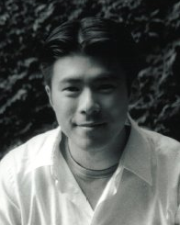
Hank Liao
Hank Liao is a researcher in the speech recognition group with interests in acoustic modeling and large vocabulary speech recognition.
Hank Liao obtained a BASc in Systems Design Engineering from the University of Waterloo in 1999. In 2002 he completed an MPhil from the University of Cambridge. In 2007 he submitted his dissertation on "Uncertainty Decoding for Noise Robust Speech Recognition" to the Cambridge University Engineering Department, supervised by Dr. Mark Gales, and sponsored by Toshiba Research Europe. He has published research on and deployed automatic captioning of YouTube videos, Google Voice's voicemail transcription, and Voice Search speech recognition systems.
Authored Publications
Sort By
USM-SCD: USM-Based Multilingual Speaker Change Detection
Yongqiang Wang
Jason Pelecanos
Yu Zhang
Yiling Huang
Han Lu
ICASSP 2024 - 2024 IEEE International Conference on Acoustics, Speech and Signal Processing (ICASSP), pp. 11801-11805
Preview abstract
We introduce a multilingual speaker change detection model (USM- SCD) that can simultaneously detect speaker turns and perform ASR for 96 languages. This model is adapted from a speech foundation model trained on a large quantity of supervised and unsupervised data, demonstrating the utility of fine-tuning from a large generic foundation model for a downstream task. We analyze the performance of this multilingual speaker change detection model through a series of ablation studies. We show that the USM-SCD model can achieve more than 75% average speaker change detection F1 score across a test set that consists of data from 96 languages. On American English, the USM-SCD model can achieve an 85.8% speaker change detection F1 score across various public and internal test sets, beating the previous monolingual baseline model by 21% relative. We also show that we only need to fine-tune one-quarter of the trainable model parameters to achieve the best model performance. The USM-SCD model exhibits state-of-the-art ASR quality compared with a strong public ASR baseline, making it suitable to handle both tasks with negligible additional computational cost.
View details
On Robustness to Missing Video For Audiovisual Speech Recognition
Dmitriy (Dima) Serdyuk
Transactions on Machine Learning Research (2022)
Preview abstract
It has been shown that learning audiovisual features can lead to improved speech recognition performance over audio-only features, especially for noisy speech. However, in many common applications, the visual features are partially or entirely missing, e.g.~the speaker might move off screen. Multi-modal models need to be robust: missing video frames should not degrade the performance of an audiovisual model to be worse than that of a single-modality audio-only model. While there have been many attempts at building robust models, there is little consensus on how robustness should be evaluated. To address this, we introduce a framework that allows claims about robustness to be evaluated in a precise and testable way. We also conduct a systematic empirical study of the robustness of common audiovisual speech recognition architectures on a range of acoustic noise conditions and test suites. Finally, we show that an architecture-agnostic solution based on cascades can consistently achieve robustness to missing video, even in settings where existing techniques for robustness like dropout fall short.
View details
Preview abstract
Traditionally, audio-visual automatic speech recognition has been studied under the assumption that the speaking face on the visual signal is the face matching the audio. However, in a more realistic setting, when multiple faces are potentially on screen one needs to decide which face to feed to the A/V ASR system. The present work takes the recent progress of A/V ASR one step further and considers the scenario where multiple people are simultaneously on screen (multi-person A/V ASR). We propose a fully differentiable A/V ASR model that is able to handle multiple face tracks in a video. Instead of relying on two separate models for speaker face selection and audio-visual ASR on a single face track, we introduce an attention layer to the ASR encoder that is able to soft-select the appropriate face video track. Experiments carried out on an A/V system trained on over 30k hours of YouTube videos illustrate that the proposed approach can automatically select the proper face tracks with minor WER degradation compared to an oracle selection of the speaking face while still showing benefits of employing the visual signal instead of the audio alone.
View details
RECURRENT NEURAL NETWORK TRANSDUCER FOR AUDIO-VISUAL SPEECH RECOGNITION
Basi Garcia
Brendan Shillingford
Yannis Assael
Proceedings of IEEE Automatic Speech Recognition and Understanding Workshop (2019)
Preview abstract
This work presents a large-scale audio-visual speech recognition system based on a recurrent neural network transducer (RNN-T) architecture. To support the development of such a system, we built a large audio-visual (AV) dataset of segmented utterances extracted from YouTube public videos, leading to 31k hours of audio-visual training content. The performance of an audio-only, visual-only, and audio-visual system are compared on two large-vocabulary test sets: an internal set of YouTube utterances (YouTube-AV-Dev-18) and the publicly available TED-LRS3 set. To highlight the contribution of the visual modality, we also evaluated the performance of our system on the YouTube-AV-Dev-18 set artificially corrupted with additive background noise and overlapping speech. To the best of our knowledge, our system significantly improves the state-of-the-art on the TED-LRS3 set.
View details
Preview abstract
Multilingual training has proven to improve acoustic modeling performance by sharing and transferring knowledge in modeling different languages. Knowledge sharing is usually achieved by using common lower-level layers for different languages in a deep neural network. Recently, the domain adversarial network was proposed to reduce domain mismatch of training data and learn domain-invariant features. It is thus worth exploring whether adversarial training can further promote knowledge sharing in multilingual models. In this work, we apply the domain adversarial network to encourage the shared layers of a multilingual model to learn language-invariant features. Bidirectional Long Short-Term Memory (LSTM) recurrent neural networks (RNN) are used as building blocks. We show that shared layers learned this way contain less language identification information and lead to better acoustic modeling performance. In an automatic speech recognition task for seven languages, the resultant acoustic model improves the word error rate (WER) of the multilingual model by a relative 4% on average, and the monolingual models by 10%.
View details
Lattice Rescoring Strategies for Long Short Term Memory Language Models in Speech Recognition
Michael Alexander Nirschl
Dan Holtmann-Rice
(2017)
Preview abstract
Recurrent neural network language models (RNNLM) and Long Short Term Memory (LSTM) LMs, a variant of RNN LMs, have been shown to outperform traditional N-gram LMs on speech recognition tasks. However, these models are computationally more expensive than N-gram LMs for decoding, and thus, challenging to integrate into speech recognizers. Recent research has proposed the use of lattice-rescoring algorithms using RNNLMs and LSTMLMs as an efficient strategy to integrate these models into a speech recognition system. In this paper, we evaluate existing lattice rescoring algorithms along with a few of our own novel variants on a Youtube speech recognition task. Lattice rescoring using LSTMLMs reduces the word error rate (WER) for this task by about 6\% relative to the WER obtained using an N-gram LM.
View details
Preview abstract
We present a new algorithm for efficiently training n-gram language models on uncertain data, and illustrate its use for semi-supervised language model adaptation. We compute the probability that an n-gram occurs k times in the sample of uncertain data, and use the resulting histograms to derive a generalized Katz backoff model. We compare semi-supervised adaptation of language models for YouTube video speech recognition in two conditions: when using full lattices with our new algorithm versus just the 1-best output from the baseline speech recognizer. Unlike 1-best methods, the new algorithm provides models that yield solid improvements over the baseline on the full test set, and, further, achieves these gains without hurting performance on any of the set of channels. We show that channels with the most data yielded the largest gains. The algorithm was implemented via a new semiring in the OpenFst library and will be released as part of the OpenGrm ngram library.
View details
Preview abstract
We present results that show it is possible to build a competitive, greatly simplified, large vocabulary continuous speech recognition system with whole words as acoustic units. We model the output vocabulary of about 100,000 words directly using deep bi-directional LSTM RNNs with CTC loss. The model is trained on 125,000 hours of semi-supervised acoustic training data, which enables us to alleviate the data sparsity problem for word models. We show that the CTC word models work very well as an end-to-end all-neural speech recognition model without the use of traditional context-dependent sub-word phone units that require a pronunciation lexicon, and without any language model removing the need to decode. We demonstrate that the CTC word models perform better than a strong, more complex, state-of-the-art baseline with sub-word units.
View details
Large Vocabulary Automatic Speech Recognition for Children
Melissa Carroll
Noah Coccaro
Qi-Ming Jiang
Interspeech (2015)
Preview abstract
Recently, Google launched YouTube Kids, a mobile application for children, that uses a speech recognizer built specifically for recognizing children’s speech. In this paper we present techniques we explored to build such a system. We describe the use of a neural network classifier to identify matched acoustic training data, filtering data for language modeling to reduce the chance of producing offensive results. We also compare long short-term memory (LSTM) recurrent networks to convolutional, LSTM, deep neural networks (CLDNN). We found that a CLDNN acoustic model outperforms an LSTM across a variety of different conditions, but does not specifically model child speech relatively better than adult. Overall, these findings allow us to build a successful, state-of-the-art large vocabulary speech recognizer for both children and adults.
View details
GMM-Free DNN Training
Preview
Proceedings of the International Conference on Acoustics,Speech and Signal Processing (2014)