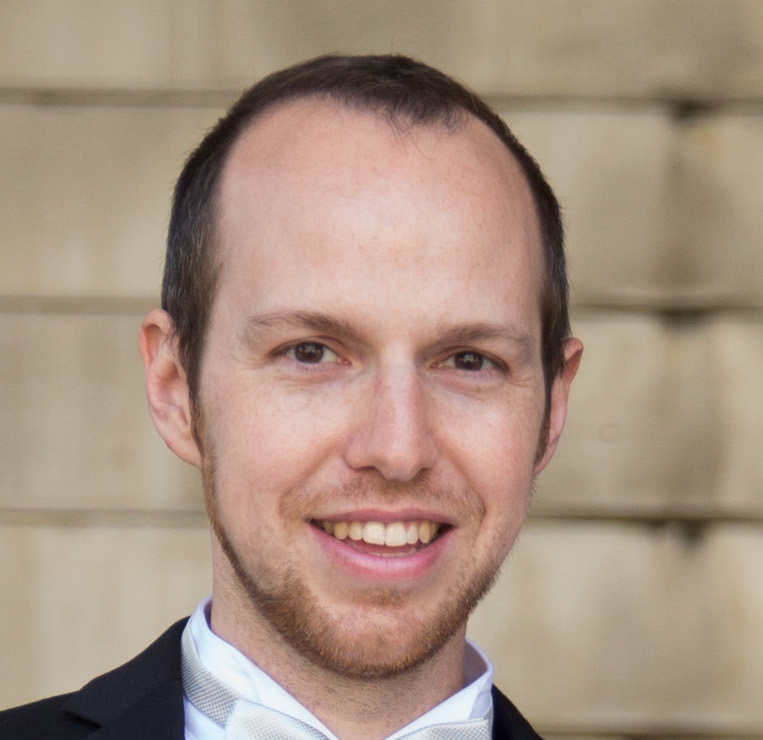
Tobias Weyand
After finishing his PhD studies in Computer Vision at RWTH Aachen University, Germany, Tobias Weyand joined Google in 2014. His research interests include large-scale image retrieval and clustering, place recognition, and image-based localization.
Research Areas
Authored Publications
Sort By
Improving Fairness in Large-Scale Object Recognition by CrowdSourced Demographic Information
Bingyi Cao
Cam Askew
Jack Sim
Mike Green
N'Mah Fodiatu Yilla-Akbari
Zu Kim
arXiv(2022)
Preview abstract
There has been increasing awareness of ethical issues in machine learning, and fairness has become an important research topic. Most fairness efforts in computer vision have been focused on human sensing applications and preventing discrimination by people's physical attributes such as race, skin color or age by increasing visual representation for particular demographic groups. We argue that ML fairness efforts should extend to object recognition as well. Buildings, artwork, food and clothing are examples of the objects that define human culture. Representing these objects fairly in machine learning datasets will lead to models that are less biased towards a particular culture and more inclusive of different traditions and values. There exist many research datasets for object recognition, but they have not carefully considered which classes should be included, or how much training data should be collected per class. To address this, we propose a simple and general approach, based on crowdsourcing the demographic composition of the contributors: we define fair relevance scores, estimate them, and assign them to each class. We showcase its application to the landmark recognition domain, presenting a detailed analysis and the final fairer landmark rankings. We present analysis which leads to a much fairer coverage of the world compared to existing datasets. The evaluation dataset was used for a public image recognition challenge, which was the first of a kind with an emphasis on fairness in generic object recognition.
View details
Towards A Fairer Landmark Recognition Dataset
Bingyi Cao
Cam Askew
Jack Sim
Mike Green
N'Mah Fodiatu Yilla-Akbari
Zu Kim
arXiv(2021)
Preview abstract
We introduce a new landmark recognition dataset, whichis created with a focus on fair worldwide representation.While previous work proposes to collect as many imagesas possible from web repositories, we instead argue thatsuch approaches can lead to biased data. To create a morecomprehensive and equitable dataset, we start by definingthe fairrelevanceof a landmark to the world population.These relevances are estimated by combining anonymizedGoogle Maps user contribution statistics with the contribu-tors’ demographic information. We present a stratificationapproach and analysis which leads to a much fairer cover-age of the world, compared to existing datasets. The result-ing datasets are used to evaluate computer vision models aspart of the the Google Landmark Recognition and RetrievalChallenges 2021.
View details
Preview abstract
While image retrieval and instance recognition techniques are progressing rapidly, there is a need for challenging datasets to accurately measure their performance -- while posing novel challenges that are relevant for practical applications. We introduce the Google Landmarks Dataset v2 (GLDv2), a new benchmark for large-scale, fine-grained instance recognition and image retrieval in the domain of human-made and natural landmarks. GLDv2 is the largest such dataset to date by a large margin, including over 5M images and 200k distinct instance labels. Its test set consists of 118k images with ground truth annotations for both the retrieval and recognition tasks. The ground truth construction involved over 800 hours of human annotator work. Our new dataset has several challenging properties inspired by real world applications that previous datasets did not consider: An extremely long-tailed class distribution, a large fraction of out-of-domain test photos and large intra-class variability. The dataset is sourced from Wikimedia Commons, the world's largest crowdsourced collection of landmark photos. We provide baseline results for both recognition and retrieval tasks based on state-of-the-art methods as well as competitive results from a public challenge. We further demonstrate the suitability of the dataset for transfer learning by showing that image embeddings trained on it achieve competitive retrieval performance on independent datasets. The dataset images, ground-truth and metric scoring code are available at this URL.
View details
CPlaNet: Enhancing Image Geolocalization by Combinatorial Partitioning of Maps
Paul Hongsuck Seo
Jack Sim
Bohyung Han
European Conference on Computer Vision (ECCV)(2018)
Preview abstract
Image geolocalization is the task of identifying the location depicted in a photo based only on its visual information. This task is inherently challenging since many photos have only few, possibly ambiguous cues to their geolocation. Recent work has cast this task as a classification problem by partitioning the earth into a set of discrete cells that correspond to geographic regions. The granularity of this partitioning presents a critical trade-off; using fewer but larger cells results in lower location accuracy while using more but smaller cells reduces the number of training examples per class and increases model size, making the model prone to overfitting. To tackle this issue, we propose a simple but effective algorithm, combinatorial partitioning, which generates a large number of fine-grained output classes by intersecting multiple coarse-grained partitionings of the earth. Each classifier votes for the fine-grained classes that overlap with their respective coarse-grained ones. This technique allows us to predict locations at a fine scale while maintaining sufficient training examples per class. Our algorithm achieves the state-of-the-art performance in location recognition on multiple benchmark datasets.
View details
Preview abstract
We propose an attentive local feature descriptor suitable for large-scale image retrieval, referred to as DELF (DEep Local Feature). The new feature is based on convolutional neural networks, which are trained only with image-level annotations on a landmark image dataset. To identify semantically useful local features for image retrieval, we also propose an attention mechanism for keypoint selection, which shares most network layers with the descriptor. This framework can be used for image retrieval as a drop-in replacement for other keypoint detectors and descriptors, enabling more accurate feature matching and geometric verification. Our system produces reliable confidence scores to reject false positives---in particular, it is robust against queries that have no correct match in the database. To evaluate the proposed descriptor, we introduce a new large-scale dataset, referred to as Google-Landmarks dataset, which involves challenges in both database and query such as background clutter, partial occlusion, multiple landmarks, objects in variable scales, etc. We show that DELF outperforms the state-of-the-art global and local descriptors in the large-scale setting by significant margins.
View details
Preview abstract
Is it possible to determine the location of a photo from just its pixels? While the general problem seems exceptionally difficult, photos often contain cues such as landmarks, weather patterns, vegetation, road markings, and architectural details, which in combination allow to infer the location. In computer vision, this problem is usually approached using image retrieval methods. In contrast, we pose the problem as one of classification by subdividing the surface of the earth into thousands of multi-scale geographic cells, and train a deep network using millions of geotagged images. We show that the resulting model, called PlaNet, outperforms previous approaches and even attains superhuman accuracy in some cases. Moreover, we extend our model to photo albums by combining it with a long short-term memory (LSTM) architecture. By learning to exploit temporal coherence to geolocate uncertain photos, this model achieves a 50% performance improvement over the single-image model.
View details