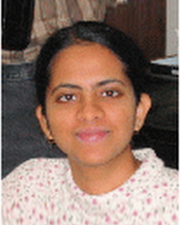
Vidhya Navalpakkam
I am currently a Principal Scientist at Google Research. I lead an interdisciplinary team at the intersection of Machine learning, Neuroscience, Cognitive Psychology and Vision. My interests are in modeling user attention and behavior across multimodal interfaces, for improved usability and accessibility of Google products. I am also interested in applications of attention for healthcare (e.g., smartphone-based screening for health conditions).
Authored Publications
Google Publications
Other Publications
Sort By
Rich Human Feedback for Text to Image Generation
Katherine Collins
Nicholas Carolan
Yang Li
Youwei Liang
Peizhao Li
Dj Dvijotham
Junfeng He
Sarah Young
Jiao Sun
Arseniy Klimovskiy
Preview abstract
Recent Text-to-Image (T2I) generation models such as Stable Diffusion and Imagen have made significant progress in generating high-resolution images based on text descriptions. However, many generated images still suffer from issues such as artifacts/implausibility, misalignment with text descriptions, and low aesthetic quality.
Inspired by the success of Reinforcement Learning with Human Feedback (RLHF) for large language models, prior work collected human-provided scores as feedback on generated images and trained a reward model to improve the T2I generation.
In this paper, we enrich the feedback signal by (i) marking image regions that are implausible or misaligned with the text, and (ii) annotating which keywords in the text prompt are not represented in the image.
We collect such rich human feedback on 18K generated images and train a multimodal transformer to predict these rich feedback automatically.
We show that the predicted rich human feedback can be leveraged to improve image generation, for example, by selecting high-quality training data to finetune and improve the generative models, or by creating masks with predicted heatmaps to inpaint the problematic regions.
Notably, the improvements generalize to models (Muse) beyond those used to generate the images on which human feedback data were collected (Stable Diffusion variants).
View details
Digital biomarker of mental fatigue
Vincent Wen-Sheng Tseng
Venky Ramachandran
Tanzeem Choudhury
npj Digital Medicine, 4(2021), pp. 1-5
Preview abstract
Mental fatigue is an important aspect of alertness and wellbeing. Existing fatigue tests are subjective and/or time-consuming. Here, we show that smartphone-based gaze is significantly impaired with mental fatigue, and tracks the onset and progression of fatigue. A simple model predicts mental fatigue reliably using just a few minutes of gaze data. These results suggest that smartphone-based gaze could provide a scalable, digital biomarker of mental fatigue.
View details
Accelerating eye movement research via accurate and affordable smartphone eye tracking
Na Dai
Ethan Steinberg
Junfeng He
Kantwon Rogers
Venky Ramachandran
Mina Shojaeizadeh
Li Guo
Nature Communications, 11(2020)
Preview abstract
Eye tracking has been widely used for decades in vision research, language and usability. However, most prior research has focused on large desktop displays using specialized eye trackers that are expensive and cannot scale. Little is known about eye movement behavior on phones, despite their pervasiveness and large amount of time spent. We leverage machine learning to demonstrate accurate smartphone-based eye tracking without any additional hardware. We show that the accuracy of our method is comparable to state-of-the-art mobile eye trackers that are 100x more expensive. Using data from over 100 opted-in users, we replicate key findings from previous eye movement research on oculomotor tasks and saliency analyses during natural image viewing. In addition, we demonstrate the utility of smartphone-based gaze for detecting reading comprehension difficulty. Our results show the potential for scaling eye movement research by orders-of-magnitude to thousands of participants (with explicit consent), enabling advances in vision research, accessibility and healthcare.
View details
On-device Few-shot Personalization for Real-time Gaze Estimation
Junfeng He
Khoi Pham
Chase Riley Roberts
Dmitry Lagun
ICCV 2019 Gaze workshop
Preview abstract
Recent research has demonstrated the ability to estimate user’s gaze on mobile devices, by performing inference from an image captured with the phone’s front-facing camera, and without requiring specialized hardware. Gaze estimation accuracy is known to improve with additional calibration data from the user. However, most existing methods require either significant number of calibration
points or computationally intensive model fine-tuning that is practically infeasible on a mobile device. In this paper, we overcome limitations of prior work by proposing a novel few-shot personalization approach for 2D gaze estimation. Compared to the best calibration-free model [11], the proposed method yields substantial improvements in gaze prediction accuracy (24%) using only 3 calibration
points in contrast to previous personalized models that offer less improvement while requiring more calibration points. The proposed model requires 20x fewer FLOPS than the state-of-the-art personalized model [11] and can be run entirely on-device and in real-time, thereby unlocking a variety of important applications like accessibility, gaming and human-computer interaction.
View details
Towards better measurement of attention and satisfaction in mobile search
Dmitry Lagun
Chih-Hung Hsieh
SIGIR '14 Proceedings of the 37th international ACM SIGIR conference on Research & development in information retrieval(2014), pp. 113-122
Preview abstract
Web Search has seen two big changes recently: rapid growth in mobile search traffic, and an increasing trend towards providing answer-like results for relatively simple information needs (e.g., [weather today]). Such results display the answer or relevant information on the search page itself without requiring a user to click. While clicks on organic search results have been used extensively to infer result relevance and search satisfaction, clicks on answer-like results are often rare (or meaningless), making it challenging to evaluate answer quality. Together, these call for better measurement and understanding of search satisfaction on mobile devices. In this paper, we studied whether tracking the browser viewport (visible portion of a web page) on mobile phones could enable accurate measurement of user attention at scale, and provide good measurement of search satisfaction in the absence of clicks. Focusing on answer-like results in web search, we designed a lab study to systematically vary answer presence and relevance (to the user's information need), obtained satisfaction ratings from users, and simultaneously recorded eye gaze and viewport data as users performed search tasks. Using this ground truth, we identified increased scrolling past answer and increased time below answer as clear, measurable signals of user dissatisfaction with answers. While the viewport may contain three to four results at any given time, we found strong correlations between gaze duration and viewport duration on a per result basis, and that the average user attention is focused on the top half of the phone screen, suggesting that we may be able to scalably and reliably identify which specific result the user is looking at, from viewport data alone.
View details
Measurement and modeling of eye-mouse behavior
Preview
LaDawn Jentzsch
Rory Sayres
Sujith Ravi
Amr Ahmed
Alex J. Smola
Proceedings of the 22nd International World Wide Web Conference(2013)
Mouse tracking: measuring and predicting users' experience of web-based content
On saliency, affect and focused attention
Attention and Selection in Online Choice Tasks
Multimedia features for click prediction of new ads in display advertising
Haibin Cheng
Roelof van Zwol
Javad Azimi
Eren Manavoglu
Ruofei Zhang
Yang Zhou
KDD(2012), pp. 777-785
Behavior and neural basis of near-optimal visual search
Wei Ji Ma
Jeff Beck
Ronald van den Berg
Alex Pouget
Nature Neuroscience, 14(2011), pp. 783-790
Preview abstract
The ability to search efficiently for a target in a cluttered environment is one of the most remarkable functions of the nervous system. This task is difficult under natural circumstances, as the reliability of sensory information can vary greatly across space and time and is typically a priori unknown to the observer. In contrast, visual-search experiments commonly use stimuli of equal and known reliability. In a target detection task, we randomly assigned high or low reliability to each item on a trial-by-trial basis. An optimal observer would weight the observations by their trial-to-trial reliability and combine them using a specific nonlinear integration rule. We found that humans were near-optimal, regardless of whether distractors were homogeneous or heterogeneous and whether reliability was manipulated through contrast or shape. We present a neural-network implementation of near-optimal visual search based on probabilistic population coding. The network matched human performance.
View details
Predicting response time and error rates in visual search
Using gaze patterns to study and predict reading struggles due to distraction
Optimal reward harvesting in complex perceptual environments
Christof Koch
Antonio Rangel
Pietro Perona
Proceedings of National Academy of Sciences (PNAS), 107(2010), 5232–5237
Preview abstract
The ability to choose rapidly among multiple targets embedded in a complex perceptual environment is key to survival. Targets may differ in their reward value as well as in their low-level perceptual properties (e.g., visual saliency). Previous studies investigated separately the impact of either value or saliency on choice; thus, it is not known how the brain combines these two variables during decision making. We addressed this question with three experiments in which human subjects attempted to maximize their monetary earnings by rapidly choosing items from a brief display. Each display contained several worthless items (distractors) as well as two targets, whose value and saliency were varied systematically. We compared the behavioral data with the predictions of three computational models assuming that (i) subjects seek the most valuable item in the display, (ii) subjects seek the most easily detectable item, and (iii) subjects behave as an ideal Bayesian observer who combines both factors to maximize the expected reward within each trial. Regardless of the type of motor response used to express the choices, we find that decisions are influenced by both value and feature-contrast in a way that is consistent with the ideal Bayesian observer, even when the targets’ feature-contrast is varied unpredictably between trials. This suggests that individuals are able to harvest rewards optimally and dynamically under time pressure while seeking multiple targets embedded in perceptual clutter.
View details
Homo economicus in visual search
Preview abstract
How do reward outcomes affect early visual performance? Previous studies found a suboptimal influence, but they ignored the non-linearity in how subjects perceived the reward outcomes. In contrast, we find that when the non-linearity is accounted for, humans behave optimally and maximize expected reward. Our subjects were asked to detect the presence of a familiar target object in a cluttered scene. They were rewarded according to their performance. We systematically varied the target frequency and the reward/penalty policy for detecting/missing the targets. We find that 1) decreasing the target frequency will decrease the detection rates, in accordance with the literature. 2) Contrary to previous studies, increasing the target detection rewards will compensate for target rarity and restore detection performance. 3) A quantitative model based on reward maximization accurately predicts human detection behavior in all target frequency and reward conditions; thus, reward schemes can be designed to obtain desired detection rates for rare targets. 4) Subjects quickly learn the optimal decision strategy; we propose a neurally plausible model that exhibits the same properties. Potential applications include designing reward schemes to improve detection of life-critical, rare targets (e.g., cancers in medical images).
View details
Search goal tunes visual features optimally
Preview abstract
How does a visual search goal modulate the activity of neurons encoding different visual features (e.g., color, direction of motion)? Previous research suggests that goal-driven attention enhances the gain of neurons representing the target's visual features. Here, we present mathematical and behavioral evidence that this strategy is suboptimal and that humans do not deploy it. We formally derive the optimal feature gain modulation theory, which combines information from both the target and distracting clutter to maximize the relative salience of the target. We qualitatively validate the theory against existing electrophysiological and psychophysical literature. A surprising prediction is that it is sometimes optimal to enhance nontarget features. We provide experimental evidence toward this through psychophysics experiments on human subjects, thus suggesting that humans deploy the optimal gain modulation strategy.
View details
An Integrated Model of Top-Down and Bottom-Up Attention for Optimizing Detection Speed
Optimal cue selection strategy
A Goal Oriented Attention Guidance Model