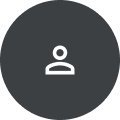
Gary Wang
Researcher working on Speech Recognition and Text to Speech.
Research Areas
Authored Publications
Google Publications
Other Publications
Sort By
Virtuoso: Massive Multilingual Speech-Text Joint Semi-Supervised Learning for Text-to-Speech
Takaaki Saeki
Zhehuai Chen
Nobuyuki Morioka
Yu Zhang
ICASSP (2023)
Preview abstract
This paper proposes Virtuoso, a massive multilingual speech–text joint learning framework for text-to-speech synthesis (TTS) models. Existing multilingual TTS typically supports tens of languages, which are a small fraction of thousands of languages in the world. One difficulty to scale multilingual TTS to hundreds of languages is collecting high-quality speech–text paired data in low-resource languages. This study extends Maestro, which is a speech–text semi-supervised joint pretraining framework for automatic speech recognition (ASR), to speech generation tasks. To train a TTS model from various types of speech and text data, different training schemes are designed to handle supervised (paired TTS and ASR data) and unsupervised (untranscribed speech and unspoken text) datasets. Experimental evaluation shows that 1) multilingual TTS models trained on Virtuoso can achieve significantly better naturalness and intelligibility than baseline TTS models in seen languages, and 2) these models can synthesize reasonably good speech for unseen languages where no paired TTS data is available.
View details
Accented Speech Recognition: Benchmarking, Pre-training, and Diverse Data
Zhehuai Chen
Chung-Cheng Chiu
Pavel Golik
Wei Han
Levi King
Suzan Schwartz
(2022)
Preview abstract
Building inclusive speech recognition systems is a crucial step towards developing technologies that speakers of all language varieties can use. Therefore, ASR systems must work for everybody independently of the way they speak. To accomplish this goal, there should be available data sets representing language varieties, and also an understanding of model configuration that is the most helpful in achieving robust understanding of all types of speech. However, there are not enough data sets for accented speech, and for the ones that are already available, more training approaches need to be explored to improve the quality of accented speech recognition. In this paper, we discuss recent progress towards developing more inclusive ASR systems, namely, the importance of building new data sets representing linguistic diversity, and exploring novel training approaches to improve performance for all users. We address recent directions within benchmarking ASR systems for accented speech, measure the effects of wav2vec 2.0 pre-training on accented speech recognition, and highlight corpora relevant for diverse ASR evaluations.
View details
Semi-Supervision in ASR: Sequential Mixmatch and Factorized TTS-Based Augmentation
Zhehuai Chen
Yu Zhang
Yinghui Huang
Jesse Emond
Pedro Jose Moreno Mengibar
(2021)
Preview abstract
Semi- and self-supervised training techniques have the potential to improve performance of speech recognition systems without additional transcribed speech data. In this work, we demonstrate the efficacy of two approaches to semi-supervision for automated speech recognition. The two approaches lever-age vast amounts of available unspoken text and untranscribed audio. First, we present factorized multilingual speech synthesis to improve data augmentation on unspoken text. Next, we present an online implementation of Noisy Student Training to incorporate untranscribed audio. We propose a modified Sequential MixMatch algorithm with iterative learning to learn from untranscribed speech. We demonstrate the compatibility of these techniques yielding a relative reduction of word error rate of up to 14.4% on the voice search task.
View details
Improving Speech Recognition using GAN-based Speech Synthesis and Contrastive Unspoken Text Selection
Zhehuai Chen
Yu Zhang
Pedro Jose Moreno Mengibar
Interspeech 2020
Preview abstract
Text-to-Speech synthesis (TTS) based data augmentation is a relatively new mechanism for utilizing text-only data to improve automatic speech recognition (ASR) training without parameter or inference architecture changes. However, efforts to train speech recognition systems on synthesized utterances suffer from limited acoustic diversity of TTS outputs. Additionally, the text-only corpus is always much larger than the transcribed speech corpus by several orders of magnitude, which makes speech synthesis of all the text data impractical. In this work, we propose to combine generative adversarial network (GAN) and multi-style training (MTR) to increase acoustic diversity in the synthesized data. We also present a contrastive language model-based data selection technique to improve the efficiency of learning from unspoken text. We demonstrate the ability of our proposed method to enable efficient, large-scale unspoken text learning which achieving a 32.7\% relative WER reduction on a voice-search task.
View details
SCADA: Stochastic, Consistent and Adversarial Data Augmentation to Improve ASR
Zhehuai Chen
Yu Zhang
Pedro Moreno
Proceedings of Interspeech 2020, pp. 2832-2836
Preview abstract
Recent developments in data augmentation has brought great gains in improvement for automatic speech recognition (ASR). Parallel developments in augmentation policy search in computer vision domain has shown improvements in model performance and robustness. In addition, recent developments in semi-supervised learning has shown that consistency measures are crucial for performance and robustness. In this work, we demonstrate that combining augmentation policies with consistency measures and model regularization can greatly improve speech recognition performance. Using the Librispeech task, we show: 1) symmetric consistency measures such as the Jensen-Shannon Divergence provide 11\% relative improvements in ASR performance; 2) Augmented adversarial inputs using Virtual Adversarial Noise (VAT) provides 8.9\% relative win; and 3) random sampling from arbitrary combination of augmentation policies yields the best policy. These contributions result in an overall reduction in Word Error Rate (WER) of 18\% relative on the Librispeech task presented in this paper.
View details
Improving Speech Recognition Using Consistent Predictions on Synthesized Speech
Zhehuai Chen
Yu Zhang
Pedro Jose Moreno Mengibar
IEEE ICASSP 2020
Preview abstract
Speech synthesis has advanced to the point of being close to indistinguishable from human speech. However, efforts to train speech recognition systems on synthesized utterances have not been able to show that synthesized data can be effectively used to augment or replace human speech.
In this work, we demonstrate that promoting consistent predictions in response to real and synthesized speech enables significantly improved speech recognition performance.
We also find that training on 460 hours of LibriSpeech augmented with 500 hours of transcripts (without audio) performance is within 0.2\% WER of a system trained on 960 hours of transcribed audio. This suggests that with this approach, when there is sufficient text available, reliance on transcribed audio can be cut nearly in half.
View details
No Results Found