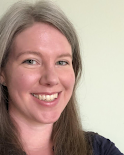
Meg Kurdziolek
Meg is a UX Researcher for Google Cloud AI and Industry Solutions, where she focuses her research on Explainable AI and Model Understanding. She has had a varied career working for start-ups and large corporations alike across fields such as EdTech, weather forecasting, and commercial robotics. She has published articles on topics such as information visualization, educational-technology design, human-robot interaction (HRI), and voice user interface (VUI) design. Meg is also a proud alumnus of Virginia Tech, where she received her Ph.D. in Human-Computer Interaction (HCI).
Research Areas
Authored Publications
Google Publications
Other Publications
Sort By
Explaining the hard to explain: An overview of Explainable AI (XAI) for UX
IxDA-Pittsburgh (2022)
Preview abstract
There is a growing problem facing ML-driven product designers and engineers. Products and services increasingly generate and rely on larger, more complex datasets. As datasets grow in breadth and volume, the ML models built on them are increasing in complexity. But as ML model complexity grows, they become increasingly opaque. Without a means of understanding ML model decision making, end-users are less likely to trust and adopt the technology. Furthermore, the audiences for ML model explanations come from varied backgrounds, have different levels of experience with mathematics and statistics, and will rely on these technologies in a variety of contexts. In order to show the “whys” behind complex machine learning decision making, technologists will need to employ “Explainable AI.” In this talk, I'll sketch out “the basics” of Explainable AI (XAI) and describe, at a high level, popular methods and techniques. Then I describe the current challenges facing the field, and how UX can advocate for better experiences in ML driven products.
View details
Explaining the Unexplainable: Explainable AI (XAI) for UX
UXPA Magazine, vol. 22.3 (2022)
Preview abstract
A problem faces machine learning (ML) product designers. As datasets grow larger and more complex, the ML models built on them grow complex and increasingly opaque. End users are less likely to trust and adopt the technology without clear explanations. Furthermore, audiences for ML model explanations vary considerably in background, experience with mathematical reasoning, and contexts in which they apply these technologies. UX professionals can use explainable artificial intelligence (XAI) methods and techniques to explain the reasoning behind ML products.
View details
Everyday Experiences With Robots
UXPA 2019 (2019)
A Special Topics Course on Personal Information Management
Manuel Pérez-Quiñones
Pardha S. Pyla
TR 06-26, Virginia Tech, Dept. of Computer Science (2006)