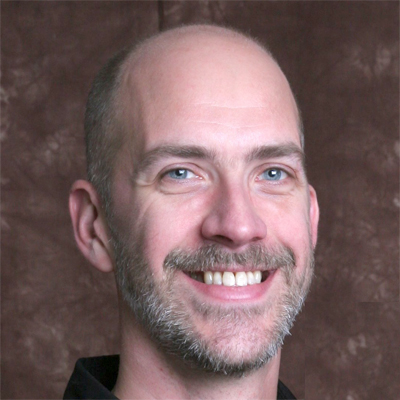
Tom Kenter
Tom Kenter received his PhD in 2017 from the Information and Language Processing Systems group at the University of Amsterdam, supervised by prof. dr. Maarten de Rijke. He is currently doing research at Google UK, on the topic of text-to-speech and natural language understanding.
He has published at ACL, CIKM, SIGIR and AAAI.
Research Areas
Authored Publications
Sort By
Preview abstract
Inter-sentence pauses are the silences that occur between sentences in a paragraph or a dialogue.
They are an important aspect of long-form speech prosody, as they can affect the naturalness, intelligibility, and effectiveness of communication.
However, the user perception of inter-sentence pauses in long-form speech synthesis is not well understood. Previous work often evaluates pause modelling in conjunction with other prosodic features making it hard to explicitly study how raters perceive differences in inter-sentence pause lengths.
In this paper, using multiple text-to-speech (TTS) datasets that cover different content types, domains, and settings, we investigate how sensitive raters are to changes to the durations of inter-sentence pauses in long-form speech by comparing ground truth audio samples with renditions that have manipulated pause durations.
This experimental design is meant to allow us to draw conclusions regarding the utility that can be expected from similar evaluations when applied to synthesized long-form speech.
We find that, using standard evaluation methodologies, raters are not sensitive to variations in pause lengths unless these deviate exceedingly from the norms or expectations of the speech context.
View details
Preview abstract
Inter-sentence pauses are the silences that occur between sentences in a paragraph or a dialogue.
They are an important aspect of long-form speech prosody, as they can affect the naturalness, intelligibility, and effectiveness of communication.
However, the user perception of inter-sentence pauses in long-form speech synthesis is not well understood. Previous work often evaluates pause modelling in conjunction with other prosodic features making it hard to explicitly study how raters perceive differences in inter-sentence pause lengths.
In this paper, using multiple text-to-speech (TTS) datasets that cover different content types, domains, and settings, we investigate how sensitive raters are to changes to the durations of inter-sentence pauses in long-form speech by comparing ground truth audio samples with renditions that have manipulated pause durations.
This experimental design is meant to allow us to draw conclusions regarding the utility that can be expected from similar evaluations when applied to synthesized long-form speech.
We find that, using standard evaluation methodologies, raters are not sensitive to variations in pause lengths unless these deviate exceedingly from the norms or expectations of the speech context.
View details
Preview abstract
The quality of synthetic speech is typically evaluated using subjective listening tests. An underlying assumption is that these tests are reliable, i.e., running the test multiple times gives consistent results. A common approach to study reliability is a replication study. Existing studies focus primarily on Mean Opinion Score (MOS), and few consider the error bounds from the original test. In contrast, we present a replication study of both MOS and AB preference tests to answer two questions: (1) which of the two test types is more reliable for system comparison, and (2) for both test types, how reliable are the results with respect to their estimated standard error? We find that while AB tests are more reliable for system comparison, standard errors are underestimated for both test types. We show that these underestimates are partially due to broken independence assumptions, and suggest alternate methods of standard error estimation that account for dependencies among ratings.
View details
Training Text-To-Speech Systems From Synthetic Data: A Practical Approach For Accent Transfer Tasks
Lev Finkelstein
Norman Casagrande
Ye Jia
Alexey Petelin
Jonathan Shen
Yu Zhang
Interspeech(2022)
Preview abstract
Transfer tasks in text-to-speech (TTS) synthesis — where one
or more aspects of the speech of one set of speakers is transferred
to another set of speakers that do not feature these aspects originally —
remains a challenging task. One of the challenges is that models
that have high-quality transfer capabilities can have issues in stability,
making them impractical for user-facing critical tasks. This paper
demonstrates that transfer can be obtained by training an robust TTS
system on data generated by a less robust TTS system designed for a high-quality
transfer task; In particular, a CHiVE-BERT monolingual TTS
system is trained on the output of a Tacotron model designed
for accent transfer. While some quality loss is inevitable with
this approach, experimental results show that the models trained
on synthetic data this way can produce high quality audio displaying accent
transfer, while preserving speaker characteristics such as speaking style.
View details
Frugal Paradigm Completion
Alex Erdmann
Christian Schallhart
Proceedings of the 58th Annual Meeting of the Association for Computational Linguistics(2020), pp. 8248-8273
Preview abstract
Lexica distinguishing all morphologically related forms of each lexeme are crucial to many language technologies, yet building them is expensive. We propose Frugal Paradigm Completion, an approach that predicts all related forms in a morphological paradigm from as few manually provided forms as possible. It induces typological information during training which it uses to determine the best sources at test time. We evaluate our language-agnostic approach on 7 diverse languages. Compared to popular alternative approaches, our Frugal Paradigm Completion approach reduces manual labor by 16-63% and
is the most robust to typological variation.
View details
Preview abstract
The prosody of currently available speech synthesis systems can be unnatural due to the systems only having access to the text, possibly enriched by linguistic information such as part-of-speech tags and parse trees. We show that incorporating a BERT model in an RNN-based speech synthesis model - where the BERT model is pretrained on large amounts of unlabeled data, and fine-tuned to the speech domain - improves prosody. Additionally, we propose a way of handling arbitrarily long sequences with BERT. Our findings indicate that small BERT models work better than big ones, and that fine-tuning
the BERT part of the model is pivotal for getting good results.
View details
Preview abstract
Recently, WaveNet has become a popular choice of neural network to synthesize speech audio. Autoregressive WaveNet is capable of producing high-fidelity audio, but is too slow for real-time synthesis. As a remedy, Parallel WaveNet was proposed, which can produce audio faster than real time through distillation of an autoregressive teacher into a feedforward student network. A shortcoming of this approach, however, is that a large amount of recorded speech data is required to produce high-quality student models, and this data is not always available. In this paper, we propose StrawNet: a self-training approach to train a Parallel WaveNet. Self-training is performed using the synthetic examples generated by the autoregressive WaveNet teacher. We show that, in low-data regimes, training on high-fidelity synthetic data from an autoregressive teacher model is superior to training the student model on (much fewer) examples of recorded speech. We compare StrawNet to a baseline Parallel WaveNet, using both side-by-side tests and Mean Opinion Score evaluations. To our knowledge, synthetic speech has not been used to train neural text-to-speech before.
View details
Preview abstract
Text-to-speech systems are typically evaluated on single sentences. When long-form content, such as data consisting of full paragraphs or dialogues is considered, evaluating sentences in isolation is not always appropriate as the context in which the sentences are synthesized is missing.
In this paper, we investigate three different ways of evaluating the naturalness of long-form text-to-speech synthesis. We compare the results obtained from evaluating sentences in isolation, evaluating whole paragraphs of speech, and presenting a selection of speech or text as context and evaluating the subsequent speech. We find that, even though these three evaluations are based upon the same material, the outcomes differ per setting, and moreover that these outcomes do not necessarily correlate with each other. We show that our findings are consistent between a single speaker setting of read paragraphs and a two-speaker dialogue scenario. We conclude that to evaluate the quality of long-form speech, the traditional way of evaluating sentences in isolation does not suffice, and that multiple evaluations are required.
View details
Personal Knowledge Graphs: A Research Agenda
Proceedings of the ACM SIGIR International Conference on the Theory of Information Retrieval (ICTIR), ACM(2019)
Preview abstract
Knowledge graphs, organizing structured information about entities, and their attributes and relationships, are ubiquitous today. Entities, in this context, are usually taken to be anyone or anything considered to be globally important. This, however, rules out many entities people interact with on a daily basis. In this position paper, we present the concept of personal knowledge graphs: resources of structured information about entities personally related to its user, including the ones that might not be globally important. We discuss key aspects that separate them for general knowledge graphs, identify the main challenges involved in constructing and
using them, and define a research agenda.
View details
CHiVE: Varying Prosody in Speech Synthesis with a Linguistically Driven Dynamic Hierarchical Conditional Variational Network
Jakub Vit
Proceedings of the 36th International Conference on Machine Learning (ICML 2019), PMLR, pp. 3331-3340
Preview abstract
The prosodic aspects of speech signals produced by current text-to-speech systems are typically averaged over training material, and as such lack the variety and liveliness found in natural speech. To avoid monotony and averaged prosody contours, it is desirable to have a way of modeling the variation in the prosodic aspects of speech, so audio signals can be synthesized in multiple ways for a given text. We present a new, hierarchically structured conditional variational autoencoder to generate prosodic features (fundamental frequency, energy and duration) suitable for use with a vocoder or a generative model like WaveNet. At inference time, an embedding representing the prosody of a sentence may be sampled from the variational layer to allow for prosodic variation. To efficiently capture the hierarchical nature of the linguistic input (words, syllables and phones), both the encoder and decoder parts of the auto-encoder are hierarchical, in line with the linguistic structure, with layers being clocked dynamically at the respective rates. We show in our experiments that our dynamic hierarchical network outperforms a non-hierarchical state-of-the-art baseline, and, additionally, that prosody transfer across sentences is possible by employing the prosody embedding of one sentence to generate the speech signal of another.
View details