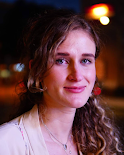
Polina Zablotskaia
Polina is an NLP and ML researcher working at Google since 2020. Prior to that she was at the University of British Columbia, where she worked on generative models for video generation.
Research Areas
Authored Publications
Google Publications
Other Publications
Sort By
Will you Find these Shortcuts? A Protocol for Evaluating Faithfulness of Input Salience Methods for Text Classification
Sebastian Ebert
Proceedings of EMNLP 2022 (to appear)
Preview abstract
Feature attribution a.k.a. input salience methods which assign an importance score to a feature are abundant but may produce surprisingly different results for the same model on the same input. While differences are expected if disparate definitions of importance are assumed, most methods claim to provide faithful attributions and point at features most relevant for a model's prediction. Existing work on faithfulness evaluation is not conclusive and does not provide a clear answer as to how different methods are to be compared.
Focusing on text classification and the model debugging scenario, we propose a protocol for faithfulness evaluation which makes use of partially synthetic data to obtain ground truth for feature importance ranking.
Following the protocol, we do an in-depth analysis of four standard salience method classes on a range of datasets and shortcuts for BERT and LSTM models. We demonstrate that some of the most common method configurations provide poor results even for simplest shortcuts while a method judged to be too simplistic works remarkably well for BERT.
View details
Preview abstract
We address efficient calculation of influence functions (Koh & Liang 2017) for tracking predictions back to the training data. We propose and analyze a new approach to speeding up the inverse Hessian calculation based on Arnoldi iteration (Arnoldi 1951). With this improvement, we achieve, to the best of our knowledge, the first successful implementation of influence functions that scales to full-size (language and vision) Transformer models with several hundreds of millions of parameters. We evaluate our approach in image classification and sequence-to-sequence tasks with tens to a hundred of millions of training examples. Our implementation will be publicly available at https://github.com/google-research/jax-influence.
View details
No Results Found