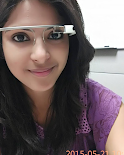
Shefali Garg
Shefali Garg received her Masters in Intelligent Information Systems from Language Technologies Institute at Carnegie Mellon University, USA in 2019 with research focus on NLP and Speech. She completed her Bachelors in Computer Science from Birla Institute of Technology and Science, India in 2016.
Following her Masters, Shefali joined the Speech Research Group at Google. At Google, her research has focused primarily on developing and improving end-to-end acoustic models, while keeping the principles of user privacy and DEI in mind.
Research Areas
Authored Publications
Google Publications
Other Publications
Sort By
Preview abstract
Personalization of speech models on mobile devices (on-device personalization) is an active area of research, but more often than not, mobile devices have more text-only data than paired audio-text data. We explore training a personalized language model on text-only data, used during inference to improve speech recognition performance for that user. We experiment on a user-clustered LibriSpeech corpus, supplemented with personalized text-only data for each user from Project Gutenberg. We release this User-Specific LibriSpeech (UserLibri) dataset to aid future personalization research. LibriSpeech audio-transcript pairs are grouped into 55 users from the test-clean dataset and 52 users from test-other. We are able to lower the average word error rate per user across both sets in streaming and nonstreaming models, including an improvement of 2.5 for the harder set of test-other users when streaming.
View details
Large-scale ASR Domain Adaptation by Self- and Semi-supervised Learning
David Qiu
Nikhil Siddhartha
ICASSP (2022) (to appear)
Preview abstract
Self- and Semi-supervised learning methods have been actively investigated to reduce labeled training data or enhance the model performance. However, the approach mostly focus on in-domain performance for public datasets. In this study, we utilize the combination of self- and semi-supervised learning methods to solve unseen domain adaptation problem in a large-scale production setting for online ASR model. This approach demonstrates that using the source domain data with a small fraction of the target domain data (3%) can recover the performance gap compared to a full data baseline: relative 13.5% WER improvement for target domain data.
View details
No Results Found