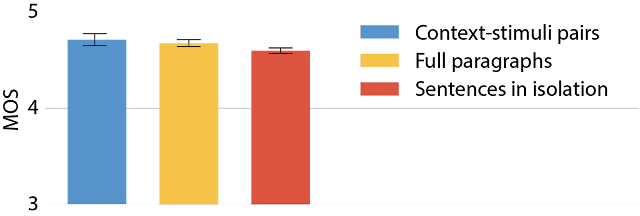
Assessing the Quality of Long-Form Synthesized Speech
September 9, 2019
Posted by Tom Kenter, Google Research, London
Automatically generated speech is everywhere, from directions being read out aloud while you are driving, to virtual assistants on your phone or smart speaker devices at home. While much research is being done to try to make synthesized speech sound as natural as possible—such as generating speech for low-resource languages and creating human-like speech with Tacotron 2—how does one evaluate the generated speech? The best way to find out is to ask people, who are very good at telling if something sounds natural or not.
In the field of speech synthesis, subjects are routinely asked to listen to samples of synthesized speech and rate their quality. Yet, until now, evaluation of synthesized speech has been done on a sentence-by-sentence basis. But often one wants to know the quality of a series of sentences that belong together, such as a paragraph in a news article or a turn in a conversation. This is where it gets interesting, as there is more than one way of evaluating sentences that naturally occur in a sequence, and, surprisingly, a rigorous comparison of these different methods has not been carried out. This in turn can hinder research progress in developing products that rely on generated speech.
To address this challenge, we present “Evaluating Long-form Text-to-Speech: Comparing the Ratings of Sentences and Paragraphs”, a publication to appear at SSW10 in which we compare several ways of evaluating synthesized speech for multi-line texts. We find that when a sentence is evaluated as part of a longer text involving several sentences, the outcome is influenced by the way in which the audio sample is presented to the people evaluating it. For example, when the sentence is presented by itself, without any context, the rating people give on average is substantially different from the rating they give when they listen to the same sentence with some context (while the context doesn't have to be rated).
Evaluating Automatically Generated Speech
To determine the quality of speech signals, it is common practice to ask several human raters to give their opinion for a particular sample, on a 1-to-5 scale. This sample can be automatically generated, but it can also be natural speech (i.e., an actual person saying a sentence out loud), which serves as a control. The scores of all reviewers rating a particular speech sample are averaged to get a Mean Opinion Score (MOS).
Until now, MOS ratings were typically collected per sentence, i.e., raters listened to sentences in isolation to form their opinion. Instead of this typical approach, we consider three different ways of presenting speech samples to raters—both with and without context—and we show that each approach yields different results. The first, presenting the sentence in isolation, is the default method commonly used in the field. An alternative method is to provide the full context for the sentence. In this case, the entire paragraph to which the sentence belongs is included and the ensemble is rated. The final approach is to provide a context-stimulus pair. Here, rather than providing full context, only some context is provided, such as the preceding sentence(s) from the original paragraph.
Interestingly, these three different approaches for presenting speech give different results even when applied to natural speech. This is demonstrated in the figure below, where the MOS scores are presented for natural speech samples rated using the three different methods of presentation. Even though the sentences being rated are identical across the three different settings, the scores are different on average, depending on the context in which they were presented.
![]() |
MOS results for natural speech from a dataset consisting of news articles. Though the differences appear small, they are significant between all conditions (two-tailed t-test with α=0.05). |
When evaluated synthesized speech, the differences are more pronounced.
![]() |
MOS results for synthesized speech on the same news article dataset used above. All lines are synthesized speech, unless indicated otherwise. |
Predicting Paragraph Score
When an entire paragraph of synthesized speech is played (the yellow bar), this is perceived as even less natural than in the other settings. Our original hypothesis was a weakest-link argument—the rating is probably as bad as the worst sentence in the paragraph. If that were the case, it should be easy to predict the rating of a paragraph by considering the ratings of the individual sentences in it, perhaps simply taking the minimum value to get the paragraph rating. It turns out, however, that does not work.
The failure of the weakest-link hypothesis may be due to more subtle factors that are difficult to tease out with such a simple approach. To test this, we also trained a machine learning algorithm to predict the paragraph score from the individual sentences. However, this approach, too, was unable to successfully predict paragraph scores reliably.
Conclusion
Evaluating synthesized speech is not straightforward when multiple sentences are involved. The traditional paradigm of rating sentences in isolation does not give the full picture, and one should be aware of anchoring effects when context is provided. Rating full paragraphs might be the most conservative approach. We hope our findings help advance future work in speech synthesis where long-form content is concerned, such as audio book readers and conversational agents.
Acknowledgments
Many thanks to all authors of the paper: Rob Clark, Hanna Silen, Ralph Leith.