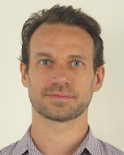
Andrew Rosenberg
Andrew Rosenberg is a Research Scientist at Google. Previously he was a Research Staff Member at IBM, and a Professor at CUNY Queens College and The CUNY Graduate Center.
Research Areas
Authored Publications
Google Publications
Other Publications
Sort By
Virtuoso: Massive Multilingual Speech-Text Joint Semi-Supervised Learning for Text-to-Speech
Takaaki Saeki
Zhehuai Chen
Nobuyuki Morioka
Yu Zhang
ICASSP (2023)
Preview abstract
This paper proposes Virtuoso, a massive multilingual speech–text joint learning framework for text-to-speech synthesis (TTS) models. Existing multilingual TTS typically supports tens of languages, which are a small fraction of thousands of languages in the world. One difficulty to scale multilingual TTS to hundreds of languages is collecting high-quality speech–text paired data in low-resource languages. This study extends Maestro, which is a speech–text semi-supervised joint pretraining framework for automatic speech recognition (ASR), to speech generation tasks. To train a TTS model from various types of speech and text data, different training schemes are designed to handle supervised (paired TTS and ASR data) and unsupervised (untranscribed speech and unspoken text) datasets. Experimental evaluation shows that 1) multilingual TTS models trained on Virtuoso can achieve significantly better naturalness and intelligibility than baseline TTS models in seen languages, and 2) these models can synthesize reasonably good speech for unseen languages where no paired TTS data is available.
View details
Reducing domain mismatch in self-supervised speech pretraining
Yu Zhang
submission to Interspeech 2022 (2022) (to appear)
Preview abstract
Masked speech modeling (MSM) methods such as wav2vec2 or w2v-BERT learn representations over speech frames which are randomly masked within an utterance. While these methods improve performance of Automatic Speech Recognition (ASR) systems, they have one major limitation. They treat all unsupervised speech samples with equal weight, which hinders learning as not all samples have relevant information to learn meaningful representations. In this work, we address this limitation. We propose ask2mask (ATM), a novel approach to focus on specific samples during MSM pre-training. ATM employs an external ASR model or scorer to weight unsupervised input samples in two different ways: 1) A fine-grained data selection is performed by masking over the highly confident input frames as
chosen by the scorer. This allows the model to learn meaningful representations. 2) ATM is further extended to focus at utterancelevel by weighting the final MSM loss with the utterancelevel confidence score. We conduct fine-tuning experiments on two well-benchmarked corpora: LibriSpeech (matching the pretraining data) and Commonvoice, TED-LIUM, AMI and CHiME6 (not matching the pre-training data). The results substantiate the efficacy of ATM on significantly improving the recognition
performance under mismatched conditions (up to 11.6% relative over published results and upto 4.46% relative over our internal baseline) while still yielding modest improvements under matched
conditions.
View details
MAESTRO: Matched Speech Text Representations through Modality Matching
Pedro Jose Moreno Mengibar
Yu Zhang
Zhehuai Chen
interspeech 2022 (2022) (to appear)
Preview abstract
We present Maestro, a self-supervised training method to
unify representations learnt from speech and text modalities.
Self-supervised learning from speech signals aims to learn the
latent structure inherent in the signal, while self-supervised
learning from text attempts to capture lexical information.
Learning aligned representations from unpaired speech and
text sequences is a challenging task. Previous work either
implicitly enforced the representations learnt from these two
modalities to be aligned in the latent space through multi-
tasking and parameter sharing or explicitly through conversion
of modalities via speech synthesis. While the former suffers
from interference between the two modalities, the latter
introduces additional complexity. In this paper, we propose
Maestro, a novel algorithm to learn unified representations from
both these modalities simultaneously that can transfer to diverse
downstream tasks such as Automated Speech Recognition
(ASR) and Speech Translation (ST). Maestro learns unified
representations through sequence alignment, duration predic-
tion and matching embeddings in the learned space through
an aligned masked-language model loss. We establish a new
state-of-the-art (SOTA) on VoxPopuli multilingual ASR with
a 8% relative reduction in Word Error Rate (WER), multi-
domain SpeechStew ASR (3.7% relative) and 21 languages to
English multilingual ST on CoVoST 2 with an improvement of
2.8 BLEU averaged over 21 languages.
View details
Accented Speech Recognition: Benchmarking, Pre-training, and Diverse Data
Zhehuai Chen
Chung-Cheng Chiu
Pavel Golik
Wei Han
Levi King
Suzan Schwartz
(2022)
Preview abstract
Building inclusive speech recognition systems is a crucial step towards developing technologies that speakers of all language varieties can use. Therefore, ASR systems must work for everybody independently of the way they speak. To accomplish this goal, there should be available data sets representing language varieties, and also an understanding of model configuration that is the most helpful in achieving robust understanding of all types of speech. However, there are not enough data sets for accented speech, and for the ones that are already available, more training approaches need to be explored to improve the quality of accented speech recognition. In this paper, we discuss recent progress towards developing more inclusive ASR systems, namely, the importance of building new data sets representing linguistic diversity, and exploring novel training approaches to improve performance for all users. We address recent directions within benchmarking ASR systems for accented speech, measure the effects of wav2vec 2.0 pre-training on accented speech recognition, and highlight corpora relevant for diverse ASR evaluations.
View details
Maestro-U: Leveraging joint speech-text representation learning for zero supervised speech ASR
Zhehuai Chen
Yu Zhang
Pedro Moreno Mengibar
IEEE SLT (2022)
Preview abstract
Training state-of-the-art Automated Speech Recognition (ASR) models typically requires a substantial amount of transcribed speech. In this work, we demonstrate that a modality-matched joint speech and text model introduced in~\cite{zhehuai2021} can be leveraged to train a massively multilingual ASR model without any transcribed speech. In most zero resource conditions, lack of transcribed speech also implies lack of lexicons. This paper explores the use of jointly learnt speech and text representations in a massively multilingual, zero transcribed speech, real-world setting to expand the set of languages covered by ASR models with only unlabeled speech and text in the target languages. We define the task to cover $102$ languages, where transcribed speech is available in $52$ of these languages and can be used to improve end-to-end ASR quality on the remaining $50$. First, we show that by combining speech representations with byte-level text representations coupled with the effective use of language embeddings, we can dramatically reduce the resource requirements for deploying an ASR model to a new language. On the FLEURS dataset, this approach is able to reduce the CER on languages with no transcribed speech from 64.1\% to 29.6\%, a relative reduction of 54\%. Second, using a subset of Indic languages we show that the proposed method can learn effectively from languages with transcribed speech even when there is limited to no graphemeic overlap with the target languages, reducing the average CER of the target languages from 56.3 to 17.2. We believe this is the first demonstration that competitive ASR performance can be achieved for an unseen language using no language resources other than text and untranscribed speech.
View details
Ask2Mask: Guided Data Selection for Masked Speech Modeling
Pedro Jose Moreno Mengibar
Yu Zhang
IEEE Journal of Selected Topics in Signal Processing (2022)
Preview abstract
Masked speech modeling (MSM) pre-training methods such as wav2vec2 or w2v-BERT randomly mask speech frames in an utterance and compute losses on the masked instances. While these methods improve performance of Automated Speech Recognition (ASR) systems, they have one major limitation. They generally perform best under matched conditions, i.e., when the data used for pre-training is matched to the data used for fine-tuning. Using out-of-domain (OOD) pre-training data with limited in-domain fine-tuning data from the target domain results in reduced gains. The relative value of in-domain data within a MSM pre-training corpus has not been well-explored in the literature. In this work, we address precisely this limitation. We propose ask2mask, a novel approach to focus on samples relevant to the target domain (in-domain) during pre-training with OOD or any available data. To perform this fine-grained data selection, ATM applies masking only to input frames with high confidence scores obtained from an external classification model. This allows the model to achieve meaningful in-domain representations and simultaneously discard low-confidence frames which could lead to learning erroneous representations. The ATM approach is further extended to focus on utterances with high confidences by scaling the final MSM loss computed for each masked input frame with the utterance-level confidence score. We conduct experiments on two well-benchmarked read speech corpus (Librispeech) and conversational speech corpus (AMI). The results substantiate the efficacy of ATM on significantly improving target domain performance under mismatched conditions while still yielding modest improvements under matched conditions.
View details
Extending Parrotron: An End-to-End, Speech Conversion and Speech Recognition Model for Atypical Speech
Rohan Doshi
Youzheng Chen
Liyang Jiang
Xia Zhang
Andrea Chu
Pedro Jose Moreno Mengibar
ICASSP 2021 - 2021 IEEE International Conference on Acoustics, Speech and Signal Processing (ICASSP)
Preview abstract
We present an extended Parrotron model: a single, end-to-end model that enables voice conversion and recognition simultaneously. Input spectrograms are transformed to output spectrograms in the voice of a predetermined target speaker while also generating hypotheses in the target vocabulary. We study the performance of this novel architecture that jointly predicts speech and text on atypical (‘dysarthric’) speech. We show that with as little as an hour of atypical speech, speaker adaptation can yield up to 67% relative reduction in Word Error Rate (WER). We also show that data augmentation using a customized synthesizer built on the atypical speech can provide an additional 10% relative improvement over the best speaker-adapted model. Finally, we show that these methods generalize across 8 dysarthria etiologies with a range of severities.
View details
Semi-Supervision in ASR: Sequential Mixmatch and Factorized TTS-Based Augmentation
Zhehuai Chen
Yu Zhang
Yinghui Huang
Jesse Emond
Pedro Jose Moreno Mengibar
(2021)
Preview abstract
Semi- and self-supervised training techniques have the potential to improve performance of speech recognition systems without additional transcribed speech data. In this work, we demonstrate the efficacy of two approaches to semi-supervision for automated speech recognition. The two approaches lever-age vast amounts of available unspoken text and untranscribed audio. First, we present factorized multilingual speech synthesis to improve data augmentation on unspoken text. Next, we present an online implementation of Noisy Student Training to incorporate untranscribed audio. We propose a modified Sequential MixMatch algorithm with iterative learning to learn from untranscribed speech. We demonstrate the compatibility of these techniques yielding a relative reduction of word error rate of up to 14.4% on the voice search task.
View details
Improving Speech Recognition Using Consistent Predictions on Synthesized Speech
Zhehuai Chen
Yu Zhang
Pedro Jose Moreno Mengibar
IEEE ICASSP 2020
Preview abstract
Speech synthesis has advanced to the point of being close to indistinguishable from human speech. However, efforts to train speech recognition systems on synthesized utterances have not been able to show that synthesized data can be effectively used to augment or replace human speech.
In this work, we demonstrate that promoting consistent predictions in response to real and synthesized speech enables significantly improved speech recognition performance.
We also find that training on 460 hours of LibriSpeech augmented with 500 hours of transcripts (without audio) performance is within 0.2\% WER of a system trained on 960 hours of transcribed audio. This suggests that with this approach, when there is sufficient text available, reliance on transcribed audio can be cut nearly in half.
View details
Generating diverse and natural text-to-speech samples using quantized fine-grained VAE and autoregressive prosody prior
Guangzhi Sun
Yu Zhang
ICASSP (2020)
Preview abstract
Recently proposed approaches for fine-grained prosody control of end-to-end text-to-speech samples enable precise control of the prosody of synthesized speech.
Such models incorporate a fine-grained variational autoencoder (VAE) structure into a sequence-to-sequence model, extracting latent prosody features for each input token (e.g.\ phonemes).
Generating samples using the standard VAE prior, an independent Gaussian at each time step, results in very unnatural and discontinuous speech, with dramatic variation between phonemes.
In this paper we propose a sequential prior in the discrete latent space which can be used to generate more natural samples.
This is accomplished by discretizing the latent prosody features using vector quantization, and training an autoregressive (AR) prior model over the result.
The AR prior is learned separately from the training of the posterior.
We evaluate the approach using subjective listening tests, objective metrics of automatic speech recognition (ASR) performance, as well as measurements of prosody attributes including volume, pitch, and phoneme duration.
Compared to the fine-grained VAE baseline, the proposed model achieves equally good copy synthesis reconstruction performance, but significantly improves naturalness in sample generation.
The diversity of the prosody in random samples better matches that of the real speech.
Furthermore, initial experiments demonstrate that samples generated from the quantized latent sapce can be used as an effective data augmentation strategy to improve ASR performance.
View details
SCADA: Stochastic, Consistent and Adversarial Data Augmentation to Improve ASR
Zhehuai Chen
Yu Zhang
Pedro Moreno
Proceedings of Interspeech 2020, pp. 2832-2836
Preview abstract
Recent developments in data augmentation has brought great gains in improvement for automatic speech recognition (ASR). Parallel developments in augmentation policy search in computer vision domain has shown improvements in model performance and robustness. In addition, recent developments in semi-supervised learning has shown that consistency measures are crucial for performance and robustness. In this work, we demonstrate that combining augmentation policies with consistency measures and model regularization can greatly improve speech recognition performance. Using the Librispeech task, we show: 1) symmetric consistency measures such as the Jensen-Shannon Divergence provide 11\% relative improvements in ASR performance; 2) Augmented adversarial inputs using Virtual Adversarial Noise (VAT) provides 8.9\% relative win; and 3) random sampling from arbitrary combination of augmentation policies yields the best policy. These contributions result in an overall reduction in Word Error Rate (WER) of 18\% relative on the Librispeech task presented in this paper.
View details
Improving Speech Recognition using GAN-based Speech Synthesis and Contrastive Unspoken Text Selection
Zhehuai Chen
Yu Zhang
Pedro Jose Moreno Mengibar
Interspeech 2020
Preview abstract
Text-to-Speech synthesis (TTS) based data augmentation is a relatively new mechanism for utilizing text-only data to improve automatic speech recognition (ASR) training without parameter or inference architecture changes. However, efforts to train speech recognition systems on synthesized utterances suffer from limited acoustic diversity of TTS outputs. Additionally, the text-only corpus is always much larger than the transcribed speech corpus by several orders of magnitude, which makes speech synthesis of all the text data impractical. In this work, we propose to combine generative adversarial network (GAN) and multi-style training (MTR) to increase acoustic diversity in the synthesized data. We also present a contrastive language model-based data selection technique to improve the efficiency of learning from unspoken text. We demonstrate the ability of our proposed method to enable efficient, large-scale unspoken text learning which achieving a 32.7\% relative WER reduction on a voice-search task.
View details
Speech Recognition with Augmented Synthesized Speech
Pedro Moreno
Ye Jia
Yu Zhang
ASRU 2019 (to appear)
Preview abstract
Recent success of the Tacotron speech synthesis architecture and its variants in producing natural sounding multi-speaker synthesized speech has raised the exciting possibility of replacing expensive, manually transcribed, domain-specific, human speech that is used to train speech recognizers. The multi-speaker speech synthesis architecture can learn latent embedding spaces of prosody, speaker and style variations derived from input acoustic representations thereby allowing for manipulation of the synthesized speech. In this paper, we evaluate the feasibility of enhancing speech recognition performance using speech synthesis using two corpora from different domains. We explore algorithms to provide the necessary acoustic and lexical diversity needed for robust speech recognition. Finally, we demonstrate the feasibility of this approach as a data augmentation strategy for domain-transfer.
View details
Learning to speak fluently in a foreign language: Multilingual speech synthesis and cross-language voice cloning
Yu Zhang
Ye Jia
Interspeech (2019)
Preview abstract
We present a multispeaker, multilingual text-to-speech (TTS) synthesis model based on Tacotron that is able to produce high quality speech in multiple languages. Moreover, the model is able to transfer voices across languages, e.g. synthesize fluent Spanish speech using an English speaker's voice, without training on any bilingual or parallel examples. Such transfer works across distantly related languages, e.g. English and Mandarin.
Critical to achieving this result are: 1. using a phonemic input representation to encourage sharing of model capacity across languages, and 2. incorporating an adversarial loss term to encourage the model to disentangle its representation of speaker identity (which is perfectly correlated with language in the training data) from the speech content. Further scaling up the model by training on multiple speakers of each language, and incorporating an autoencoding input to help stabilize attention during training, results in a model which can be used to consistently synthesize intelligible speech for training speakers in all languages seen during training, and in native or foreign accents.
View details
No Results Found