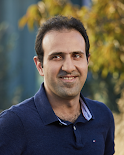
Ahmad Beirami
Ahmad Beirami is a research scientist at Google Research, leading a research team on building safe, helpful, and scalable generative language models. At Meta AI, he led research to power the next generation of virtual digital assistants with AR/VR capabilities through robust generative language modeling. At Electronic Arts, he led the AI agent research program for automated playtesting of video games and cooperative reinforcement learning. Before moving to industry in 2018, he held a joint postdoctoral fellow position at Harvard & MIT, focused on problems in the intersection of core machine learning and information theory. He is the recipient of the 2015 Sigma Xi Best PhD Thesis Award from Georgia Tech.
See his personal homepage for more information and news.
See his personal homepage for more information and news.
Authored Publications
Google Publications
Other Publications
Sort By
Preview abstract
Language models still struggle on moral reasoning, despite their impressive performance in many other tasks. In particular, the Moral Scenarios task in MMLU (Multi-task Language Understanding) is among the worst performing tasks for many language models, including GPT-3. In this work, we propose a new prompting framework, Thought Experiments, to teach language models to do better moral reasoning using counterfactuals. Experiment results show that our framework elicits counterfactual questions and answers from the model, which in turn helps improve the accuracy on Moral Scenarios task by 9-16% compared to other zero-shot baselines. Interestingly, unlike math reasoning tasks, zero-shot Chain-of-Thought (CoT) reasoning doesn't work out of the box, and even reduces accuracy by around 4% compared to direct zero-shot. We further observed that with minimal human supervision in the form of 5 few-shot examples, the accuracy of the task can be improved to as much as 80%.
View details
Towards A Scalable Solution for Improving Multi-Group Fairness in Compositional Classification
Tina Tian
Ben Packer
Meghana Deodhar
Alex Beutel
The Second Workshop on Spurious Correlations, Invariance and Stability @ ICML 2023 (2023)
Preview abstract
Despite the rich literature on machine learning fairness, relatively little attention has been paid to remediating complex systems, where the final prediction is the combination of multiple classifiers and where multiple groups are present. In this paper, we first show that natural baseline approaches for improving equal opportunity fairness scale linearly with the product of the number of remediated groups and the number of remediated prediction labels, rendering them impractical. We then introduce two simple techniques, called task-overconditioning and group-interleaving, to achieve a constant scaling in this multi-group multi-label setup. Our experimental results in academic and real-world environments demonstrate the effectiveness of our proposal at mitigation within this environment.
View details
Preview abstract
Video self-supervised learning (VSSL) has made significant progress in recent years. However, the exact behavior and dynamics of these models under different forms of distribution shift are not yet known. In this paper, we comprehensively study the behavior of six popular self-supervised methods (v-SimCLR, v-MoCo, v-BYOL, v-SimSiam, v-DINO, v-MAE) in response to various forms of natural distribution shift, i.e., (i) context shift, (ii) viewpoint shift, (iii) actor shift, (iv) source shift, (v) generalizability to unknown classes (zero-shot), and (vi) open-set recognition. To perform this extensive study, we carefully craft a test bed consisting of 17 in-distribution and out-of-distribution benchmark pairs using available public datasets and a series of evaluation protocols to stress-test the different methods under the intended shifts. Our study uncovers a series of intriguing findings and interesting behaviors of VSSL methods. For instance, we observe that while video models generally struggle with context shifts, v-MAE and supervised learning exhibit more robustness. Moreover, our study shows that v-MAE is a strong temporal learner, whereas contrastive methods, v-SimCLR and v-MoCo, exhibit strong performances against viewpoint shifts. When studying the notion of open-set recognition, we notice a trade-off between closed-set and open-set recognition performance if the pretrained VSSL encoders are used without finetuning. We hope that our work will contribute to the development of robust video representation learning frameworks for various real-world scenarios.
View details
No Results Found