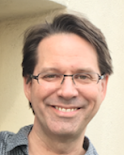
Brian Curless
Research Areas
Authored Publications
Google Publications
Other Publications
Sort By
Preview abstract
We present FederNeRF, a method that takes a collection of photos of a subject (e.g. Roger Federer) captured across multiple years with arbitrary body poses and appearances, and enables rendering the subject with arbitrary novel combinations of viewpoint, body pose, and appearance. NeRFederer builds a customized neural volumetric 3D model of the subject that is able to render an entire space spanned by camera viewpoint, body pose, and appearance. A central challenge in this task is dealing with sparse observations; a given body pose is likely only observed by a single viewpoint with a single appearance, and a given appearance is only observed under a handful of different body poses. We address this issue by recovering a canonical T-pose neural volumetric representation of the subject that allows for changing appearance across different observations, but uses a shared pose-dependent motion field across all observations. We demonstrate that this approach, along with regularization of the recovered volumetric geometry to encourage smoothness, is able to recover a model that renders compelling images from novel combinations of viewpoint, pose, and appearance from these challenging unstructured photo collections, outperforming prior work for free-viewpoint human rendering.
View details
HumanNeRF: Free-viewpoint Rendering of Moving People from Monocular Video
Chung-Yi Weng
Pratul Srinivasan
CVPR (Computer Vision and Pattern Recognition), IEEE and the Computer Vision Foundation (2022) (to appear)
Preview abstract
We introduce a free-viewpoint rendering method -- HumanNeRF -- that works on a given monocular video of a human performing complex body motions, e.g. a video from YouTube. Our method enables pausing the video at any frame and rendering the subject from arbitrary new camera viewpoints or even a full 360-degree camera path for that particular frame and body pose. This task is particularly challenging, as it requires synthesizing photorealistic details of the body, as seen from various camera angles that may not exist in the input video, as well as synthesizing fine details such as cloth folds and facial appearance. Our method optimizes for a volumetric representation of the person in a canonical T-pose, in concert with a motion field that maps the estimated canonical representation to every frame of the video via backward warps. The motion field is decomposed into skeletal rigid and non-rigid motions, produced by deep networks. We show significant performance improvements over prior work, and compelling examples of free-viewpoint renderings from monocular video of moving humans in challenging uncontrolled capture scenarios.
View details
3D Moments from Near Duplicate Photos
Qianqian Wang
Zhengqi Li
Conference on Computer Vision and Pattern Recognition (CVPR) (2022)
Preview abstract
We introduce a new computational photography effect, starting from a pair of near duplicate photos that are prevalent in people's photostreams. Combining monocular depth synthesis and optical flow, we build a novel end-to-end system that can interpolate scene motion while simultaneously allowing independent control of the camera. We use our system to create short videos with scene motion and cinematic camera motion. We compare our method against two different baselines and demonstrate that our system outperforms them both qualitatively and quantitatively in publicly available benchmark datasets.
View details
FILM: Frame Interpolation for Large Motion
Fitsum Reda
Eric Tabellion
Proceedings of the European conference on computer vision (ECCV) (2022)
Preview abstract
We present a frame interpolation algorithm that synthesizes
an engaging slow-motion video from near-duplicate photos which often
exhibit large scene motion. Near-duplicates interpolation is an interesting
new application, but large motion poses challenges to existing methods.
To address this issue, we adapt a feature extractor that shares weights
across the scales, and present a “scale-agnostic” motion estimator. It
relies on the intuition that large motion at finer scales should be similar
to small motion at coarser scales, which boosts the number of available
pixels for large motion supervision. To inpaint wide disocclusions caused
by large motion and synthesize crisp frames, we propose to optimize
our network with the Gram matrix loss that measures the correlation
difference between features. To simplify the training process, we further
propose a unified single-network approach that removes the reliance on
additional optical-flow or depth network and is trainable from frame
triplets alone. Our approach outperforms state-of-the-art methods on
the Xiph large motion benchmark while performing favorably on Vimeo90K, Middlebury and UCF101. Source codes and pre-trained models are
available at https://film-net.github.io.
View details
SLIDE: Single Image 3D Photography with Soft Layering and Depth-aware Inpainting
Varun Jampani*
Huiwen Chang*
Kyle Gregory Sargent
Dominik Philemon Kaeser
Ce Liu
ICCV 2021 (2021)
Preview abstract
Single image 3D photography enables viewers to view a still image from novel viewpoints. Recent approaches for single-image view synthesis combine monocular depth network along with inpainting networks resulting in compelling novel view synthesis results. A drawback of these approaches is the use of hard layering making them not suitable to model intricate appearance effects such as matting. We present SLIDE, a modular and unified system for single image 3D photography that uses simple yet effective soft layering strategy to model appearance effects. In addition, we propose a novel depth-aware training of inpainting network suitable for 3D photography task. Extensive experimental analysis on 3 different view synthesis datasets in combination with user studies on in-the-wild image collections demonstrate the superior performance of our technique in comparison to existing strong baselines.
View details
Removing an object and its shadows from a photograph
Edward Zhang
Ricardo Martin-Brualla
IEEE/CVF Conference on Computer Vision and Pattern Recognition (CVPR) (2021)
Preview abstract
Removing objects from images is an important problem for many applications, such as in mixed reality. For believable removals, the shadows that the object casts onto the scene should also be removed. Current inpainting-based methods for object removal do not consider shadows, or at best require manually adding shadow regions to the inpainting mask. We introduce a deep learning pipeline for removing a shadow along with its caster. We leverage rough scene models in order to remove a wide variety of shadows (hard or soft, dark or subtle, large or thin) from planar surfaces with a wide variety of surface textures. We train our pipeline on synthetically rendered data, and show qualitative and quantitative results on both synthetic and real scenes.
View details
Monster Mash: A Single-View Approach to Casual 3D Modeling and Animation
Marek Dvoroznak
Olga Sorkine-Hornung
ACM Transactions on Graphics (TOG), ACM, New York, NY, USA (2020), pp. 1-12 (to appear)
Preview abstract
We present a new framework for sketch-based modeling and animation of 3D organic shapes that can work entirely in an intuitive 2D domain, enabling a playful, casual experience. Unlike previous sketch-based tools, our approach does not require a tedious part-based multi-view workflow with the explicit specification of an animation rig. Instead, we combine 3D inflation with a novel rigidity-preserving, layered deformation model, ARAP-L, to produce a smooth 3D mesh that is immediately ready for animation. Moreover, the resulting model can be animated from a single viewpoint — and without the need to handle unwanted inter-penetrations, as required by previous approaches. We demonstrate the benefit of our approach on a variety of examples produced by inexperienced users as well as professional animators. For less experienced users, our single-view approach offers a simpler modeling and animating experience than working in a 3D environment, while for professionals, it offers a quick and casual workspace for ideation.
View details
No Results Found