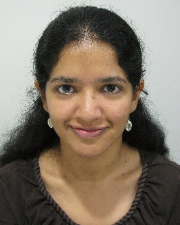
Kshipra Bhawalkar
Research Areas
Authored Publications
Sort By
Simple Mechanisms for Welfare Maximization in Rich Advertising Auctions
Divyarthi Mohan
Alex Psomas
Advances in Neural Information Processing Systems 35 (NeurIPS 2022) Main Conference Track
Preview abstract
Internet ad auctions have evolved from a few lines of text to richer informational layouts that include images, sitelinks, videos, etc. Ads in these new formats occupy varying amounts of space, and an advertiser can provide multiple formats, only one of which can be shown.The seller is now faced with a multi-parameter mechanism design problem.Computing an efficient allocation is computationally intractable, and therefore the standard Vickrey-Clarke-Groves (VCG) auction, while truthful and welfare-optimal, is impractical. In this paper, we tackle a fundamental problem in the design of modern ad auctions. We adopt a
Myersonian'' approach and study allocation rules that are monotone both in the bid and set of rich ads. We show that such rules can be paired with a payment function to give a truthful auction. Our main technical challenge is designing a monotone rule that yields a good approximation to the optimal welfare. Monotonicity doesn't hold for standard algorithms, e.g. the incremental bang-per-buck order, that give good approximations to
knapsack-like'' problems such as ours. In fact, we show that no deterministic monotone rule can approximate the optimal welfare within a factor better than 2 (while there is a non-monotone FPTAS). Our main result is a new, simple, greedy and monotone allocation rule that guarantees a 3-approximation. In ad auctions in practice, monotone allocation rules are often paired with the so-called \emph{Generalized Second Price (GSP)} payment rule, which charges the minimum threshold price below which the allocation changes. We prove that, even though our monotone allocation rule paired with GSP is not truthful, its Price of Anarchy (PoA) is bounded. Under standard no-overbidding assumptions, we prove bounds on the a pure and Bayes-Nash PoA. Finally, we experimentally test our algorithms on real-world data.
View details
Preview abstract
We study the joint optimization problem of pricing trips in a transportation network and serving the induced demands by routing a fleet of available service vehicles to maximize revenue. Our framework encompasses applications that include traditional transportation networks (e.g., airplanes, buses) and their more modern counterparts (e.g., ride-sharing systems). We describe a simple combinatorial model, in which each edge in the network is endowed with a curve that gives the demand for traveling between its endpoints at any given price. We are supplied with a number of vehicles and a time budget to serve the demands induced by the prices that we set, seeking to maximize revenue. We first focus on a (preliminary) special case of our model with unit distances and unit time horizon. We show that this version of the problem can be solved optimally in polynomial time. Switching to the general case of our model, we first present a two-stage approach that separately optimizes for prices and routes, achieving a logarithmic approximation to revenue in the process. Next, using the insights gathered in the first two results, we present a constant factor approximation algorithm that jointly optimizes for prices and routes for the supply vehicles. Finally, we discuss how our algorithms can handle capacitated vehicles, impatient demands, and selfish (wage-maximizing) drivers.
View details
Preview abstract
Modern ad auctions allow advertisers to target more specific segments of the user population. Unfortunately,
this is not always in the best interest of the ad platform – partially hiding some information
could be more beneficial for the platform’s revenue. In this paper, we examine the following basic question
in the context of second-price ad auctions: how should an ad platform optimally reveal information
about the ad opportunity to the advertisers in order to maximize revenue? We consider a model in which
bidders’ valuations depend on a random state of the ad opportunity. Different from previous work, we
focus on a more practical, and challenging, situation where the space of possible realizations of ad opportunities
is extremely large. We thus focus on developing algorithms whose running time is polynomial
in the number of bidders, but is independent of the number of ad opportunity realizations.
We assume that the auctioneer can commit to a signaling scheme to reveal noisy information about
the realized state of the ad opportunity, and examine the auctioneer’s algorithmic question of designing
the optimal signaling scheme. We first consider that the auctioneer is restricted to send a public
signal to all bidders. As a warm-up, we start with a basic (though less realistic) setting in which the
auctioneer knows the bidders’ valuations, and show that an -optimal scheme can be implemented in
time polynomial in the number of bidders and 1/. We then move to a well-motivated Bayesian valuation
setting in which the auctioneer and bidders both have private information, and present two results.
First, we exhibit a characterization result regarding approximately optimal schemes and prove that any
constant-approximate public signaling scheme must use exponentially many signals. Second, we present
a “simple” public signaling scheme that serves as a constant approximation under mild assumptions.
Finally, we initiate an exploration on the power of being able to send different signals privately to
different bidders. In the basic setting where the auctioneer knows bidders’ valuations, we exhibit a
polynomial-time private scheme that extracts almost full surplus even in the worst Bayes Nash equilibrium.
This illustrates the surprising power of private signaling schemes in extracting revenue.
View details
Concise Bid Optimization Strategies with Multiple Budget Constraints
Arash Asadpour
WINE, The 10th Conference on Web and Internet Economics(2014)
Preview abstract
A major challenge faced by the marketers attempting to optimize their advertising campaigns is to deal with budget constraints. The problem is even harder in the face of multidimensional budget constraints, particularly in the presence of many decision variables involved, and the interplay among the decision variables through these such constraints. Concise bidding strategies help advertisers deal with this challenge by introducing fewer variables to act on.
In this paper, we study the problem of finding optimal concise bidding strategies for advertising campaigns with multiple budget constraints. Given bid landscapes—i.e., predicted value (e.g., number of clicks) and cost per click for any bid—that are typically provided by ad-serving systems, we optimize the value given budget constraints. In particular, we consider bidding strategies that consist of no more than k different bids for all keywords. For constant k, we provide a PTAS to optimize the profit, whereas for arbitrary k we show how constant-factor approximation can be obtained via a combination of solution enumeration and dependent LP-rounding techniques.
Finally, we evaluate the performance of our algorithms on real datasets in two regimes with 1- and 3-dimensional budget constraint. In the former case where uniform bidding has provable performance guarantee, our algorithm beats the state of the art by an increase of 1% to 6% in the expected number of clicks. This is achieved by only two or three clusters—contrast with the single cluster permitted in uniform bidding. With only three dimensions in the budget constraint (one for total consumption, and another two for enforcing minimal diversity), the gap between the performance of our algorithm and an enhanced version of uniform bidding grows to an average of 5% to 6% (sometimes as high as 9%). Although the details of experiments for the multidimensional budget constraint to the full version of the paper are deferred to the full version of the paper, we report some highlights from the results.
View details
Value of Targeting
Patrick Hummel
Proceedings of the 7th International Symposium on Algorithmic Game Theory (SAGT)(2014), pp. 194-205
Preview abstract
We undertake a formal study of the value of targeting data to an advertiser. As expected, this value is increasing in the utility difference between realizations of the targeting data and the accuracy of the data, and depends on the distribution of competing bids. However, this value may vary non-monotonically with an advertiser’s budget. Similarly, modeling the values as either private or correlated, or allowing other advertisers to also make use of the data, leads to unpredictable changes in the value of data. We address questions related to multiple data sources, show that utility of additional data may be non-monotonic, and provide tradeoffs between the quality and the price of data sources. In a game-theoretic setting, we show that advertisers may be worse off than if the data had not been available at all. We also ask whether a publisher can infer the value an advertiser would place on targeting data from the advertiser’s bidding behavior and illustrate that this is impossible.
View details