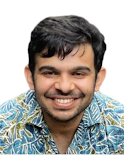
Saurabh Kadekodi
Saurabh Kadekodi is a research scientist working in the Storage Analytics team. He specializes in reliability anf performance of large-scale storage clusters. Saurabh completed his Ph.D. from Carnegie Mellon University as part of the Parallel Data Laboratory. Prior to that he has done his Masters from Northwestern University and bachelors from Pune Institute of Computer Technology, India.
Authored Publications
Google Publications
Other Publications
Sort By
Thesios: Synthesizing Accurate Counterfactual I/O Traces from I/O Samples
Soroush Ghodrati
Selene Moon
ASPLOS 2024, Association for Computing Machinery
Preview abstract
Representative modeling of I/O activity is crucial when designing large-scale distributed storage systems. Particularly important use cases are counterfactual “what-if” analyses that assess the impact of anticipated or hypothetical new storage policies or hardware prior to deployment. We propose Thesios, a methodology to accurately synthesize such hypothetical full-resolution I/O traces by carefully combining down-sampled I/O traces collected from multiple disks attached to multiple storage servers. Applying this approach to real-world traces that a real ready routinely sampled at Google, we show that our synthesized traces achieve 95–99.5% accuracy in read/write request numbers, 90–97% accuracy in utilization, and 80–99.8% accuracy in read latency compared to metrics collected from actual disks. We demonstrate how The-sios enables diverse counterfactual I/O trace synthesis and analyses of hypothetical policy, hardware, and server changes through four case studies: (1) studying the effects of changing disk’s utilization, fullness, and capacity, (2) evaluating new data placement policy, (3) analyzing the impact on power and performance of deploying disks with reduced rotations-per-minute (RPM), and (4) understanding the impact of increased buffer cache size on a storage server. Without Thesios, such counterfactual analyses would require costly and potentially risky A/B experiments in production.
View details
Practical Design Considerations for Wide Locally Recoverable Codes (LRCs)
Shashwat Silas
Dave Clausen
File and Storage Technologies (FAST), USENIX (2023)
Preview abstract
Most of the data in large-scale storage clusters is erasure coded. At exascale, optimizing erasure codes for low storage overhead, efficient reconstruction, and easy deployment is of critical importance. Locally recoverable codes (LRCs) have deservedly gained central importance in this field, because they can balance many of these requirements. In our work we study wide LRCs; LRCs with large number of blocks per stripe and low storage overhead. These codes are a natural next step for practitioners to unlock higher storage savings, but they come with their own challenges. Of particular interest is their reliability, since wider stripes are prone to more simultaneous failures.
We conduct a practically-minded analysis of several popular and novel LRCs. We find that wide LRC reliability is a subtle phenomenon that is sensitive to several design choices, some of which are overlooked by theoreticians, and others by practitioners. Based on these insights, we construct novel LRCs called Uniform Cauchy LRCs, which show excellent performance in simulations, and a 33% improvement in reliability on unavailability events observed by a wide LRC deployed in a Google storage cluster. We also show that these codes are easy to deploy in a manner that improves their robustness to common maintenance events. Along the way, we also give a remarkably simple and novel construction of distance optimal LRCs (other constructions are also known), which may be of interest to theory-minded readers.
View details
Tiger: disk-adaptive redundancy without placement restrictions
Francisco Maturana
Sanjith Athlur
Rashmi KV
Gregory R. Ganger
Tiger: disk-adaptive redundancy without placement restrictions (2022)
Preview abstract
Large-scale cluster storage systems use redundancy (via erasure coding) to ensure data durability. Disk-adaptive redundancy—dynamically tailoring the redundancy scheme to observed disk failure rates—promises significant space and cost savings. Existing disk-adaptive redundancy systems, however, pose undesirable constraints on data placement, partitioning disks into subclusters with homogeneous failure rates and forcing each erasure-coded stripe to be entirely placed on the disks within one subcluster. This design increases risk, by reducing intra-stripe diversity and being more susceptible to unanticipated changes in a make/model’s failure rate, and only works for very large storage clusters fully committed to
disk-adaptive redundancy.
Tiger is a new disk-adaptive redundancy system that efficiently avoids adoption-blocking placement constraints, while also providing higher space-savings and lower risk relative to prior designs. To do so, Tiger introduces the eclectic stripe, in which disks with different failure rates can be used to store a stripe that has redundancy tailored to the set of failure rates of those disks. With eclectic stripes, pre-existing placement policies can be used while still enjoying the space-savings and robustness benefits of disk-adaptive redundancy. This paper introduces eclectic striping and Tiger’s design, including a new mean time-to-data-loss (MTTDL) approximation technique and new approaches for ensuring safe per-stripe settings given that failure rates of different devices change over time. Evaluation with logs from real-world clusters show that Tiger provides better space-savings, less bursty IO for changing redundancy schemes, and better robustness (due to increased risk-diversity) than prior disk-adaptive redundancy designs.
View details
Preview abstract
Small (kilobyte-sized) objects are the bane of highly scalable cloud object stores. Larger (at least megabytesized) objects not only improve performance, but also result in orders of magnitude lower cost, due to the current operation-based pricing model of commodity cloud object stores. For example, in Amazon S3’s current pricing scheme, uploading 1GiB data by issuing 4KiB PUT requests (at 0.0005¢ each) is approximately 57x more expensive than storing that same 1GiB for a month. To address this problem, we propose client-side packing of small immutable files into gigabyte-sized blobs with embedded indices to identify each file’s location. Experiments with a packing implementation in Alluxio (an open-source distributed file system) illustrate the potential benefits, such as simultaneously increasing file creation throughput by up to 60x and decreasing cost to 1/25000 of the original.
View details
Preview abstract
With the ever increasing filesystem sizes, there is a constant need for faster filesystem access. A vital requirement to achieve this is efficient filesystem metadata management. The bitmap technique currently used to manage free space in Ext4 is faced by scalability challenges owing to this exponential increase. This has led us to re-examine the available choices and explore a radically different design of managing free space called Space Maps. This paper describes the design and implementation of space maps in Ext4. The paper also highlights the limitations of bitmaps and does a comparative study of howspace maps fare against them. In space maps, free space is represented by extent based red-black trees and logs. The design of space maps makes the free space information of the filesystem extremely compact allowing it to be stored in main memory at all times. This significantly reduces the long, random seeks on the disk that were required for updating the metadata. Likewise, analogous on-disk structures and their interaction with the in-memory space maps ensure that filesystem integrity is maintained. Since seeks are the bottleneck as far as filesystem performance is concerned, their extensive reduction leads to faster filesystem operations. Apart from the allocation/deallocation improvements, the log based design of Space Maps helps reduce fragmentation at the filesystem level itself. Space Maps uplift the performance of the filesystem and keep the metadata management in tune with the highly scalable Ext4.
View details
No Results Found