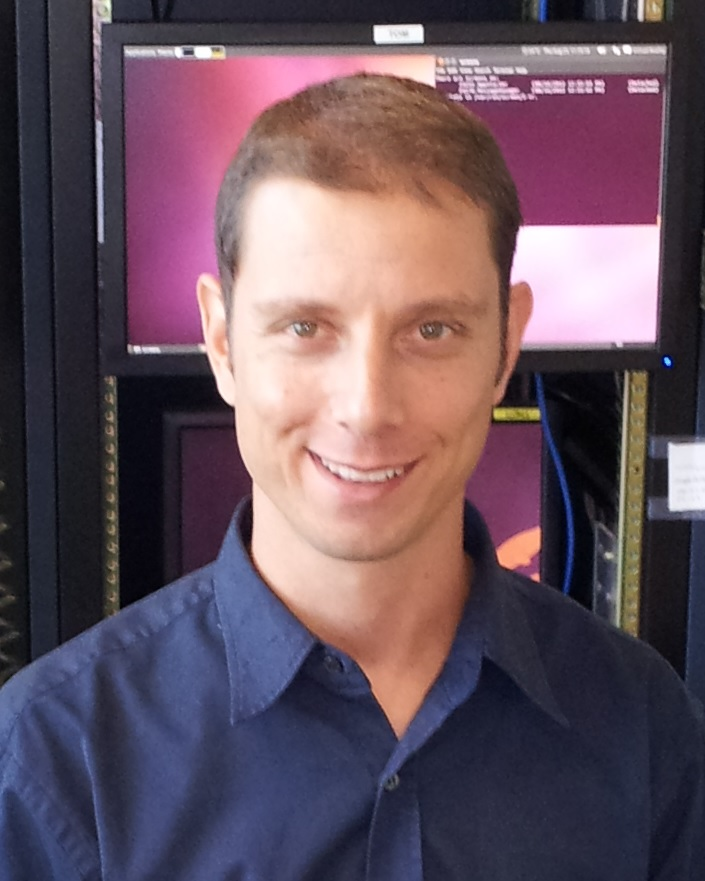
Sagi Perel
I joined Google in 2015 to work on unsupervised anomaly detection; and currently work on Machine Learning, with a focus on black box optimization.
My work includes a mixture of research and applied engineering.
I completed my PhD in Neural Engineering (Machine Learning & Neuroscience) at the University of Pittsburgh and the Center for Neural Basis of Cognition in 2012; and my post-doctoral research at the Department of Bio-engineering, Carnegie Mellon University.
My research interests span Machine Learning, Brain-Computer Interfaces and Neuroscience. I'm interested in how human brains learn and transfer knowledge across domains, and what governing principles be adapted to learning algorithms.
My academic home page is at www.cnbc.cmu.edu/~sagi
Authored Publications
Sort By
Population Based Training as a Service
Ang Li
Ola Spyra
Valentin Dalibard
Max Jaderberg
Chenjie Gu
David Budden
Tim Harley
Pramod Gupta
NIPS Systems for ML Workshop(2018) (to appear)
Preview abstract
Population Based Training (PBT) is a recent approach that jointly optimizes neural network weights and hyperparameters which periodically copies weights of the best performers and mutates hyperparameters during training. We present in this extended abstract a generalized black-box service framework for Population Based Training. We argue that the black-box PBT service design has benefits in system scalability and required engineering effort. We perform a case study on WaveNet speech synthesis to demonstrate the effectiveness of our PBT service.
View details