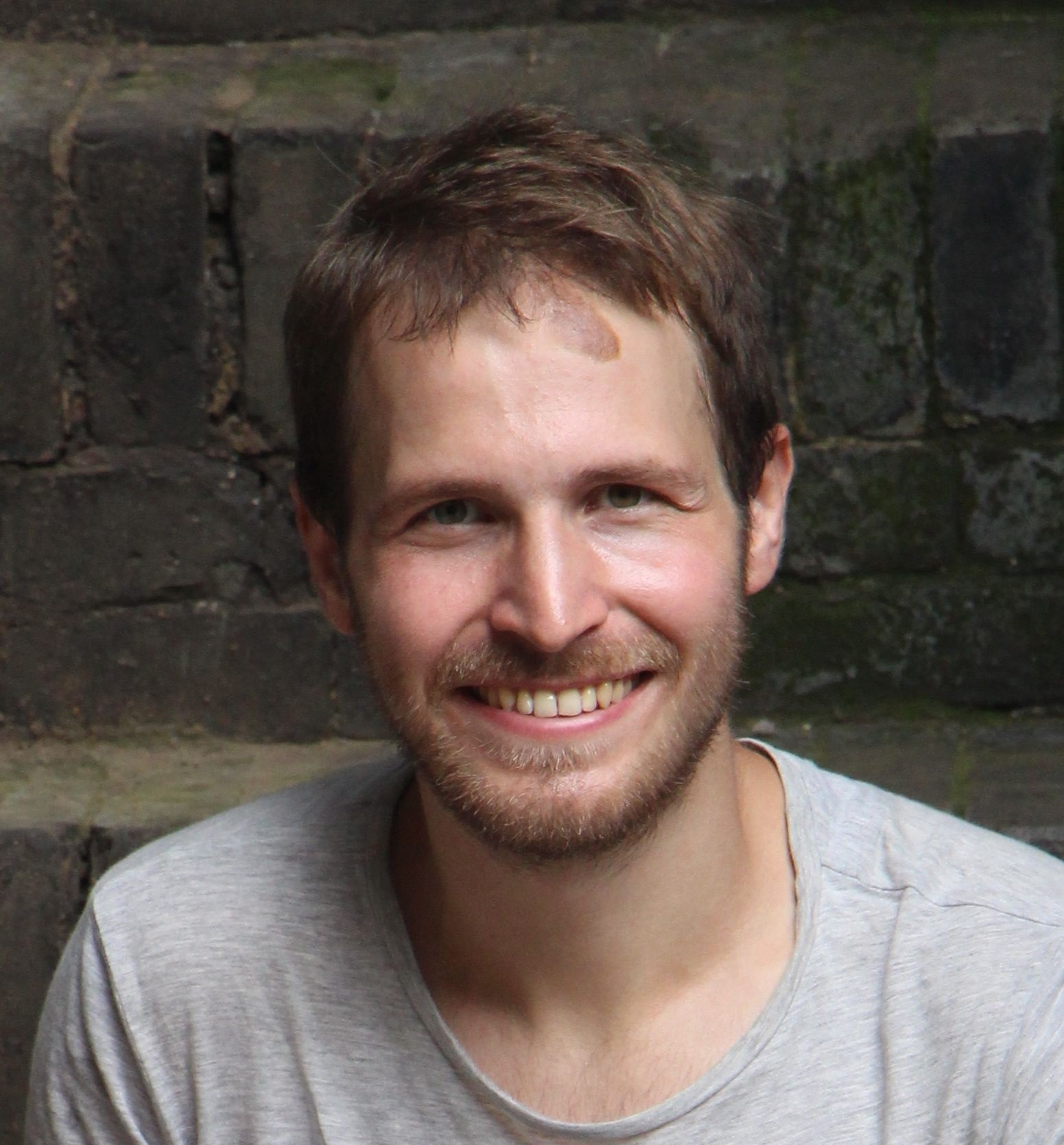
Johannes Gasteiger, né Klicpera
Johannes Gasteiger is a research scientist interested in the safety and interpretability of advanced ML models. He received his PhD from TU Munich for work on how to leverage geometry and structure in graph neural networks, with a particular focus on molecular systems.
Research Areas
Authored Publications
Sort By
Accelerating Molecular Graph Neural Networks via Knowledge Distillation
Filip Ekström Kelvinius
Dimitar Georgiev
Artur Petrov Toshev
Thirty-seventh Conference on Neural Information Processing Systems (NeurIPS)(2023)
Preview abstract
Recent advances in graph neural networks (GNNs) have allowed molecular simulations with accuracy on par with conventional gold-standard methods at a fraction of the computational cost. Nonetheless, as the field has been progressing to bigger and more complex architectures, state-of-the-art GNNs have become largely prohibitive for many large-scale applications. In this paper, we, for the first time, explore the utility of knowledge distillation (KD) for accelerating molecular GNNs. To this end, we devise KD strategies that facilitate the distillation of hidden representations in directional and equivariant GNNs and evaluate their performance on the regression task of energy and force prediction. We validate our protocols across different teacher-student configurations and demonstrate that they can boost the predictive accuracy of student models without altering their architecture. We also conduct comprehensive optimization of various components of our framework, and investigate the potential of data augmentation to further enhance performance. All in all, we manage to close as much as 59% of the gap in predictive accuracy between models like GemNet-OC and PaiNN with zero additional cost at inference.
View details
Ewald-Based Long-Range Message Passing for Molecular Graphs
Arthur Kosmala
Nicholas Gao
Stephan Günnemann
International Conference on Machine Learning (ICML)(2023)
Preview abstract
Neural architectures that learn potential energy surfaces from molecular data have undergone fast improvement in recent years. A key driver of this success is the Message Passing Neural Network (MPNN) paradigm. Its favorable scaling with system size partly relies upon a spatial distance limit on messages. While this focus on locality is a useful inductive bias, it also impedes the learning of long-range interactions such as electrostatics and van der Waals forces. To address this drawback, we propose Ewald message passing: a nonlocal Fourier space scheme which limits interactions via a cutoff on frequency instead of distance, and is theoretically well-founded in the Ewald summation method. It can serve as an augmentation on top of existing MPNN architectures as it is computationally inexpensive and agnostic to architectural details. We test the approach with four baseline models and two datasets containing diverse periodic (OC20) and aperiodic structures (OE62). We observe robust improvements in energy mean absolute errors across all models and datasets, averaging 10% on OC20 and 16% on OE62. Our analysis shows an outsize impact of these improvements on structures with high long-range contributions to the ground truth energy.
View details