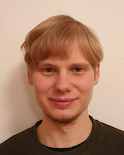
Julian Zimmert
Julian is passionate about deriving principled solutions for challenging applications. He completed his PhD at the University of Copenhagen about adversarially robust multi-armed bandits problems and has hence worked on a range of problems in online learning and reinforcement learning.
Research Areas
Authored Publications
Google Publications
Other Publications
Sort By
A Model Selection Approach for Corruption Robust Reinforcement Learning
Chen-Yu Wei
33rd International Conference on Algorithmic Learning Theory (ALT 2022) (2022)
Preview abstract
We develop a model selection approach to tackle reinforcement learning with adversarial corruption in both transition and reward. For finite-horizon tabular MDPs, without prior knowledge on the total amount of corruption, our algorithm achieves a regret bound of O(min{1/∆,√T}+C)where T is the number of episodes, C is the total amount of corruption, and ∆ is the reward gap between the best and the second-best policies. This is the first worst-case optimal bound achieved without knowledge of C, improving previous results of Lykouris et al. (2021); Chen et al. (2021); Wu et al.(2021). For finite-horizon linear MDPs, we develop a computationally efficient algorithm with a regret bound of ̃O(√(1 +C)T), and another computationally inefficient one with O(√T+C),improving the result of Lykouris et al. (2021) and answering an open question by Zhang et al.(2021b). Finally, our model selection framework can be easily applied to other settings including linear bandits, linear contextual bandits, and MDPs with general function approximation, leading to several improved or new results.
View details
Preview abstract
Multiclass logistic regression is a fundamental task in machine learning with applications in classification and boosting. Previous work (Foster et a. 2018) has highlighted the importance of improper predictors for achieving ``fast rates'' in the online multiclass logistic regression problem without suffering exponentially from secondary problem parameters, such as the norm of the predictors in the comparison class. While Foster et al. introduced a statistically optimal algorithm, it is in practice computationally intractable due to its run-time complexity being a large polynomial in the time horizon and dimension of input feature vectors. It has remained an open problem if algorithms exist that are both statistically and computationally efficient. Jezequel et al. answered this question for binary logistic regression by introducing AIOLI, an algorithm based on online newton step.
However, their technique fails to generalize to more than two classes and it remains open whether their algorithm can be applied to practical applications of logistic regression, such as bandit multiclass learning or online boosting.
In this paper, we develop a new algorithm, FOLKLORE, for the problem which runs significantly faster than the algorithm of Foster et al. -- the running time per iteration scales quadratically in the dimension -- at the cost of a linear dependence on the norm of the predictors in the regret bound. This yields the first practical algorithm for online multiclass logistic regression, resolving an open problem of Jezequel et al. We resolve the open questions by deriving an extension of AIOLI to the multi-class setting.
Furthermore, we show that our algorithm can be applied to online bandit multiclass prediction and online multiclass boosting, yielding more practical algorithms for both problems compared to the ones in Foster et al. with similar performance guarantees. Finally, we also provide an online-to-batch conversion result for our algorithm.
View details
No Results Found