
Proceedings of the 2012 Joint Conference on Empirical Methods in Natural Language Processing and Computational Natural Language Learning, Association for Computational Linguistics, Jeju Island, Korea, pp. 972-983
Preview abstract
When trained on very large parallel corpora, the phrase table component of a machine translation system grows to consume vast computational resources. In this paper, we introduce a novel pruning criterion that places phrase table pruning on a sound theoretical foundation. Systematic experiments on four language pairs under various data conditions show that our principled approach is superior to existing ad hoc pruning methods.
View details
Fast and Scalable Decoding with Language Model Look-Ahead for Phrase-based Statistical Machine Translation
Joern Wuebker
Hermann Ney
Proceedings of the 50th Annual Meeting of the Association for Computational Linguistics, Association for Computational Linguistics, Jeju, Republic of Korea (2012), pp. 28-32
Preview abstract
In this work we present two extensions to
the well-known dynamic programming beam
search in phrase-based statistical machine
translation (SMT), aiming at increased effi-
ciency of decoding by minimizing the number
of language model computations and hypothesis expansions. Our results show that language
model based pre-sorting yields a small improvement in translation quality and a speedup
by a factor of 2. Two look-ahead methods are
shown to further increase translation speed by
a factor of 2 without changing the search space
and a factor of 4 with the side-effect of some
additional search errors. We compare our approach with Moses and observe the same performance, but a substantially better trade-off
between translation quality and speed. At a
speed of roughly 70 words per second, Moses
reaches 17.2% BLEU, whereas our approach
yields 20.0% with identical models.
View details
Improvements for Beam Search in Statistical Machine Translation
Oliver Bender
Hermann Ney
Handbook of Natural Language Processing and Machine Translation, Springer (2011)
Name extraction and translation for distillation
Heng Ji
Ralph Grishman
Dayne Freitag
Matthias Blume
John Wang
Shahram Khadivi
Hermann Ney
Handbook of Natural Language Processing and Machine Translation: DARPA Global Autonomous Language Exploitation (2009)
Improvements in Dynamic Programming Beam Search for Phrase-based Statistical Machine Translation
Hermann Ney
Proceedings of the International Workshop on Spoken Language Translation, Honolulu, HI (2008), pp. 195-205
Efficient Speech Translation through Confusion Network Decoding
Nicola Bertoldi
Marcello Federico
Wade Shen
IEEE Transactions on Audio, Speech and Language Processing, vol. 16 (2008), pp. 1696-1705
Moses: Open source toolkit for statistical machine translation
Philipp Koehn
Hieu Hoang
Alexandra Birch
Chris Callison-Burch
Marcello Federico
Nicola Bertoldi
Brooke Cowan
Wade Shen
Christine Moran
Chris Dyer
Ondrej Bojar
Alexandra Constantin
Evan Herbst
Proceedings of the 45th Annual Meeting of the ACL - Demo and Poster Sessions, Association for Computational Linguistics, Prague, Czech Republic (2007), pp. 177-180
Chunk-level reordering of source language sentences with automatically learned rules for statistical machine translation
Yuqi Zhang
Hermann Ney
Proceedings of the NAACL-HLT 2007/AMTA Workshop on Syntax and Structure in Statistical Translation, Association for Computational Linguistics, Rochester, New York, pp. 1-8
Speech translation by confusion network decoding
Nicola Bertoldi
Marcello Federico
2007 IEEE International Conference on Acoustics, Speech and Signal Processing - ICASSP '07, Honolulu, Hawaii, USA, IV-1297-1300
Improved chunk-level reordering for statistical machine translation
Efficient Phrase-table Representation for Machine Translation with Applications to Online MT and Speech Translation
Hermann Ney
Proceedings of the Human Language Technology Conference of the North American Chapter of the Association for Computational Linguistics (HLT-NAACL), ACL, Rochester, NY (2007), pp. 492-499
A Systematic Comparison of Training Criteria for Statistical Machine Translation
Sasa Hasan
Hermann Ney
Proceedings of the Conference on Empirical Methods in Natural Language Processing (EMNLP), ACL, Prague, Czech Republic (2007), pp. 524-532
Minimum Bayes risk decoding for BLEU
The JHU workshop 2006 IWSLT system
The RWTH statistical machine translation system for the IWSLT 2006 evaluation
N-Gram Posterior Probabilities for Statistical Machine Translation
Hermann Ney
Human Language Technology Conference of the North American Chapter of the Association for Computational Linguistics (HLT-NAACL): Proceedings of the Workshop on Statistical Machine Translation, ACL, New York City, NY (2006), pp. 72-77
Discriminative Reordering Models for Statistical Machine Translation
Hermann Ney
Human Language Technology Conference of the North American Chapter of the Association for Computational Linguistics (HLT-NAACL): Proceedings of the Workshop on Statistical Machine Translation, ACL, New York City, NY (2006), pp. 55-63
Word graphs for statistical machine translation
Hermann Ney
Proceedings of the ACL Workshop on Building and Using Parallel Texts (2005), pp. 191-198
The RWTH Phrase-based Statistical Machine Translation System
Oliver Bender
Sasa Hasan
Shahram Khadivi
Evgeny Matusov
Jia Xu
Yuqi Zhang
Hermann Ney
IWSLT (2005)
Novel reordering approaches in phrase-based statistical machine translation
Stephan Kanthak
David Vilar
Evgeny Matusov
Hermann Ney
Proceedings of the ACL Workshop on Building and Using Parallel Texts, Association for Computational Linguistics, Ann Arbor, Michigan, USA (2005), pp. 167-174
Improved Word Alignment Using a Symmetric Lexicon Model
Evgeny Matusov
Hermann Ney
Proceedings of the 20th International Conference on Computational Linguistics (Coling), Geneva, Switzerland (2004), pp. 36-42
Reordering Constraints for Phrase-Based Statistical Machine Translation
Hermann Ney
Taro Watanabe
Eiichiro Sumita
Proceedings of the 20th International Conference on Computational Linguistics (Coling), Geneva, Switzerland (2004), pp. 205-211
Symmetric word alignments for statistical machine translation
Evgeny Matusov
Hermann Ney
COLING '04 Proceedings of the 20th international conference on Computational Linguistics, Association for Computational Linguistics, Geneva, Switzerland (2004)
Alignment templates: the RWTH SMT system
Improvements in phrase-based statistical machine translation
Hermann Ney
Proceedings of HLT-NAACL, Association for Computational Linguistics, Boston, MA (2004), pp. 257-264
A Comparative Study on Reordering Constraints in Statistical Machine Translation
Hermann Ney
Proceedings of the Annual Meeting of the Association for Computational Linguistics (ACL), ACL, Sapporo, Japan (2003), pp. 144-151
Efficient Search for Interactive Statistical Machine Translation
Franz Josef Och
Hermann Ney
Proceedings of the tenth conference of the European chapter of the Association for Computational Linguistics (EACL), Budapest, Hungary (2003), pp. 387-394
Phrase-Based Statistical Machine Translation