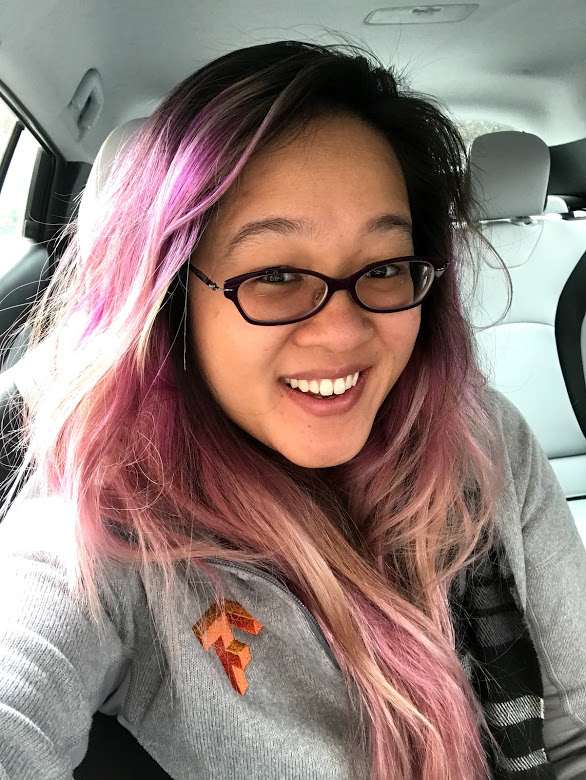
Sherol Chen
Sherol Chen has studied AI for over a decade. Currently, she is part of Google Research working on building and understanding large Machine Learning models. At Google, Sherol advocated for Cloud enterprises, worked at Google Brain for Machine Learning in Music and Creativity for project Magenta, and built algorithmic search results for YouTube. She's taught Artificial Intelligence for Stanford University Pre-Collegiate and around the world in Kazakhstan, China, India, Chile, and Peru. Her PhD work is in Computer Science, researching storytelling and Artificial Intelligence at the Expressive Intelligence Studio.
Authored Publications
Sort By
Preview abstract
What are dimensions of human intent, and how do writing tools shape and augment these expressions? From papyrus to auto-complete, a major turning point was when Alan Turing famously asked, “Can Machines Think?” If so, should we offload aspects of our thinking to machines, and what impact do they have in enabling the intentions we have? This paper adapts the Authorial Leverage framework, from the Intelligent Narrative Technologies literature, for evaluating recent generative model advancements. With increased widespread access to Large Language Models (LLMs), the evolution of our evaluative frameworks follow suit. To do this, we discuss previous expert studies of deep generative models for fiction writers and playwrights, and propose two future directions, (1) author-focused and (2) audience-focused, for furthering our understanding of Authorial Leverage of LLMs, particularly in the domain of comedy writing.
View details
Preview abstract
This paper aims to survey the problem space around cultural barriers in research collaboration, specifically for Machine Learning (ML). We review (1) unequal representation in ML/AI and STEM, (2) socioeconomic influences on retention of scientists and researchers, and (3) existing educational opportunity programs for people from underresourced backgrounds, with emphasis on Post-Baccalaureate support. We provide evidence that scientists from disadvantaged backgrounds not only experience barriers to gaining intellectual and technical expertise, but also often experience cultural gaps that impede their inclusion in research collaborations. We discuss relevant research on culture differences and the ways that some U.S. Federal TRIO programs explicitly address them, highlighting standardization as one means of demystifying academic and research cultures. We conclude with recommendations toward understanding post-education culture gaps, with the goal of finding better solutions for increasing diversity in research collaborations.
View details
Story Centaur: Large Language Model Few Shot Learning as a Creative Writing Tool
Ben Pietrzak
Ben Swanson
Monica Dinculescu
EACL (European Association of Computational Linguistics)(2021)
Preview abstract
Few shot learning with large language models has the potential to give people without formal machine learning training the access to a wide range of text to text models. We consider how this applies to creative writers and present \textsc{Story Centaur}, a user interface for prototyping few shot models and a set of recombinable web components that deploy them. \textsc{Story Centaur}'s goal is to expose creative writers to few shot learning with a simple but powerful interface that lets these writers compose their own co-creation tools that further their own unique artistic directions. We build out several examples of this goal, and in the process probe the boundaries and issues surrounding generation with large language models.
View details
Preview abstract
A majority of games keep to discrete inputs and have not easily realized the expressivity of spoken language interfaces. Furthermore, natural language processing systems had limitations understanding language intent. For this paper, we define a type of language interface, Semantic Chat, and the challenges of achieving this functionality for interactive fiction and multiplayer games. In the past, games accepted text chat, through a keyboard, or voice chat, through a microphone; however, the inputs were often read verbatim and, at most, pattern matched to a desired intent. With recent advancements in deep learning, language models are able to more effectively derive the semantic meaning behind the textual input, and machine learning models have become increasingly better at transcribing voice. Even so, Semantic Chat is still rarely found in games. In practice, the application of these neural language models is an open problem, with non-trivial challenges in deployment. Using techniques like transfer learning, we discuss the obstacles in realizing believable voice avatars.
View details
Preview abstract
Our objective is to create an expressive language interface that allows human participants to have agency in narrative-driven virtual worlds. Text to Dialog (TTD) gives narrative designers an opportunity to paint audience participants into a story universe utilizing semantic similarity. To do this, we apply the Universal Sentence Encoder by using embedding vectors that specifically target transfer learning to story-dialog related NLP tasks. We conclude that building expressive tools like TTD could enable new artistic experiences through (1) Semantic Dialect Matching, where human-generated textual statements are semantically matched with a pre-scripted list of dialog (from an avatar's dialect, voice, or way of speaking), and (2) Semantic Dialog Selection, where natural language can maneuver decision points through semantic matching. We reference two case-studies to demonstrate each use-case.
View details
Identifying the intersections: User experience + research scientist collaboration in a generative machine learning interface
Jess Scon Holbrook
ACM CHI Conference 2019(2019)
Preview abstract
Creative generative machine learning interfaces are stronger when multiple actors bearing different points of view actively contribute to them. User experience (UX) research and design involvement in the creation of machine learning (ML) models help ML research scientists to more effectively identify human needs that ML models will fulfill. The People and AI Research (PAIR) group within Google developed a novel program method in which UXers are embedded into an ML research group for three months to provide a human-centered perspective on the creation of ML models. The first full-time cohort of UXers were embedded in a team of ML research scientists focused on deep generative models to assist in music composition. Here, we discuss the structure and goals of the program, challenges we faced during execution, and insights gained as a result of the process. We offer practical suggestions for how to foster communication between UX and ML research teams and recommended UX design processes for building creative generative machine learning interfaces.
View details
Preview abstract
We argue for the benefit of designing deep generative models through mixed-initiative combinations of deep learning algorithms and human specifications for authoring sequential content, such as stories and music.
Sequence models have shown increasingly convincing results in domains such as auto-completion, speech to text, and translation; however, longer-term structure remains a major challenge. Given lengthy inputs and outputs, deep generative systems still lack reliable representations of beginnings, middles, and ends, which are standard aspects of creating content in domains such as music composition. This paper aims to contribute a framework for mixed-initiative learning approaches, specifically for creative deep generative systems, and presents a case study of a deep generative model for music, Counterpoint by Convolutional Neural Network (Coconet).
View details