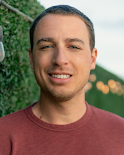
Alexander Wakim
Alex is a Data Scientist who works on statistical methodology for Google measurement products.
Research Areas
Authored Publications
Google Publications
Other Publications
Sort By
Media Mix Model Calibration With Bayesian Priors
Mike Wurm
Brenda Price
Ying Liu
research.google (2024)
Preview abstract
Effective model calibration is a critical and indispensable component in developing Media Mix Models (MMMs). One advantage of Bayesian-based MMMs lies in their capacity to accommodate the information from experiment results and the modelers' domain knowledge about the ad effectiveness by setting priors for the model parameters. However, it remains ambiguous about how and which Bayesian priors should be tuned for calibration purpose. In this paper, we propose a new calibration method through model reparameterization. The reparameterized model includes Return on Ads Spend (ROAS) as a model parameter, enabling straightforward adjustment of its prior distribution to align with either experiment results or the modeler's prior knowledge. The proposed method also helps address several key challenges regarding combining MMMs and incrementality experiments. We use simulations to demonstrate that our approach can significantly reduce the bias and uncertainty in the resultant posterior ROAS estimates.
View details
Preview abstract
Reach and frequency (R&F) is a core lever in the execution of ad campaigns, but it is not widely captured in the marketing mix models (MMMs) being fitted today due to the unavailability of accurate R&F metrics for some traditional media channels. Current practice usually uses impressions aggregated at regional level as inputs for MMMs, which does not take into account the fact that individuals can be exposed to an advertisement multiple times, and that the impact of an advertisement on an individual can change based on the number of times they are exposed. To address this limitation, we propose a R&F MMM which is an extension to Geo-level Bayesian Hierarchical Media Mix Modeling (GBHMMM) and is applicable when R&F data is available for at least one media channel. By incorporating R&F into MMM models, the new methodology is shown to produce more accurate estimates of the impact of marketing on business outcomes, and helps users optimize their campaign execution based on optimal frequency recommendations.
View details
No Results Found