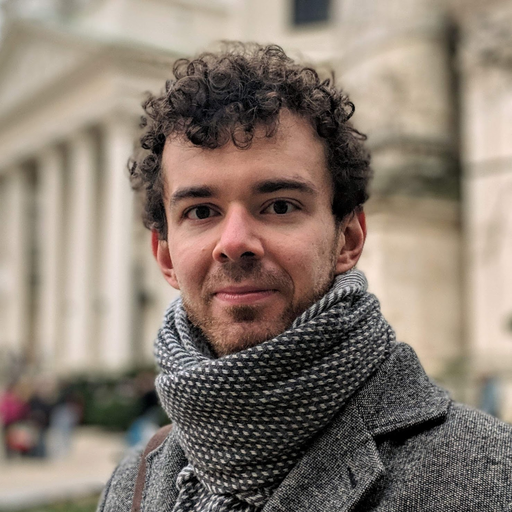
Sergii Kashubin
Research Areas
Authored Publications
Sort By
Preview abstract
Most variance reduction methods for stochastic optimization are primarily designed for smooth and strongly convex functions. They also often come with high memory requirements. Consequently, they do not scale to large scale deep learning settings where we are in presence of massive neural networks and virtually infinite data due to the use of data augmentation strategies. In this work, we extend convex online variance reduction to the realm of deep learning. We exploit the ubiquitous clustering structure of rich datasets used in deep learning to design a scalable variance reduced optimization procedure. Our proposal allows to leverage prior knowledge about a given problem to speedup the learning process. It is robust and theoretically well-motivated. Our experiments show that it is superior or on par with most widely used optimizers in deep learning on standard benchmark datasets.
View details
Continental-scale building detection from high resolution satellite imagery
Wojciech Sirko
Yasser Salah Eddine Bouchareb
Maxim Neumann
Moustapha Cisse
arXiv(2021)
Preview abstract
Identifying the locations and footprints of buildings is vital for many practical and scientific purposes, and such information can be particularly useful in developing regions where alternative data sources may be scarce. In this work, we describe a model training pipeline for detecting buildings across the entire continent of Africa, given 50cm satellite imagery. Starting with the U-Net model, widely used in satellite image analysis, we study variations in architecture, loss functions, regularization, pre-training, self-training and post-processing that increase instance segmentation performance. Experiments were carried out using a dataset of 100k satellite images across Africa containing 1.75M manually labelled building instances, and further datasets for pre-training and self-training. We report novel methods for improving performance of building detection with this type of model, including the use of mixup (mAP +0.12) and self-training with soft KL loss (mAP +0.06). The resulting pipeline obtains good results even on a wide variety of challenging rural and urban contexts, and was used to create the Open Buildings dataset of approximately 600M Africa-wide building footprints.
View details
Measuring Compositional Generalization: A Comprehensive Method on Realistic Data
Nathanael Schärli
Nathan Scales
Hylke Buisman
Daniel Furrer
Nikola Momchev
Danila Sinopalnikov
Lukasz Stafiniak
Tibor Tihon
Dmitry Tsarkov
ICLR(2020)
Preview abstract
State-of-the-art machine learning methods exhibit limited compositional generalization. At the same time, there is a lack of realistic benchmarks that comprehensively measure this ability, which makes it challenging to find and evaluate improvements. We introduce a novel method to systematically construct such benchmarks by maximizing compound divergence while guaranteeing a small atom divergence between train and test sets, and we quantitatively compare this method to other approaches for creating compositional generalization benchmarks. We present a large and realistic natural language question answering dataset that is constructed according to this method, and we use it to analyze the compositional generalization ability of three machine learning architectures. We find that they fail to generalize compositionally and that there is a surprisingly strong negative correlation between compound divergence and accuracy. We also demonstrate how our method can be used to create new compositionality benchmarks on top of the existing SCAN dataset, which confirms these findings.
View details