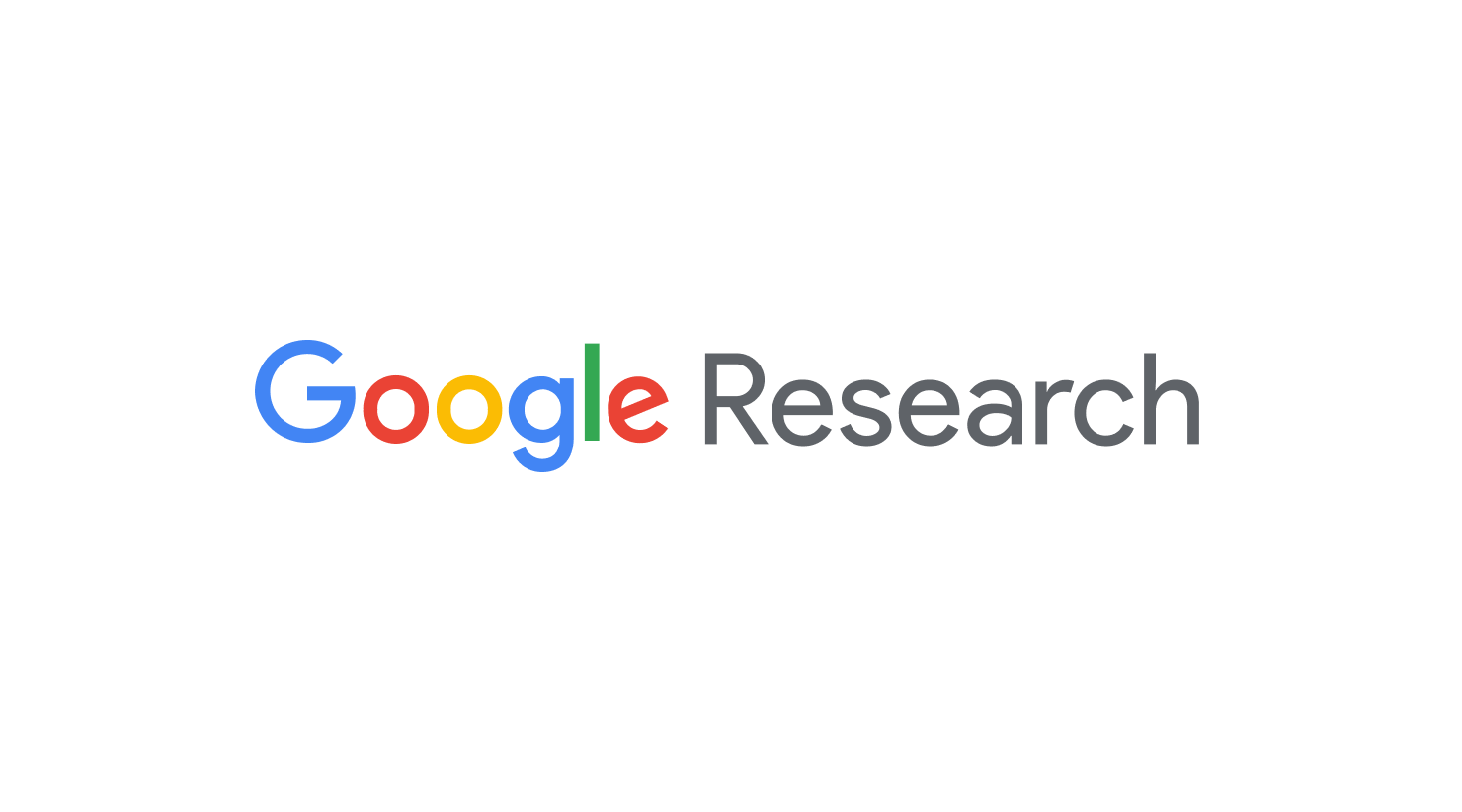
Google at ICLR 2019
May 6, 2019
Posted by Andrew Helton, Editor, Google AI Communications
This week, New Orleans, LA hosts the 7th International Conference on Learning Representations (ICLR 2019), a conference focused on how one can learn meaningful and useful representations of data for machine learning. ICLR offers conference and workshop tracks, both of which include invited talks along with oral and poster presentations of some of the latest research on deep learning, metric learning, kernel learning, compositional models, non-linear structured prediction and issues regarding non-convex optimization.
At the forefront of innovation in neural networks and deep learning, Google focuses on on both theory and application, developing learning approaches to understand and generalize. As Platinum Sponsor of ICLR 2019, Google will have a strong presence with over 200 researchers attending, contributing to and learning from the broader academic research community by presenting papers and posters, in addition to participating on organizing committees and in workshops.
If you are attending ICLR 2019, we hope you'll stop by our booth and chat with our researchers about the projects and opportunities at Google that go into solving interesting problems for billions of people. You can also learn more about our research being presented at ICLR 2019 in the list below (Googlers highlighted in blue).
Officers and Board Members
Hugo Larochelle, Samy Bengio, Tara Sainath
General Chair
Tara Sainath
Workshop Chairs
Been Kim, Graham Taylor
Program Committee includes:
Chelsea Finn, Dale Schuurmans, Dumitru Erhan, Katherine Heller, Lihong Li, Samy Bengio, Rohit Prabhavalkar, Alex Wiltschko, Slav Petrov, George Dahl
Oral Contributions
Generating High Fidelity Images with Subscale Pixel Networks and Multidimensional Upscaling
Jacob Menick, Nal Kalchbrenner
Enabling Factorized Piano Music Modeling and Generation with the MAESTRO Dataset
Curtis Hawthorne, Andrew Stasyuk, Adam Roberts, Ian Simon, Anna Huang, Sander Dieleman, Erich Elsen, Jesse Engel, Douglas Eck
Meta-Learning Update Rules for Unsupervised Representation Learning
Luke Metz, Niru Maheswaranathan, Brian Cheung, Jascha Sohl-Dickstein
Posters
A Data-Driven and Distributed Approach to Sparse Signal Representation and Recovery
Ali Mousavi, Gautam Dasarathy, Richard G. Baraniuk
Bayesian Deep Convolutional Networks with Many Channels are Gaussian Processes
Roman Novak, Lechao Xiao, Yasaman Bahri, Jaehoon Lee, Greg Yang, Jiri Hron, Daniel A. Abolafia, Jeffrey Pennington, Jascha Sohl-Dickstein
Diversity-Sensitive Conditional Generative Adversarial Networks
Dingdong Yang, Seunghoon Hong, Yunseok Jang, Tianchen Zhao, Honglak Lee
Diversity and Depth in Per-Example Routing Models
Prajit Ramachandran, Quoc V. Le
Eidetic 3D LSTM: A Model for Video Prediction and Beyond
Yunbo Wang, Lu Jiang, Ming-Hsuan Yang, Li-Jia Li, Mingsheng Long, Li Fei-Fei
GANSynth: Adversarial Neural Audio Synthesis
Jesse Engel, Kumar Krishna Agrawal, Shuo Chen, Ishaan Gulrajani, Chris Donahue, Adam Roberts
K for the Price of 1: Parameter-efficient Multi-task and Transfer Learning
Pramod Kaushik Mudrakarta, Mark Sandler, Andrey Zhmoginov, Andrew Howard
Learning to Describe Scenes with Programs
Yunchao Liu, Zheng Wu, Daniel Ritchie, William Freeman, Joshua B Tenenbaum, Jiajun Wu
Learning to Infer and Execute 3D Shape Programs
Yonglong Tian, Andrew Luo, Xingyuan Sun, Kevin Ellis, William Freeman, Joshua B Tenenbaum, Jiajun Wu
The Singular Values of Convolutional Layers
Hanie Sedghi, Vineet Gupta, Philip M. Long
Unsupervised Discovery of Parts, Structure, and Dynamics
Zhenjia Xu, Zhijian Liu, Chen Sun, Kevin Murphy, William Freeman, Joshua B Tenenbaum, Jiajun Wu
Adversarial Reprogramming of Neural Networks
Gamaleldin Elsayed, Ian Goodfellow (no longer at Google), Jascha Sohl-Dickstein
Discriminator Rejection Sampling
Ian Goodfellow (no longer at Google), Jascha Sohl-Dickstein
On Self Modulation for Generative Adversarial Networks
Ting Chen, Mario Lucic, Neil Houlsby, Sylvain Gelly
Towards GAN Benchmarks Which Require Generalization
Ishaan Gulrajani, Colin Raffel, Luke Metz
Understanding and Improving Interpolation in Autoencoders via an Adversarial Regularizer
David Berthelot, Colin Raffel, Aurko Roy, Ian Goodfellow (no longer at Google)
A new dog learns old tricks: RL finds classic optimization algorithms
Weiwei Kong, Christopher Liaw, Aranyak Mehta, D. Sivakumar
Contingency-Aware Exploration in Reinforcement Learning
Jongwook Choi, Yijie Guo, Marcin Moczulski, Junhyuk Oh, Neal Wu, Mohammad Norouzi, Honglak Lee
Discriminator-Actor-Critic: Addressing Sample Inefficiency and Reward Bias in Adversarial Imitation Learning
Ilya Kostrikov, Kumar Krishna Agrawal, Debidatta Dwibedi, Sergey Levine, Jonathan Tompson
Diversity is All You Need: Learning Skills without a Reward Function
Benjamin Eysenbach, Abhishek Gupta, Julian Ibarz, Sergey Levine
Episodic Curiosity through Reachability
Nikolay Savinov, Anton Raichuk, Raphael Marinier, Damien Vincent, Marc Pollefeys, Timothy Lillicrap, Sylvain Gelly
Learning to Navigate the Web
Izzeddin Gur, Ulrich Rueckert, Aleksandra Faust, Dilek Hakkani-Tur
Meta-Learning Probabilistic Inference for Prediction
Jonathan Gordon, John Bronskill, Matthias Bauer, Sebastian Nowozin, Richard E. Turner
Multi-step Retriever-Reader Interaction for Scalable Open-domain Question Answering
Rajarshi Das, Shehzaad Dhuliawala, Manzil Zaheer, Andrew McCallum
Near-Optimal Representation Learning for Hierarchical Reinforcement Learning
Ofir Nachum, Shixiang Gu, Honglak Lee, Sergey Levine
Neural Logic Machines
Honghua Dong, Jiayuan Mao, Tian Lin, Chong Wang, Lihong Li, Dengyong Zhou
Neural Program Repair by Jointly Learning to Localize and Repair
Marko Vasic, Aditya Kanade, Petros Maniatis, David Bieber, Rishabh Singh
Optimal Completion Distillation for Sequence Learning
Sara Sabour, William Chan, Mohammad Norouzi
Recall Traces: Backtracking Models for Efficient Reinforcement Learning
Anirudh Goyal, Philemon Brakel, William Fedus, Soumye Singhal, Timothy Lillicrap, Sergey Levine, Hugo Larochelle, Yoshua Bengio
Sample Efficient Adaptive Text-to-Speech
Yutian Chen, Yannis M Assael, Brendan Shillingford, David Budden, Scott Reed, Heiga Zen, Quan Wang, Luis C. Cobo, Andrew Trask, Ben Laurie, Caglar Gulcehre, Aaron van den Oord, Oriol Vinyals, Nando de Freitas
Synthetic Datasets for Neural Program Synthesis
Richard Shin, Neel Kant, Kavi Gupta, Chris Bender, Brandon Trabucco, Rishabh Singh, Dawn Song
The Laplacian in RL: Learning Representations with Efficient Approximations
Yifan Wu, George Tucker, Ofir Nachum
A Mean Field Theory of Batch Normalization
Greg Yang, Jeffrey Pennington, Vinay Rao, Jascha Sohl-Dickstein, Samuel S Schoenholz
Efficient Training on Very Large Corpora via Gramian Estimation
Walid Krichene, Nicolas Mayoraz, Steffen Rendle, Li Zhang, Xinyang Yi, Lichan Hong, Ed Chi, John Anderson
Predicting the Generalization Gap in Deep Networks with Margin Distributions
Yiding Jiang, Dilip Krishnan, Hossein Mobahi, Samy Bengio
InfoBot: Transfer and Exploration via the Information Bottleneck
Anirudh Goyal, Riashat Islam, DJ Strouse, Zafarali Ahmed, Hugo Larochelle, Matthew Botvinick, Sergey Levine, Yoshua Bengio
AntisymmetricRNN: A Dynamical System View on Recurrent Neural Networks
Bo Chang, Minmin Chen, Eldad Haber, Ed H. Chi
Complement Objective Training
Hao-Yun Chen, Pei-Hsin Wang, Chun-Hao Liu, Shih-Chieh Chang, Jia-Yu Pan, Yu-Ting Chen, Wei Wei, Da-Cheng Juan
DOM-Q-NET: Grounded RL on Structured Language
Sheng Jia, Jamie Kiros, Jimmy Ba
From Language to Goals: Inverse Reinforcement Learning for Vision-Based Instruction Following
Justin Fu, Anoop Korattikara Balan, Sergey Levine, Sergio Guadarrama
Harmonic Unpaired Image-to-image Translation
Rui Zhang, Tomas Pfister, Li-Jia Li
Hierarchical Generative Modeling for Controllable Speech Synthesis
Wei-Ning Hsu, Yu Zhang, Ron Weiss, Heiga Zen, Yonghui Wu, Yuxuan Wang, Yuan Cao, Ye Jia, Zhifeng Chen, Jonathan Shen, Patrick Nguyen, Ruoming Pang
Learning Finite State Representations of Recurrent Policy Networks
Anurag Koul, Alan Fern, Samuel Greydanus
Learning to Screen for Fast Softmax Inference on Large Vocabulary Neural Networks
Patrick Chen, Si Si, Sanjiv Kumar, Yang Li, Cho-Jui Hsieh
Music Transformer: Generating Music with Long-Term Structure
Chen-Zhi Anna Huang, Ashish Vaswani, Jakob Uszkoreit, Ian Simon, Curtis Hawthorne, Noam Shazeer, Andrew Dai, Matthew D Hoffman, Monica Dinculescu, Douglas Eck
Universal Transformers
Mostafa Dehghani, Stephan Gouws, Oriol Vinyals, Jakob Uszkoreit, Lukasz Kaiser
What do you learn from context? Probing for sentence structure in contextualized word representations
Ian Tenney, Patrick Xia, Berlin Chen, Alex Wang, Adam Poliak, Tom McCoy, Najoung Kim, Benjamin Van Durme, Samuel R. Bowman, Dipanjan Das, Ellie Pavlick
Doubly Reparameterized Gradient Estimators for Monte Carlo Objectives
George Tucker, Dieterich Lawson, Shixiang Gu, Chris J. Maddison
How Important Is a Neuron?
Kedar Dhamdhere, Mukund Sundararajan, Qiqi Yan
Integer Networks for Data Compression with Latent-Variable Models
Johannes Ballé, Nick Johnston, David Minnen
Modeling Uncertainty with Hedged Instance Embeddings
Seong Joon Oh, Andrew Gallagher, Kevin Murphy, Florian Schroff, Jiyan Pan, Joseph Roth
Preventing Posterior Collapse with delta-VAEs
Ali Razavi, Aaron van den Oord, Ben Poole, Oriol Vinyals
Spectral Inference Networks: Unifying Deep and Spectral Learning
David Pfau, Stig Petersen, Ashish Agarwal, David GT Barrett, Kimberly L Stachenfeld
Stochastic Prediction of Multi-Agent Interactions from Partial Observations
Chen Sun, Per Karlsson, Jiajun Wu, Joshua B Tenenbaum, Kevin Murphy
Workshops
Learning from Limited Labeled Data
Sponsored by Google
Deep Reinforcement Learning Meets Structured Prediction
Organizing Committee includes: Chen Liang
Invited Speaker: Mohammad Norouzi
Debugging Machine Learning Models
Organizing Committee includes: D. Sculley
Invited Speaker: Dan Moldovan
Structure & Priors in Reinforcement Learning (SPiRL)
Organizing Committee includes: Chelsea Finn
Task-Agnostic Reinforcement Learning (TARL)
Sponsored by Google
Organizing Committee includes: Danijar Hafner, Marc G. Bellemare
Invited Speaker: Chelsea Finn
AI for Social Good
Program Committee includes: Ernest Mwebaze
Safe Machine Learning Specification, Robustness and Assurance
Program Committee includes: Nicholas Carlini
Representation Learning on Graphs and Manifolds
Program Committee includes: Bryan Perozzi
-
Labels:
- Conferences & Events