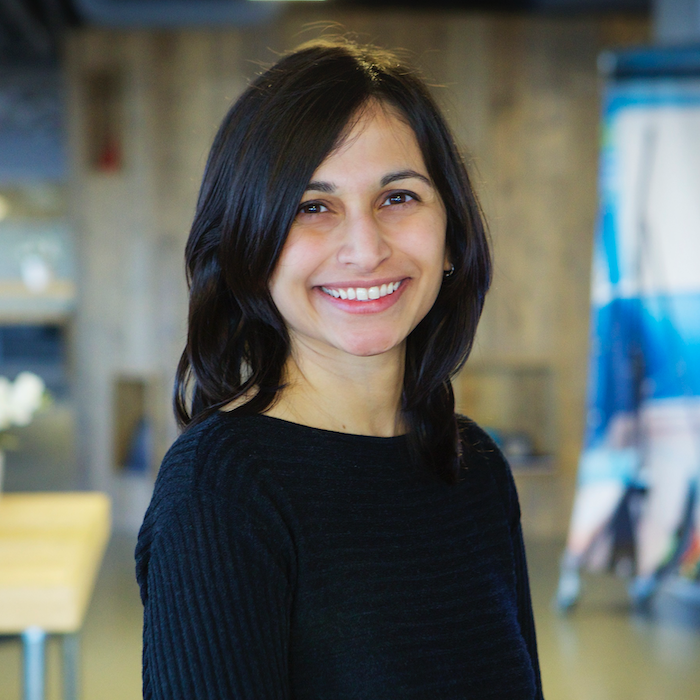
Pooja Rao
Pooja is a research scientist within Google's Health AI group.
Research Areas
Authored Publications
Google Publications
Other Publications
Sort By
Crowdsourcing Dermatology Images with Google Search Ads: Creating a Diverse and Representative Dataset of Real-World Skin Conditions
Abbi Ward
Ashley Carrick
Christopher Semturs
Dawn Siegel
Jay Hartford
Jimmy Li
Julie Wang
Justin Ko
Pradeep Kumar S
Renee Wong
Sriram Lakshminarasimhan
Steven Lin
Sunny Virmani
arXiv(2024)
Preview abstract
Background
Health datasets from clinical sources do not reflect the breadth and diversity of disease in the real world, impacting research, medical education and artificial intelligence (AI) tool development. Dermatology is a suitable area to develop and test a new and scalable method to create representative health datasets.
Methods
We used Google Search advertisements to solicit contributions of images of dermatology conditions, demographic and symptom information from internet users in the United States (US) over 265 days starting March 2023. With informed contributor consent, we described and released this dataset containing 10,106 images from 5058 contributions, with dermatologist labels as well as Fitzpatrick Skin Type and Monk Skin Tone labels for the images.
Results
We received 22 ± 14 submissions/day over 265 days. Female contributors (66.04%) and younger individuals (52.3% < age 40) had a higher representation in the dataset compared to the US population, and 36.6% of contributors had a non-White racial or ethnic identity. Over 97.5% of contributions were genuine images of skin conditions. Image quality had no impact on dermatologist confidence in assigning a differential diagnosis. The dataset consists largely of short duration (54% with onset < 7 days ago) allergic, infectious, and inflammatory conditions. Fitzpatrick skin type distribution is well-balanced, considering the geographical origin of the dataset and the absence of enrichment for population groups or skin tones.
Interpretation
Search ads are effective at crowdsourcing images of health conditions. The SCIN dataset bridges important gaps in the availability of representative images of common skin conditions.
View details
Deep learning algorithms for detection of critical findings in head CT scans: a retrospective study
Sasank Chilamkurthy
Rohit Ghosh
Swetha Tanamala
Mustafa Biviji
Norbert G Campeau
Vasantha Kumar Venugopal
Vidur Mahajan
Prashant Warier
The Lancet(2018)
Preview abstract
Background
Non-contrast head CT scan is the current standard for initial imaging of patients with head trauma or stroke symptoms. We aimed to develop and validate a set of deep learning algorithms for automated detection of the following key findings from these scans: intracranial haemorrhage and its types (ie, intraparenchymal, intraventricular, subdural, extradural, and subarachnoid); calvarial fractures; midline shift; and mass effect.
Methods
We retrospectively collected a dataset containing 313 318 head CT scans together with their clinical reports from around 20 centres in India between Jan 1, 2011, and June 1, 2017. A randomly selected part of this dataset (Qure25k dataset) was used for validation and the rest was used to develop algorithms. An additional validation dataset (CQ500 dataset) was collected in two batches from centres that were different from those used for the development and Qure25k …
View details
Machine Learning Methods Improve Prognostication, Identify Clinically Distinct Phenotypes, and Detect Heterogeneity in Response to Therapy in a Large Cohort of Heart Failure Patients
Tariq Ahmad
Lars H. Lund
Rohit Ghosh
Prashant Warier
Benjamin Vaccaro,
Ulf Dahlström
Christopher M. O'Connor
G. Michael Felker
Nihar R. Desai
Journal of the American Heart Association(2018)
Preview abstract
Background
Whereas heart failure (HF) is a complex clinical syndrome, conventional approaches to its management have treated it as a singular disease, leading to inadequate patient care and inefficient clinical trials. We hypothesized that applying advanced analytics to a large cohort of HF patients would improve prognostication of outcomes, identify distinct patient phenotypes, and detect heterogeneity in treatment response.
Methods and Results
The Swedish Heart Failure Registry is a nationwide registry collecting detailed demographic, clinical, laboratory, and medication data and linked to databases with outcome information. We applied random forest modeling to identify predictors of 1‐year survival. Cluster analysis was performed and validated using serial bootstrapping. Association between clusters and survival was assessed with Cox proportional hazards modeling and interaction testing was performed to assess for heterogeneity in response to HF pharmacotherapy across propensity‐matched clusters. Our study included 44 886 HF patients enrolled in the Swedish Heart Failure Registry between 2000 and 2012. Random forest modeling demonstrated excellent calibration and discrimination for survival (C‐statistic=0.83) whereas left ventricular ejection fraction did not (C‐statistic=0.52): there were no meaningful differences per strata of left ventricular ejection fraction (1‐year survival: 80%, 81%, 83%, and 84%). Cluster analysis using the 8 highest predictive variables identified 4 clinically relevant subgroups of HF with marked differences in 1‐year survival. There were significant interactions between propensity‐matched clusters (across age, sex, and left ventricular ejection fraction and the following medications: diuretics, angiotensin‐converting enzyme inhibitors, β‐blockers, and nitrates, P<0.001, all).
Conclusions
Machine learning algorithms accurately predicted outcomes in a large data set of HF patients. Cluster analysis identified 4 distinct phenotypes that differed significantly in outcomes and in response to therapeutics. Use of these novel analytic approaches has the potential to enhance effectiveness of current therapies and transform future HF clinical trials.
View details
MicroRNAs as biomarkers for CNS disease
Preview abstract
For many neurological diseases, the efficacy and outcome of treatment depend on early detection. Diagnosis is currently based on the detection of symptoms and neuroimaging abnormalities, which appear at relatively late stages in the pathogenesis. However, the underlying molecular responses to genetic and environmental insults begin much earlier and non-coding RNA networks are critically involved in these cellular regulatory mechanisms. Profiling RNA expression patterns could thus facilitate presymptomatic disease detection. Obtaining indirect readouts of pathological processes is particularly important for brain disorders because of the lack of direct access to tissue for molecular analyses. Living neurons and other CNS cells secrete microRNA and other small non-coding RNA into the extracellular space packaged in exosomes, microvesicles or lipoprotein complexes. This discovery, together with the rapidly evolving massive sequencing technologies that allow detection of virtually all RNA species from small amounts of biological material, has allowed significant progress in the use of extracellular RNA as a biomarker for CNS malignancies, neurological and psychiatric diseases. There is also recent evidence that the interactions between external stimuli and brain pathological processes may be reflected in peripheral tissues, facilitating their use as potential diagnostic markers. In this review, we explore the possibilities and challenges of using microRNA and other small RNAs as a signature for neurodegenerative and other neuropsychatric conditions.
View details