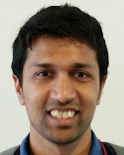
Pramod Kaushik Mudrakarta
Research Areas
Authored Publications
Google Publications
Other Publications
Sort By
K for the price of 1. Parameter efficient multi-task and transfer learning
Andrew Howard
International Conference on Learning Representations (2019)
Preview abstract
In this paper we introduce a novel method that enables parameter efficient transfer and multitask learning.
We show that by reusing more than 95\% of the parameters we can re-purpose neural networks to solve very
different types of problems such as going from COCO-dataset SSD detection to Imagenet classification.
Our approach allows both simultaneous (e.g. multi-task) learning as well as sequential fine-tuning where
we change the already trained networks to solve a different problem.
We show that our approach leads to significant increase in accuracy when compared to traditional logits-only fine-tuning
while using much fewer parameters. Interestingly, for multi-task learning our approach sometimes acts as a regularizer often leading
to improved performance when compared to models trained on a single task.
Our approach has multiple immediate applications. It can be used to dramatically increase the number of models available in resource-constrained settings, since the marginal cost of a new model is now less than 5\% of the full model. The constrained fine-tuning enables better generalization when limited amount data is available. We evaluate our approach on multiple datasets and multiple models.
View details
Preview abstract
We analyze state-of-the-art deep learning
models for three tasks: question answering
on (1) images, (2) tables, and (3) passages
of text. Using the notion of attribution
(word importance), we find that
these deep networks often ignore important
question terms. Leveraging such behavior,
we perturb questions to craft a variety
of adversarial examples. Our strongest
attacks drop the accuracy of a visual question
answering model from 61.1% to 19%,
and that of a tabular question answering
model from 33.5% to 3.3%. Additionally,
we show how attributions can strengthen
attacks proposed by Jia and Liang (2017)
on paragraph comprehension models. Our
results demonstrate that attributions can
augment standard measures of accuracy
and empower investigation of model performance.
When a model is accurate but
for the wrong reasons, attributions can surface
erroneous logic in the model that indicates
inadequacies in the test data.
View details
No Results Found