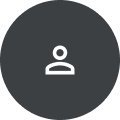
Sven Gowal
Research Areas
Authored Publications
Sort By
Generative models improve fairness of medical classifiers under distribution shifts
Ira Ktena
Olivia Wiles
Isabela Albuquerque
Sylvestre-Alvise Rebuffi
Ryutaro Tanno
Danielle Belgrave
Taylan Cemgil
Nature Medicine(2024)
Preview abstract
Domain generalization is a ubiquitous challenge for machine learning in healthcare. Model performance in real-world conditions might be lower than expected because of discrepancies between the data encountered during deployment and development. Underrepresentation of some groups or conditions during model development is a common cause of this phenomenon. This challenge is often not readily addressed by targeted data acquisition and ‘labeling’ by expert clinicians, which can be prohibitively expensive or practically impossible because of the rarity of conditions or the available clinical expertise. We hypothesize that advances in generative artificial intelligence can help mitigate this unmet need in a steerable fashion, enriching our training dataset with synthetic examples that address shortfalls of underrepresented conditions or subgroups. We show that diffusion models can automatically learn realistic augmentations from data in a label-efficient manner. We demonstrate that learned augmentations make models more robust and statistically fair in-distribution and out of distribution. To evaluate the generality of our approach, we studied three distinct medical imaging contexts of varying difficulty: (1) histopathology, (2) chest X-ray and (3) dermatology images. Complementing real samples with synthetic ones improved the robustness of models in all three medical tasks and increased fairness by improving the accuracy of clinical diagnosis within underrepresented groups, especially out of distribution.
View details
Benchmarking Robustness to Adversarial Image Obfuscations
Florian Stimberg
Yintao Liu
Merve Kaya
Cyrus Rashtchian
Ariel Fuxman
Mehmet Tek
Advances in Neural Information Processing Systems(2023)
Preview abstract
Automated content filtering and moderation is an important tool that allows online platforms to build striving user communities that facilitate cooperation and prevent abuse. Unfortunately, resourceful actors try to bypass automated filters in a bid to post content that violate platform policies and codes of conduct. To reach this goal, these malicious actors obfuscate policy violating content to prevent machine learning models from reaching the correct decision. In this paper, we invite researchers to tackle this specific issue and present a new image benchmark. This benchmark, based on ImageNet, simulates the type of obfuscations created by malicious actors. It goes beyond ImageNet-C and ImageNet-C-Bar by proposing general, drastic, adversarial modifications that preserve the original content intent. It aims to tackle a more common adversarial threat than the one considered by Lp-norm bounded adversaries. Our hope is that this benchmark will encourage researchers to test their models and methods and try to find new approaches that are more robust to these obfuscations.
View details
Challenges of Real-World Reinforcement Learning:Definitions, Benchmarks & Analysis
Cosmin Paduraru
Daniel J. Mankowitz
Jerry Li
Nir Levine
Todd Hester
Machine Learning Journal(2021)
Preview abstract
Reinforcement learning (RL) has proven its worth in a series of artificialdomains, and is beginning to show some successes in real-world scenarios. However,much of the research advances in RL are hard to leverage in real-world systemsdue to a series of assumptions that are rarely satisfied in practice. We identifyand formalize a series of independent challenges that embody the difficulties thatmust be addressed for RL to be commonly deployed in real-world systems. Foreach challenge, we define it formally in the context of a Markov Decision Process,analyze the effects of the challenge on state-of-the-art learning algorithms, andpresent some existing attempts at tackling it. We believe that an approach thataddresses all nine challenges would be readily deployable in a large number of realworld problems. We implement our proposed challenges in a suite of continuouscontrol environments calledrealworldrl-suitewhich we propose an as an open-source benchmark.
View details