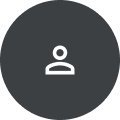
Michael Dikovsky
Physicist by education, Michael Dikovsky is interested in diverse areas of science and engineering: Software Engineering, Mathematical Physics and Scientific Computing, Plasma Physics, Spectroscopy, Quantum Mechanics, Clean Energy, Power Grid, Electronics, FPGAs, Compilers, Formal Logic, Deterministic and Stochastic Optimization Methods, Multi-physics Simulation and Modeling of Complex Systems.
Current research focus is on understanding behavior of nuclear fusion plasma using advanced bayesian inference methods.
Authored Publications
Google Publications
Other Publications
Sort By
Preview abstract
The Hamiltonian Monte Carlo (HMC) method allows sampling from continuous densities. Favorable scaling with dimension has led to wide adoption of HMC by the statistics community. Modern auto-differentiating software should allow more widespread usage in Bayesian inverse problems. This paper analyzes the two major difficulties encountered using HMC for inverse problems: poor conditioning and multi-modality. Novel results on preconditioning and replica exchange Monte Carlo parameter selection are presented in the context of spectroscopy. Recommendations are analyzed rigorously in the Gaussian case, and shown to generalize in a fusion plasma reconstruction.
View details
Multi-instrument Bayesian reconstruction of plasma shape evolution in C-2W experiment
Erik Trask
Hiroshi Gota
Jesus Romero
Rob von Behren
Tom Madams
Physics of Plasmas (2021)
Preview abstract
We determined the time-dependent geometry including high-frequency oscillations of the plasma density in TAE’s C2W experiment. This was done as a joint Bayesian reconstruction from a 14-chord FIR interferometer in the midplane, 32 Mirnov probes at the periphery, and 8 shine-through detectors at the targets of the neutral beams. For each point in time we recovered, with credibility intervals: the radial density profile of the plasma; bulk plasma displacement; amplitudes, frequencies and phases of the azimuthal modes n=1 to n=4. Also reconstructed were the radial profiles of the deformations associated with each of the azimuthal modes. Bayesian posterior sampling was done via Hamiltonian
Monte Carlo with custom preconditioning. This gave us a comprehensive uncertainty quantification of the reconstructed values, including correlations and some understanding of multimodal posteriors. This method was applied to thousands of experimental shots on C-2W, producing a rich data set for analysis of plasma performance.
View details
Comprehensive Imaging of C-2W Plasmas: Instruments and Applications
Erik Granstedt
Deepak Gupta
James Sweeney
Matthew Tobin
the TAE team
Review of Scientific Instruments, vol. 92 (2021), pp. 043515
Preview abstract
The C-2W device (“Norman”), has produced and sustained beam-driven field-reversed configuration (FRC) plasmas embedded in a magnetic mirror geometry using neutral beams and end-bias electrodes located in expander divertors. Many discrete vessels comprise this device, and a suite of spatially and radiometrically calibrated, high-speed camera systems have been deployed to visualize the plasma throughout.
Besides global visualization of the plasma evolution, this imaging suite has been used in a variety of applications. Reconstruction of the magnetic field in the equilibrium vessel is complicated by eddy currents in conducting structures and thus far, non-perturbative measurements of internal field have not been available. Tomographic reconstruction of O4+ impurity emission provides an independent check of magnetic modeling and indirect evidence for field reversal within the FRC.
Voltages up to 3.5 kV are applied to electrodes in the expander divertors to control the radial electric field in the plasma located on open field-lines. This has been shown to improve the macroscopic stability of the FRC; however, a full model for how electrode potentials propagate to the center of the plasma is the subject of ongoing work. Imaging in the expander divertors is used to study gas ionization and to identify metal arcing from electrode surfaces.
View details
Modeling the effect of exposure notification and non-pharmaceutical interventions on COVID-19 transmission in Washington state
Matthew Abueg
Robert Hinch
Neo Wu
William Probert
Austin Wu
Paul Eastham
Yusef Shafi
Matt Rosencrantz
Zhao Cheng
Anel Nurtay
Lucie Abeler-Dörner
David Bonsall
Michael V. McConnell
Shawn O'Banion
Christophe Fraser
npj Digital Medicine (2021)
Preview abstract
Contact tracing is increasingly used to combat COVID-19, and digital implementations are now being deployed, many based on Apple and Google’s Exposure Notification System. These systems utilize non-traditional smartphone-based technology, presenting challenges in understanding possible outcomes. In this work, we create individual-based models of three Washington state counties to explore how digital exposure notifications combined with other non-pharmaceutical interventions influence COVID-19 disease spread under various adoption, compliance, and mobility scenarios. In a model with 15% participation, we found that exposure notification could reduce infections and deaths by approximately 8% and 6% and could effectively complement traditional contact tracing. We believe this can provide health authorities in Washington state and beyond with guidance on how exposure notification can complement traditional interventions to suppress the spread of COVID-19.
View details
Preview abstract
Hamiltonian Monte Carlo is discussed in the context of a fusion plasma reconstruction. Ill conditioned covariance and multi-modality are discussed in depth.
View details
OVERVIEW OF C-2W: HIGH TEMPERATURE, STEADY-STATE BEAM-DRIVEN FIELD-REVERSED CONFIGURATION PLASMAS
Rob von Behren
TAE
Tom Madams
William D Heavlin
Nuclear Fusion (2021)
Preview abstract
TAE Technologies, Inc. (TAE) is pursuing an alternative approach to magnetically confined fusion, which relies on field-reversed configuration (FRC) plasmas composed of mostly energetic and well-confined particles by means of a state-of-the-art tunable energy neutral-beam (NB) injector system. TAE’s current experimental device, C-2W (also called “Norman”), is the world’s largest compact-toroid device and has made significant progress in FRC performance, producing record breaking, high temperature (electron temperature, Te >500 eV; total electron and ion temperature, Ttot >3 keV) advanced beam-driven FRC plasmas, dominated by injected fast particles and sustained in steady-state for up to 30 ms, which is limited by NB pulse duration. C-2W produces significantly better FRC performance than the preceding C-2U experiment, in part due to Google’s machine-learning framework for experimental optimization, which has contributed to the discovery of a new operational regime where novel settings for the formation sections yield consistently reproducible, hot, and stable plasmas. Active plasma control system has been developed and utilized in C-2W to produce consistent FRC performance as well as for reliable machine operations using magnets, electrodes, gas injection, and tunable NBs. The active control system has demonstrated a stabilization of FRC axial instability. Overall FRC performance is well correlated with NBs and edge-biasing system, where higher total plasma energy is obtained with increasing both NB injection power and applied-voltage on biasing electrodes. C-2W divertors have demonstrated a good electron heat confinement on open-field-lines using strong magnetic mirror fields as well as expanding the magnetic field in the divertors (expansion ratio >30); the electron energy lost per ion, ~6–8, is achieved, which is close to the ideal theoretical minimum.
View details
Fusion Plasma Reconstruction
Nathan Neibauer
Rob von Behren
(2019)
Preview abstract
Fusion Plasma Reconstruction work done at Google in partnership with TAE is presented.
View details
Preview abstract
Hamiltonian Monte Carlo is a popular sampling technique for smooth target densities. The scale lengths of the target have long been known to influence integration error and sampling efficiency. However, quantitative measures intrinsic to the target have been lacking. In this paper, we restrict attention to the multivariate Gaussian and the leapfrog integrator, and obtain a condition number corresponding to sampling efficiency. This number, based on the spectral and Schatten norms, quantifies the number of leapfrog steps needed to efficiently sample. We demonstrate its utility by using this condition number to analyze HMC preconditioning techniques. We also find the condition number of large inverse Wishart matrices, from which we derive burn-in heuristics.
View details
Application of Bayesian inference for reconstruction of FRC plasma state in C-2W
Erik Trask
Hiroshi Gota
Jesus Romero
Matthew Thompson
Tom Madams
Yair Carmon
(2018)
Preview abstract
Bayesian methods are used to infer Field Reversed Configuration (FRC) plasma properties for the C-2W machine at TAE Technologies. The approach starts with a statistical distribution of possible plasma states, where physically-motivated constraints are imposed through the Bayesian prior. Possible states are processed by a forward model for the relevant instruments to assess agreement with corresponding measured experimental data. The resulting probability distribution is known as the posterior, from which the most likely plasma state and the corresponding statistical confidence are extracted. Plasma state reconstruction from multi-instrument Bayesian inference are presented in this study, implemented for the upgraded diagnostics that have come online for C-2W. FIR interferometry, Thomson scattering, Bremsstrahlung radiation measurement, and secondary electron emission detection from the neutral beams are used in reconstruction near the FRC midplane. Magnetic probes and imaging from a high-speed camera provide 3D data throughout the main confinement vessel. This study aims to further the understanding of plasma properties and dynamics, such as electron and ion densities, electron temperature, plasma current, and magnetic field topology.
View details
The Plasma Debugger
Erik Granstedt
Erik Trask
Hiroshi Gota
Jesus Romero
Matthew Thompson
Roberto Mendoza
Tom Madams
Yair Carmon
(2018)
Preview abstract
We built a "Plasma Debugger", a tool to reconstruct the state of the FRC plasma in the TAE Technologies' experimental machine C2W. This generalized Bayesian inference approach combines data from magnetic sensors, fast cameras, FIR interferometer, Thomson Scattering system, Bremsstrahlung measurements and neutral beams shine-through SEE detectors. It then reconstructs electron density, temperature and bulk plasma currents, with confidence intervals. Computation takes hundreds of CPUs and is performed in the cloud, with results showing up in the plasma machine control room within several minutes of the experiment. The display shows time evolution of the basic plasma properties, giving machine operators additional insight into the plasma behavior.
View details
Achievement of Sustained Net Plasma Heating in a Fusion Experiment with the Optometrist Algorithm
E. Trask
M. Binderbauer
H. Gota
R. Mendoza
P.F. Riley
Scientific Reports, vol. 7 (2017), pp. 6425
Preview abstract
Many fields of basic and applied science require efficiently exploring complex systems with high dimensionality. An example of such a challenge is optimising the performance of plasma fusion experiments. The highly-nonlinear and temporally-varying interaction between the plasma, its environment and external controls presents a considerable complexity in these experiments. A further difficulty arises from the fact that there is no single objective metric that fully captures both plasma quality and equipment constraints.
To efficiently optimise the system, we develop the Optometrist Algorithm, a stochastic perturbation method combined with human choice. Analogous to getting an eyeglass prescription, the Optometrist Algorithm confronts a human operator with two alternative experimental settings and associated outcomes. A human operator then chooses which experiment produces subjectively better results. This innovative technique led to the discovery of an unexpected record confinement regime with positive net heating power in a field-reversed configuration plasma, characterised by a >50% reduction in the energy loss rate and concomitant increase in ion temperature and total plasma energy.
View details
No Results Found