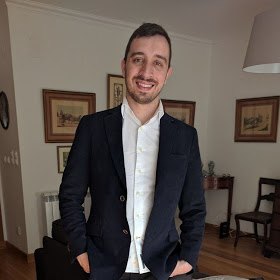
Gonçalo Simões
Authored Publications
Sort By
A Well-Composed Text is Half Done! Composition Sampling for Diverse Conditional Generation
Yao Zhao
Mirella Lapata
Proceedings of the 60th Annual Meeting of the Association for Computational Linguistics (ACL 2022), Association for Computational Linguistics, pp. 21
Preview abstract
We propose Composition Sampling, a simple but effective method to generate diverse outputs for conditional generation of higher quality compared to previous stochastic decoding strategies. It builds on recently proposed plan-based neural generation models (Narayan et al., 2021) that are trained to first create a composition of the output and then generate by conditioning on it and the input. Our approach
avoids text degeneration by first sampling a composition in the form of an entity chain and then using beam search to generate the best possible text grounded to this entity chain. Experiments on summarization (CNN/DailyMail and XSum) and question generation (SQuAD), using existing and newly proposed automatic metrics together with human-based evaluation, demonstrate that Composition Sampling is currently the best available decoding strategy for generating diverse meaningful outputs.
View details
Planning with Learned Entity Prompts for Abstractive Summarization
Yao Zhao
Ryan McDonald
Transactions of the Association for Computational Linguistics, 9(2021), 1475–1492
Preview abstract
We investigate Entity Chain -- a chain of related entities in the summary -- as an intermediate summary representation to better plan and ground the generation of abstractive summaries. In particular, we achieve this by augmenting the target by appending it with an entity chain extracted from the target. We experiment with Transformer-based encoder-decoder models; a transformer encoder first encodes the input and a transformer decoder generates an intermediate summary representation in the form of an entity chain and then continues generating the summary conditioned on the entity chain and the input. We evaluate our approach on a diverse set of text summarization tasks and show that Pegasus finetuned models with entity chains clearly outperform regular finetuning in terms of entity accuracy. We further demonstrate that our simple method can be easily used for pretraining summarization models to do entity-level content planning and summary generation. We see further gains with pretraining.
View details
Preview abstract
Document and discourse segmentation are two fundamental NLP tasks pertaining to breaking up text into constituents, which are commonly used to help downstream tasks such as information retrieval or text summarization. In this work, we propose three transformer-based architectures and provide comprehensive comparisons with previously proposed approaches on three standard datasets. We establish a new state-of-the-art, reducing in particular the error rates by a large margin in all cases. We further analyze model sizes and find that we can build models with many fewer parameters while keeping good performance, thus facilitating real-world applications.
View details
Morphosyntactic Tagging with a Meta-BiLSTM Model over Context Sensitive Token Encodings
Ryan Mcdonald
Emily Pitler
Association for Computational Linguistics (ACL), Melbourne, Australia(2018)
Preview abstract
The rise of neural networks, and particularly recurrent neural networks, has produced significant advances in part-of-speech tagging accuracy. One characteristic common among these models is the presence of rich initial word encodings. These encodings typically are composed of a recurrent character-based representation with learned and pre-trained word embeddings. However, these encodings do not consider a context wider than a single word and it is only through subsequent recurrent layers that word or sub-word information interacts. In this paper, we investigate models that use recurrent neural networks with sentence-level context for initial character and word-based representations. In particular we show that optimal results are obtained by integrating these context sensitive representations through synchronized training with a meta-model that learns to combine their states. We present results on part-of-speech and morphological tagging with state-of-the-art performance on a number of languages.
View details