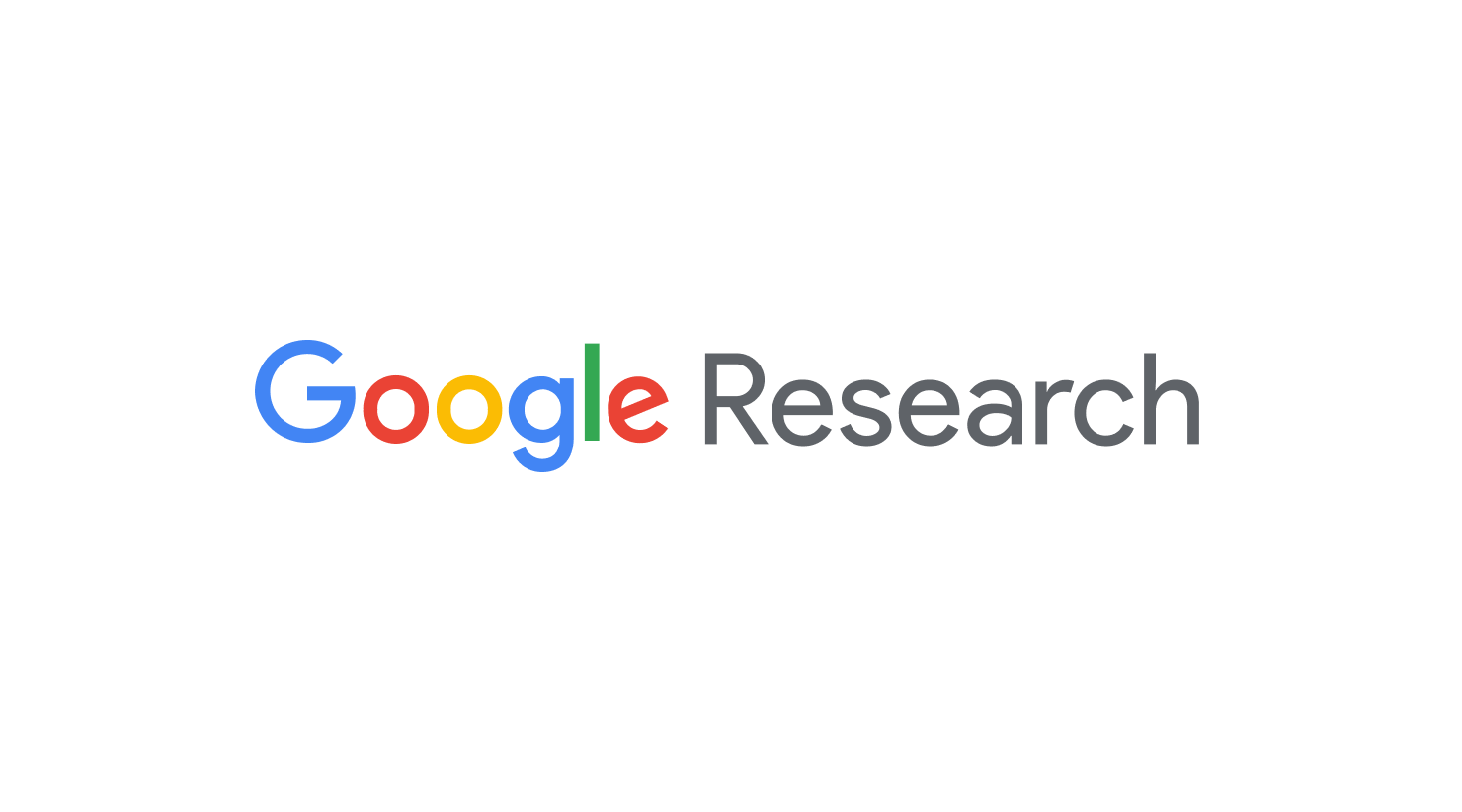
Google at ICML 2020
July 13, 2020
Posted by Jaqui Herman and Cat Armato, Program Managers
Machine learning is a key strategic focus at Google, with highly active groups pursuing research in virtually all aspects of the field, including deep learning and more classical algorithms, exploring theory as well as application. We utilize scalable tools and architectures to build machine learning systems that enable us to solve deep scientific and engineering challenges in areas of language, speech, translation, music, visual processing and more.
As a leader in machine learning research, Google is proud to be a Platinum Sponsor of the thirty-seventh International Conference on Machine Learning (ICML 2020), a premier annual event taking place virtually this week. With over 100 accepted publications and Googlers participating in workshops, we look forward to our continued collaboration with the larger machine learning research community.
If you're registered for ICML 2020, we hope you'll visit the Google virtual booth to learn more about the exciting work, creativity and fun that goes into solving some of the field's most interesting challenges. You can also learn more about the Google research being presented at ICML 2020 in the list below (Google affiliations bolded).
ICML Expo
Google Dataset Search: Building an Open Ecosystem for Dataset Discovery
Natasha Noy
End-to-end Bayesian inference workflows in TensorFlow Probability
Colin Carroll
Publications
Population-Based Black-Box Optimization for Biological Sequence Design
Christof Angermueller, David Belanger, Andreea Gane, Zelda Mariet, David Dohan, Kevin Murphy, Lucy Colwell, D Sculley
Predictive Coding for Locally-Linear Control
Rui Shu, Tung Nguyen, Yinlam Chow, Tuan Pham, Khoat Than, Mohammad Ghavamzadeh, Stefano Ermon, Hung Bui
FedBoost: A Communication-Efficient Algorithm for Federated Learning
Jenny Hamer, Mehryar Mohri, Ananda Theertha Suresh
Faster Graph Embeddings via Coarsening
Matthew Fahrbach, Gramoz Goranci, Richard Peng, Sushant Sachdeva, Chi Wang
Revisiting Fundamentals of Experience Replay
William Fedus, Prajit Ramachandran, Rishabh Agarwal, Yoshua Bengio, Hugo Larochelle, Mark Rowland, Will Dabney
Boosting for Control of Dynamical Systems
Naman Agarwal, Nataly Brukhim, Elad Hazan, Zhou Lu
Neural Clustering Processes
Ari Pakman, Yueqi Wang, Catalin Mitelut, JinHyung Lee, Liam Paninski
The Tree Ensemble Layer: Differentiability Meets Conditional Computation
Hussein Hazimeh, Natalia Ponomareva, Petros Mol, Zhenyu Tan, Rahul Mazumder
Representations for Stable Off-Policy Reinforcement Learning
Dibya Ghosh, Marc Bellemare
REALM: Retrieval-Augmented Language Model Pre-Training
Kelvin Guu, Kenton Lee, Zora Tung, Panupong Pasupat, Ming-Wei Chang
Context Aware Local Differential Privacy
Jayadev Acharya, Keith Bonawitz, Peter Kairouz, Daniel Ramage, Ziteng Sun
Scalable Deep Generative Modeling for Sparse Graphs
Hanjun Dai, Azade Nazi, Yujia Li, Bo Dai, Dale Schuurmans
Deep k-NN for Noisy Labels
Dara Bahri, Heinrich Jiang, Maya Gupta†
Revisiting Spatial Invariance with Low-Rank Local Connectivity
Gamaleldin F. Elsayed, Prajit Ramachandran, Jonathon Shlens, Simon Kornblith
SCAFFOLD: Stochastic Controlled Averaging for Federated Learning
Sai Praneeth Karimireddy, Satyen Kale, Mehryar Mohri, Sashank J. Reddi, Sebastian U. Stich, Ananda Theertha Suresh
Incremental Sampling Without Replacement for Sequence Models
Kensen Shi, David Bieber, Charles Sutton
SoftSort: A Continuous Relaxation for the argsort Operator
Sebastian Prillo, Julian Martin Eisenschlos
XTREME: A Massively Multilingual Multi-task Benchmark for Evaluating Cross-lingual Generalisation (see blog post)
Junjie Hu, Sebastian Ruder, Aditya Siddhant, Graham Neubig, Orhan Firat, Melvin Johnson
Learning to Stop While Learning to Predict
Xinshi Chen, Hanjun Dai, Yu Li, Xin Gao, Le Song
Bandits with Adversarial Scaling
Thodoris Lykouris, Vahab Mirrokni, Renato Paes Leme
SimGANs: Simulator-Based Generative Adversarial Networks for ECG Synthesis to Improve Deep ECG Classification
Tomer Golany, Daniel Freedman, Kira Radinsky
Stochastic Frank-Wolfe for Constrained Finite-Sum Minimization
Geoffrey Negiar, Gideon Dresdner, Alicia Yi-Ting Tsai, Laurent El Ghaoui, Francesco Locatello, Robert M. Freund, Fabian Pedregosa
Implicit differentiation of Lasso-type models for hyperparameter optimization
Quentin Bertrand, Quentin Klopfenstein, Mathieu Blondel, Samuel Vaiter, Alexandre Gramfort, Joseph Salmon
Infinite attention: NNGP and NTK for deep attention networks
Jiri Hron, Yasaman Bahri, Jascha Sohl-Dickstein, Roman Novak
Logarithmic Regret for Learning Linear Quadratic Regulators Efficiently
Asaf Cassel, Alon Cohen, Tomer Koren
Adversarial Learning Guarantees for Linear Hypotheses and Neural Networks
Pranjal Awasthi, Natalie Frank, Mehryar Mohri
Random Hypervolume Scalarizations for Provable Multi-Objective Black Box Optimization
Daniel Golovin, Qiuyi (Richard) Zhang
Generating Programmatic Referring Expressions via Program Synthesis
Jiani Huang, Calvin Smith, Osbert Bastani, Rishabh Singh, Aws Albarghouthi, Mayur Naik
Optimizing Long-term Social Welfare in Recommender Systems: A Constrained Matching Approach
Martin Mladenov, Elliot Creager, Omer Ben-Porat, Kevin Swersky, Richard Zemel, Craig Boutilier
AutoML-Zero: Evolving Machine Learning Algorithms From Scratch (see blog post)
Esteban Real, Chen Liang, David R. So, Quoc V. Le
How Good is the Bayes Posterior in Deep Neural Networks Really?
Florian Wenzel, Kevin Roth, Bastiaan S. Veeling, Jakub Swiatkowski, Linh Tran, Stephan Mandt, Jasper Snoek, Tim Salimans, Rodolphe Jenatton, Sebastian Nowozin†
Which Tasks Should Be Learned Together in Multi-task Learning?
Trevor Standley, Amir R. Zamir, Dawn Chen, Leonidas Guibas, Jitendra Malik, Silvio Savarese
Influence Diagram Bandits: Variational Thompson Sampling for Structured Bandit Problems
Tong Yu, Branislav Kveton, Zheng Wen, Ruiyi Zhang, Ole J. Mengshoel
Disentangling Trainability and Generalization in Deep Neural Networks
Lechao Xiao, Jeffrey Pennington, Samuel S. Schoenholz
The Many Shapley Values for Model Explanation
Mukund Sundararajan, Amir Najmi
Neural Contextual Bandits with UCB-based Exploration
Dongruo Zhou, Lihong Li, Quanquan Gu
Automatic Shortcut Removal for Self-Supervised Representation Learning
Matthias Minderer, Olivier Bachem, Neil Houlsby, Michael Tschannen
Federated Learning with Only Positive Labels
Felix X. Yu, Ankit Singh Rawat, Aditya Krishna Menon, Sanjiv Kumar
How Recurrent Networks Implement Contextual Processing in Sentiment Analysis
Niru Maheswaranathan, David Sussillo
Supervised Learning: No Loss No Cry
Richard Nock, Aditya Krishna Menon
Ready Policy One: World Building Through Active Learning
Philip Ball, Jack Parker-Holder, Aldo Pacchiano, Krzysztof Choromanski, Stephen Roberts
Weakly-Supervised Disentanglement Without Compromises
Francesco Locatello, Ben Poole, Gunnar Raetsch, Bernhard Schölkopf, Olivier Bachem, Michael Tschannen
Fast Differentiable Sorting and Ranking
Mathieu Blondel, Olivier Teboul, Quentin Berthet, Josip Djolonga
Debiased Sinkhorn barycenters
Hicham Janati, Marco Cuturi, Alexandre Gramfort
Interpretable, Multidimensional, Multimodal Anomaly Detection with Negative Sampling for Detection of Device Failure
John Sipple
Accelerating Large-Scale Inference with Anisotropic Vector Quantization
Ruiqi Guo, Philip Sun, Erik Lindgren, Quan Geng†, David Simcha, Felix Chern, Sanjiv Kumar
An Optimistic Perspective on Offline Reinforcement Learning (see blog post)
Rishabh Agarwal, Dale Schuurmans, Mohammad Norouzi
The Neural Tangent Kernel in High Dimensions: Triple Descent and a Multi-Scale Theory of Generalization
Ben Adlam, Jeffrey Pennington
Private Query Release Assisted by Public Data
Raef Bassily, Albert Cheu, Shay Moran, Aleksandar Nikolov, Jonathan Ullman, Zhiwei Steven Wu
Learning and Evaluating Contextual Embedding of Source Code
Aditya Kanade, Petros Maniatis, Gogul Balakrishnan, Kensen Shi
Evaluating Machine Accuracy on ImageNet
Vaishaal Shankar, Rebecca Roelofs, Horia Mania, Alex Fang, Benjamin Recht, Ludwig Schmidt
Imputer: Sequence Modelling via Imputation and Dynamic Programming
William Chan, Chitwan Saharia, Geoffrey Hinton, Mohammad Norouzi, Navdeep Jaitly
Domain Aggregation Networks for Multi-Source Domain Adaptation
Junfeng Wen, Russell Greiner, Dale Schuurmans
Planning to Explore via Self-Supervised World Models
Ramanan Sekar, Oleh Rybkin, Kostas Daniilidis, Pieter Abbeel, Danijar Hafner, Deepak Pathak
Context-Aware Dynamics Model for Generalization in Model-Based Reinforcement Learning
Kimin Lee, Younggyo Seo, Seunghyun Lee, Honglak Lee, Jinwoo Shin
Retro*: Learning Retrosynthetic Planning with Neural Guided A* Search
Binghong Chen, Chengtao Li, Hanjun Dai, Le Song
On the Consistency of Top-k Surrogate Losses
Forest Yang, Sanmi Koyejo
Dual Mirror Descent for Online Allocation Problems
Haihao Lu, Santiago Balseiro, Vahab Mirrokni
Efficient and Scalable Bayesian Neural Nets with Rank-1 Factors
Michael W. Dusenberry, Ghassen Jerfel, Yeming Wen, Yi-An Ma†, Jasper Snoek, Katherine Heller, Balaji Lakshminarayanan, Dustin Tran
Batch Stationary Distribution Estimation
Junfeng Wen, Bo Dai, Lihong Li, Dale Schuurmans
Small-GAN: Speeding Up GAN Training Using Core-Sets
Samarth Sinha, Han Zhang, Anirudh Goyal, Yoshua Bengio, Hugo Larochelle, Augustus Odena
Data Valuation Using Reinforcement Learning
Jinsung Yoon, Sercan Ö. Arik, Tomas Pfister
A Game Theoretic Perspective on Model-Based Reinforcement Learning
Aravind Rajeswaran, Igor Mordatch, Vikash Kumar
Encoding Musical Style with Transformer Autoencoders
Kristy Choi, Curtis Hawthorne, Ian Simon, Monica Dinculescu, Jesse Engel
The Shapley Taylor Interaction Index
Kedar Dhamdhere, Mukund Sundararajan, Ashish Agarwal
Multidimensional Shape Constraints
Maya Gupta†, Erez Louidor, Olexander Mangylov†, Nobu Morioka, Taman Narayan, Sen Zhao
Private Counting from Anonymous Messages: Near-Optimal Accuracy with Vanishing Communication Overhead
Badih Ghazi, Ravi Kumar, Pasin Manurangsi, Rasmus Pagh
Learning to Score Behaviors for Guided Policy Optimization
Aldo Pacchiano, Jack Parker-Holder, Yunhao Tang, Anna Choromanska, Krzysztof Choromanski, Michael I. Jordan
Fundamental Tradeoffs between Invariance and Sensitivity to Adversarial Perturbations
Florian Tramèr, Jens Behrmann, Nicholas Carlini, Nicolas Papernot, Jörn-Henrik Jacobsen
Optimizing Black-Box Metrics with Adaptive Surrogates
Qijia Jiang, Olaoluwa Adigun, Harikrishna Narasimhan, Mahdi Milani Fard, Maya Gupta†
Circuit-Based Intrinsic Methods to Detect Overfitting
Sat Chatterjee, Alan Mishchenko
Automatic Reparameterisation of Probabilistic Programs
Maria I. Gorinova, Dave Moore, Matthew D. Hoffman
Stochastic Flows and Geometric Optimization on the Orthogonal Group
Krzysztof Choromanski, David Cheikhi, Jared Davis, Valerii Likhosherstov, Achille Nazaret, Achraf Bahamou, Xingyou Song, Mrugank Akarte, Jack Parker-Holder, Jacob Bergquist, Yuan Gao, Aldo Pacchiano, Tamas Sarlos, Adrian Weller, Vikas Sindhwani
Black-Box Variational Inference as a Parametric Approximation to Langevin Dynamics
Matthew Hoffman, Yi-An Ma†
Concise Explanations of Neural Networks Using Adversarial Training
Prasad Chalasani, Jiefeng Chen, Amrita Roy Chowdhury, Somesh Jha, Xi Wu
p-Norm Flow Diffusion for Local Graph Clustering
Shenghao Yang, Di Wang, Kimon Fountoulakis
Empirical Study of the Benefits of Overparameterization in Learning Latent Variable Models
Rares-Darius Buhai, Yoni Halpern, Yoon Kim, Andrej Risteski, David Sontag
Robust Pricing in Dynamic Mechanism Design
Yuan Deng, Sébastien Lahaie, Vahab Mirrokni
Differentiable Product Quantization for Learning Compact Embedding Layers
Ting Chen, Lala Li, Yizhou Sun
Adaptive Region-Based Active Learning
Corinna Cortes, Giulia DeSalvo, Claudio Gentile, Mehryar Mohri, Ningshan Zhang
Countering Language Drift with Seeded Iterated Learning
Yuchen Lu, Soumye Singhal, Florian Strub, Olivier Pietquin, Aaron Courville
Does Label Smoothing Mitigate Label Noise?
Michal Lukasik, Srinadh Bhojanapalli, Aditya Krishna Menon, Sanjiv Kumar
Acceleration Through Spectral Density Estimation
Fabian Pedregosa, Damien Scieur
Momentum Improves Normalized SGD
Ashok Cutkosky, Harsh Mehta
ConQUR: Mitigating Delusional Bias in Deep Q-Learning
Andy Su, Jayden Ooi, Tyler Lu, Dale Schuurmans, Craig Boutilier
Online Learning with Imperfect Hints
Aditya Bhaskara, Ashok Cutkosky, Ravi Kumar, Manish Purohit
Go Wide, Then Narrow: Efficient Training of Deep Thin Networks
Denny Zhou, Mao Ye, Chen Chen, Tianjian Meng, Mingxing Tan, Xiaodan Song, Quoc Le, Qiang Liu, Dale Schuurmans
On Implicit Regularization in β-VAEs
Abhishek Kumar, Ben Poole
Is Local SGD Better than Minibatch SGD?
Blake Woodworth, Kumar Kshitij Patel, Sebastian U. Stich, Zhen Dai, Brian Bullins, H. Brendan McMahan, Ohad Shamir, Nathan Sreb
A Simple Framework for Contrastive Learning of Visual Representations
Ting Chen, Simon Kornblith, Mohammad Norouzi, Geoffrey Hinton
Universal Average-Case Optimality of Polyak Momentum
Damien Scieur, Fabian Pedregosa
An Imitation Learning Approach for Cache Replacement
Evan Zheran Liu, Milad Hashemi, Kevin Swersky, Parthasarathy Ranganathan, Junwhan Ahn
Collapsed Amortized Variational Inference for Switching Nonlinear Dynamical Systems
Zhe Dong, Bryan A. Seybold, Kevin P. Murphy, Hung H. Bui
Beyond Synthetic Noise: Deep Learning on Controlled Noisy Labels
Lu Jiang, Di Huang, Mason Liu, Weilong Yang
Optimizing Data Usage via Differentiable Rewards
Xinyi Wang, Hieu Pham, Paul Michel, Antonios Anastasopoulos, Jaime Carbonell, Graham Neubig
Sparse Sinkhorn Attention
Yi Tay, Dara Bahri, Liu Yang, Donald Metzler, Da-Cheng Juan
One Policy to Control Them All: Shared Modular Policies for Agent-Agnostic Control
Wenlong Huang, Igor Mordatch, Deepak Pathak
On Thompson Sampling with Langevin Algorithms
Eric Mazumdar, Aldo Pacchiano, Yi-An Ma†, Peter L. Bartlett, Michael I. Jordan
Good Subnetworks Provably Exist: Pruning via Greedy Forward Selection
Mao Ye, Chengyue Gong, Lizhen Nie, Denny Zhou, Adam Klivans, Qiang Liu
On the Global Convergence Rates of Softmax Policy Gradient Methods
Jincheng Mei, Chenjun Xiao, Csaba Szepesvari, Dale Schuurmans
Concept Bottleneck Models
Pang Wei Koh, Thao Nguyen, Yew Siang Tang, Stephen Mussmann, Emma Pierson, Been Kim, Percy Liang
Supervised Quantile Normalization for Low-Rank Matrix Approximation
Marco Cuturi, Olivier Teboul, Jonathan Niles-Weed, Jean-Philippe Vert
Missing Data Imputation Using Optimal Transport
Boris Muzellec, Julie Josse, Claire Boyer, Marco Cuturi
Learning to Combine Top-Down and Bottom-Up Signals in Recurrent Neural Networks with Attention Over Modules
Sarthak Mittal, Alex Lamb, Anirudh Goyal, Vikram Voleti, Murray Shanahan, Guillaume Lajoie, Michael Mozer, Yoshua Bengio
Stochastic Optimization for Regularized Wasserstein Estimators
Marin Ballu, Quentin Berthet, Francis Bach
Low-Rank Bottleneck in Multi-head Attention Models
Srinadh Bhojanapalli, Chulhee Yun, Ankit Singh Rawat, Sashank Jakkam Reddi, Sanjiv Kumar
Rigging the Lottery: Making All Tickets Winners
Utku Evci, Trevor Gale, Jacob Menick, Pablo Samuel Castro, Erich Elsen
Online Learning with Dependent Stochastic Feedback Graphs
Corinna Cortes, Giulia DeSalvo, Claudio Gentile, Mehryar Mohri, Ningshan Zhang
Calibration, Entropy Rates, and Memory in Language Models
Mark Braverman, Xinyi Chen, Sham Kakade, Karthik Narasimhan, Cyril Zhang, Yi Zhang
Composable Sketches for Functions of Frequencies: Beyond the Worst Case
Edith Cohen, Ofir Geri, Rasmus Pagh
Energy-Based Processes for Exchangeable Data
Mengjiao Yang, Bo Dai, Hanjun Dai, Dale Schuurmans
Near-Optimal Regret Bounds for Stochastic Shortest Path
Alon Cohen, Haim Kaplan, Yishay Mansour, Aviv Rosenberg
PEGASUS: Pre-training with Extracted Gap-sentences for Abstractive Summarization (see blog post)
Jingqing Zhang, Yao Zhao, Mohammad Saleh, Peter J. Liu
The Complexity of Finding Stationary Points with Stochastic Gradient Descent
Yoel Drori, Ohad Shamir
The k-tied Normal Distribution: A Compact Parameterization of Gaussian Mean Field Posteriors in Bayesian Neural Networks
Jakub Swiatkowski, Kevin Roth, Bas Veeling, Linh Tran, Josh Dillon, Stephan Mandt, Jasper Snoek, Tim Salimans, Rodolphe Jenatton, Sebastian Nowozin†
Regularized Optimal Transport is Ground Cost Adversarial
François-Pierre Paty, Marco Cuturi
Workshops
New In ML
Invited Speaker: Nicolas Le Roux
Organizers: Zhen Xu, Sparkle Russell-Puleri, Zhengying Liu, Sinead A Williamson, Matthias W Seeger, Wei-Wei Tu, Samy Bengio, Isabelle Guyon
LatinX in AI
Workshop Advisor: Pablo Samuel Castro
Women in Machine Learning Un-Workshop
Invited Speaker: Doina Precup
Sponsor Expo Speaker: Jennifer Wei
Queer in AI
Invited Speaker: Shakir Mohamed
Workshop on Continual Learning
Organizers: Haytham Fayek, Arslan Chaudhry, David Lopez-Paz, Eugene Belilovsky, Jonathan Schwarz, Marc Pickett, Rahaf Aljundi, Sayna Ebrahimi, Razvan Pascanu, Puneet Dokania
5th ICML Workshop on Human Interpretability in Machine Learning (WHI)
Organizers: Kush Varshney, Adrian Weller, Alice Xiang, Amit Dhurandhar, Been Kim, Dennis Wei, Umang Bhatt
Self-supervision in Audio and Speech
Organizers: Mirco Ravanelli, Dmitriy Serdyuk, R Devon Hjelm, Bhuvana Ramabhadran, Titouan Parcollet
Workshop on eXtreme Classification: Theory and Applications
Invited Speakers: Sanjiv Kumar
Healthcare Systems, Population Health, and the Role of Health-tech
Organizers: Krzysztof Choromanski, David Cheikhi, Jared Davis, Valerii Likhosherstov, Achille Nazaret, Achraf Bahamou, Xingyou Song, Mrugank Akarte, Jack Parker-Holder, Jacob Bergquist, Yuan Gao, Aldo Pacchiano, Tamas Sarlos, Adrian Weller, Vikas Sindhwani
Theoretical Foundations of Reinforcement Learning
Program Committee: Alon Cohen, Chris Dann
Uncertainty and Robustness in Deep Learning Workshop (UDL)
Invited Speaker: Justin Gilmer
Organizers: Sharon Li, Balaji Lakshminarayanan, Dan Hendrycks, Thomas Dietterich, Jasper Snoek
Program Committee: Jeremiah Liu, Jie Ren, Rodolphe Jenatton, Zack Nado, Alexander Alemi, Florian Wenzel, Mike Dusenberry, Raphael Lopes
Beyond First Order Methods in Machine Learning Systems
Industry Panel: Jonathan Hseu
Object-Oriented Learning: Perception, Representation, and Reasoning
Invited Speakers: Thomas Kipf, Igor Mordatch
Graph Representation Learning and Beyond (GRL+)
Organizers: Michael Bronstein, Andreea Deac, William L. Hamilton, Jessica B. Hamrick, Milad Hashemi, Stefanie Jegelka, Jure Leskovec, Renjie Liao, Federico Monti, Yizhou Sun, Kevin Swersky, Petar Veličković, Rex Ying, Marinka Žitnik
Speakers: Thomas Kipf
Program Committee: Bryan Perozzi, Kevin Swersky, Milad Hashemi, Thomas Kipf, Ting Cheng
ML Interpretability for Scientific Discovery
Organizers: Subhashini Venugopalan, Michael Brenner, Scott Linderman, Been Kim
Program Committee: Akinori Mitani, Arunachalam Narayanaswamy, Avinash Varadarajan, Awa Dieng, Benjamin Sanchez-Lengeling, Bo Dai, Stephan Hoyer, Subham Sekhar Sahoo, Suhani Vora
Steering Committee: John Platt, Mukund Sundararajan, Jon Kleinberg
Negative Dependence and Submodularity for Machine Learning
Organizers: Zelda Mariet, Mike Gartrell, Michal Derezinski
7th ICML Workshop on Automated Machine Learning (AutoML)
Organizers: Charles Weill, Katharina Eggensperger, Matthias Feurer, Frank Hutter, Marius Lindauer, Joaquin Vanschoren
Federated Learning for User Privacy and Data Confidentiality
Keynote: Brendan McMahan
Program Committee: Peter Kairouz, Jakub Konecný
MLRetrospectives: A Venue for Self-Reflection in ML Research
Speaker: Margaret Mitchell
Machine Learning for Media Discovery
Speaker: Ed Chi
INNF+: Invertible Neural Networks, Normalizing Flows, and Explicit Likelihood Models
Organizers: Chin-Wei Huang, David Krueger, Rianne van den Berg, George Papamakarios, Chris Cremer, Ricky Chen, Danilo Rezende
4th Lifelong Learning Workshop
Program Committee: George Tucker, Marlos C. Machado
2nd ICML Workshop on Human in the Loop Learning (HILL)
Organizers: Shanghang Zhang, Xin Wang, Fisher Yu, Jiajun Wu, Trevor Darrell
Machine Learning for Global Health
Organizers: Danielle Belgrave, Danielle Belgrave, Stephanie Hyland, Charles Onu, Nicholas Furnham, Ernest Mwebaze, Neil Lawrence
Committee
Social Chair: Adam White
†Work performed while at Google
-
Labels:
- Conferences & Events