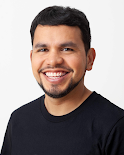
Mario Guajardo-Céspedes
Mario’s current work focuses on applied Machine Learning within Google Research focusing on leveraging large language models (LLMs) for new experiences like Gmail's "Help me write". He also focuses on AI-for-social-good as well as ML-fairness efforts. More recently, he has been focusing on strengthening the AI ecosystem in Latin America as an ML-in-residence advisor for the Google for Startups Accelerator in Latin America, as well as an ML-advisor for Google’s AI Impact Challenge. He is also a fellow of the People+AI Guidebook---a framework to help develop human-centered AI products--- focusing on outreach in Latin America.
In previous roles, Mario has helped take projects from early-stage to launch for Waze Carpool, Search, and Cloud. He also contributes to mentoring and advocacy efforts, and on using technology to solve tough social issues.
Authored Publications
Google Publications
Other Publications
Sort By
Universal Sentence Encoder
Yinfei Yang
Sheng-yi Kong
Nan Hua
Nicole Lyn Untalan Limtiaco
Rhomni St. John
Steve Yuan
Chris Tar
Brian Strope
Ray Kurzweil
In submission to: EMNLP demonstration, Association for Computational Linguistics, Brussels, Belgium (2018)
Preview abstract
We present models for encoding sentences into embedding vectors that specifically target transfer learning to other NLP tasks. The models are efficient and result in accurate performance on diverse transfer tasks. Two variants of the encoding models allow for trade-offs between accuracy and compute resources. For both variants, we investigate and report the relationship between model complexity, resource consumption, the availability of transfer task training data, and task performance. Comparisons are made with baselines that use word level transfer learning via pretrained word embeddings as well as baselines do not use any transfer learning. We find that transfer learning using sentence embeddings tends to outperform word level transfer. With transfer learning via sentence embeddings, we observe surprisingly good performance with minimal amounts of supervised training data for a transfer task. We obtain encouraging results on Word Embedding Association Tests (WEAT) targeted at detecting model bias. Our pre-trained sentence encoding models are made freely available for download and on TF Hub.
View details
Preview abstract
With the public release of embedding models, it’s important to understand the various biases that they contain. Developers who use them should be aware of the biases inherent in the models as well as how biases can manifest in downstream applications that use these models. In this post, we examine a few specific forms of bias and suggest tools for evaluating as well as mitigating bias.
View details
No Results Found