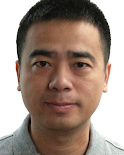
Jin Xu
Jin is a ML researcher at Applied Science of Google Research. His research interests include deep learning, AI4Science and information theory. He is currently working on developing computer vision segmentation models to automate neuron tracing for mouse brain reconstruction. Previously he worked on applying deep learning to hit finding and lead optimization in drug discovery. Google Scholar
Research Areas
Authored Publications
Google Publications
Other Publications
Sort By
Deep Learning Approach for the Discovery of Tumor-Targeting Small Organic Ligands from DNA-Encoded Chemical Libraries
Wen Torng
Ilaria Biancofiore
Sebastian Oehler
Jessica Xu
Ian Allen Watson
Brenno Masina
Luca Prati
Nicholas Favalli
Gabriele Bassi
Dario Neri
Samuele Cazzamalli
JW Feng
ACS Omega (2023)
Preview abstract
DNA-Encoded Chemical Libraries (DELs) have emerged as efficient and cost-effective ligand discovery tools, which enable the generation of protein-ligand interaction data of unprecedented size. In this article, we present an approach that combines DEL screening and instance-level deep learning modeling to identify tumor-targeting ligands against Carbonic Anhydrase IX (CAIX), a clinically validated marker of hypoxia and clear cell Renal Cell Carcinoma. We present a new ligand identification and hit-to-lead strategy driven by machine learning models trained on DELs, which expand the scope of DEL-derived chemical motifs. CAIX-screening datasets obtained from three different DELs were used to train machine learning models for generating novel hits, dissimilar to elements present in the original DELs. Out of the 152 novel potential HITs that were identified with our approach and screened in an in vitro enzymatic inhibition assay, 70% displayed submicromolar activities (IC50 < 1 µM). To generate lead compounds that are functionalized with anticancer payloads, analogues of top hits were prioritized for synthesis based on the predicted CAIX affinity and synthetic feasibility. Three LEAD candidates showed accumulation on the surface of CAIX-expressing tumor cells in cellular binding assays. The best compound displayed an in vitro KD of 5.7 nM and selectively targeted tumors in mice bearing human Renal Cell Carcinoma lesions. Our results demonstrate the synergy between DEL and machine learning for the identification of novel HITs and for the successful translation of LEAD candidates for in vivo targeting applications.
View details
Hit Expansion Driven By Machine Learning
JW Feng
Steven Kearnes
NeurIPS 2023 Workshop on New Frontiers of AI for Drug Discovery and Development (2023)
Preview abstract
Recent work \cite{McCloskey2020-es} utilized experimental data from DNA-encoded library (DEL) selections to train graph convolutional neural networks (GCNNs) \cite{Kearnes2016-sk} for identifying hit compounds for protein targets and their prospective test results demonstrated unprecedented hit rates for three diverse proteins. Building on this work, we proposed two novel approaches to leverage the DEL GCNN model's predictions and embeddings to automate hit expansion, a critical step in real-world drug discovery that guides the optimization of initial hit compounds toward clinical candidates. We prospectively tested the proposed approaches on a protein target (sEH) in the wet lab. The results showed that our methods identified more small molecules with higher potency compared to traditional molecular fingerprint similarity search based hit expansion. Specifically, we discovered $34$ analog compounds with higher potency than a sEH clinical trial candidate using our approaches. All sEH assay results are available at \url{https://www.tdcommons.org/dpubs_series/7414/}. Furthermore, applying the automated hit expansion approach to a novel protein target (WDR91) without prior known inhibitors, we discovered 2 active covalent analogs, representing the first reported small molecule ligands for this previously unexplored target.
View details
Discovery of a first-in-class small molecule ligand for WDR91 using DNA-encoded library selection followed by machine learning
Shabbir Ahmad
JW Feng
Ashley Hutchinson
Hong Zeng
Pegah Ghiabi
Aiping Dong
Paolo A. Centrella
Matthew A. Clark
Marie-Aude Guié
John P. Guilinger
Anthony D. Keefe
Ying Zhang
Thomas Cerruti
John W. Cuozzo
Moritz von Rechenberg
Albina Bolotokova
Yanjun Li
Peter Loppnau
Almagul Seitova
Yen-Yen Li
Vijayaratnam Santhakumar
Peter J. Brown
Suzanne Ackloo
Levon Halabelian
Journal of Medicinal Chemistry (2023)
Preview abstract
WD40 repeat-containing protein 91 regulates endosomal phosphatidylinositol 3-phosphate levels at the critical stage of endosome maturation and plays vital roles in endosome fusion, recycling, and transport by mediating protein-protein interactions. Due to its various roles in endocytic pathways, WDR91 has recently been identified as a potential host factor responsible for viral infection. We employed DNA-Encoded Chemical Library (DEL) selections against the WDR domain of WDR91, followed by machine learning to generate a model that was then used to predict ligands from the synthetically accessible Enamine REAL database. Screening of predicted compounds enabled us to identify the hit compound 1, which binds to WDR91 with a KD of 6±2 µM by surface plasmon resonance. Our co-crystal structure confirmed binding of 1 to the WDR91 side pocket, in a proximity of cysteine 487. Machine learning-assisted structure activity relationship-by-catalog validated the chemotype of 1 and led to the discovery of covalent analogs 18 and 19. Intact mass liquid chromatography/mass spectrometry and differential scanning fluorimetry confirmed the formation of a covalent adduct, and thermal stabilization, respectively. The discovery of 1, 18, 19 and accompanying SAR will provide valuable insights for designing more potent and selective compounds against WDR91, thus accelerating the development of novel chemical tools to evaluate the therapeutic potential of WDR91 in diseases.
View details
Preview abstract
DNA-Encoded Libraries (DEL) data, often with millions of data points, enables large deep learning models to make real contributions in drug discovery (e.g., hit-finding). The state-of-the-art method of modeling DEL data, GCNN multiclass model, requires domain experts to create mutually exclusive classification labels from multiple selection readouts of DEL data, which is not always an optimal formulation. In this work, we designed a GCNN multilabel architecture that directly models each selection data to eliminate dependency on human expertise. We selected effective choices for key modeling components such as label reduction scheme from in silico evaluation. To assess its performance in real-world drug discovery settings, we further carried out prospective wet-lab testing where the multilabel model shows consistent improvement in hit-rate (percentage of hits in a proposed molecule list) over the state-of-the-art multiclass model.
View details
No Results Found