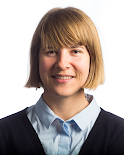
Mar Gonzalez-Franco
Mar Gonzalez-Franco, PhD, is a Computer Scientist and Neuroscientist at Google working on a new generation of Immersive technologies. With a background in real-time systems in her research she tries to build better interactions for immersive technologies using different disciplines: Virtual Reality, Augmented Reality, Avatars, computer graphics, computer vision and haptics. All while studying human behavior, perception and neuroscience.
Apart from her scientific contributions, she has a deep interest in helping the community grow more diverse and was awarded the 2022 IEEE VGTC VR New Researcher Award.
Authored Publications
Google Publications
Other Publications
Sort By
Preview abstract
This workshop aims to unite experts and practitioners in XR and AI to envision the future of AI-enabled virtual, augmented, and mixed reality experiences. Our expansive discussion includes a
variety of key topics: Generative XR, Large Language Models (LLMs) for XR, Adaptive and Context-Aware XR, Explainable AI for XR, and harnessing AI to enhance and prototype XR experiences. We aim to identify the opportunities and challenges of how recent advances of AI could bring new XR experiences, which cannot be done before, with a keen focus on the seamless blending of our digital and physical worlds.
View details
The Work Avatar Face-Off: Knowledge Worker Preferences for Realism in Meetings
Kristin Moore
22nd IEEE International Symposium on Mixed and Augmented Reality (ISMAR) (2023) (to appear)
Preview abstract
While avatars have grown in popularity in social settings, their use in the workplace is still debatable. We conducted a large-scale survey to evaluate knowledge worker sentiment towards avatars, particularly the effects of realism on their acceptability for work meetings. Our survey of 2509 knowledge workers from multiple countries rated five avatar styles for use by managers, known colleagues and unknown colleagues.
In all scenarios, participants favored higher realism, but fully realistic avatars were sometimes perceived as uncanny. Less realistic avatars were rated worse when interacting with an unknown colleague or manager, as compared to a known colleague. Avatar acceptability varied by country, with participants from the United States and South Korea rating avatars more favorably. We supplemented our quantitative findings with a thematic analysis of open-ended responses to provide a comprehensive understanding of factors influencing work avatar choices.
In conclusion, our results show that realism had a significant positive correlation with acceptability. Non-realistic avatars were seen as fun and playful, but only suitable for occasional use.
View details
Preview abstract
Producing quality segmentation masks for images is a fundamental problem in computer vision. Recent research has explored large-scale supervised training to enable zero-shot segmentation on virtually any image style and unsupervised training to enable segmentation without dense annotations. However, constructing a model capable of segmenting anything in a zero-shot manner without any annotations is still challenging. In this paper, we propose to utilize the self-attention layers in stable diffusion models to achieve this goal because the pre-trained stable diffusion model has learned inherent concepts of objects within its attention layers. Specifically, we introduce a simple yet effective iterative merging process based on measuring KL divergence among attention maps to merge them into valid segmentation masks. The proposed method does not require any training or language dependency to extract quality segmentation for any images. On COCO-Stuff-27, our method surpasses the prior unsupervised zero-shot SOTA method by an absolute 26% in pixel accuracy and 17% in mean IoU.
View details
Preview abstract
With the arrival of immersive technologies, virtual avatars have gained a prominent role in the future of social computing. However, there is a lack of free resources that can provide researchers with diverse sets of virtual avatars, and the few that are available have not been validated. In this paper, we present VALID a new, freely available 3D avatar library. VALID includes 210 fully rigged avatars that were modeled through an iterative design process and represent the seven ethnicities recommended by U.S. Census Bureau research. We validated the avatars through a user study with participants (n = 132) from 33 countries, and provide statistically validated labels for each avatar’s perceived ethnicity and gender. Through our validation, we also advance the understanding of avatar ethnicity and show it can replicate the human psychology phenomenon of own-race bias in face recognition.
View details
No Results Found