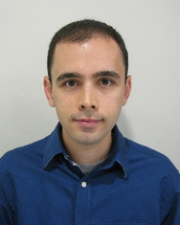
Fernando V. Bonassi
Fernando V. Bonassi graduated with his PhD in Statistics from Duke University in 2013. He also holds BS and MS degrees in Statistics from the University of Sao Paulo (Brazil). He joined Google in 2013, where he works as quantitative analyst. His major research interests include Bayesian computation, dynamic modeling, decision analysis, among other topics.
Research Areas
Authored Publications
Google Publications
Other Publications
Sort By
Bayes and Big Data: The Consensus Monte Carlo Algorithm
Steven L. Scott
Alexander W. Blocker
Hugh A. Chipman
Edward I. George
Robert E. McCulloch
International Journal of Management Science and Engineering Management, 11(2016), pp. 78-88
Preview abstract
A useful definition of ``big data'' is data that is too big to
comfortably process on a single machine, either because of
processor, memory, or disk bottlenecks. Graphics processing units
can alleviate the processor bottleneck, but memory or disk
bottlenecks can only be eliminated by splitting data across multiple
machines. Communication between large numbers of machines is
expensive (regardless of the amount of data being communicated), so
there is a need for algorithms that perform distributed approximate
Bayesian analyses with minimal communication. Consensus Monte Carlo
operates by running a separate Monte Carlo algorithm on each
machine, and then averaging individual Monte Carlo draws across
machines. Depending on the model, the resulting draws can be nearly
indistinguishable from the draws that would have been obtained by
running a single machine algorithm for a very long time. Examples
of consensus Monte Carlo are shown for simple models where
single-machine solutions are available, for large single-layer
hierarchical models, and for Bayesian additive regression trees
(BART).
View details
Sequential Monte Carlo with Adaptive Weights for Approximate Bayesian Computation
Preview abstract
Methods of approximate Bayesian computation (ABC) are increasingly used for analysis of complex models. A major challenge for ABC is over-coming the often inherent problem of high rejection rates in the accept/reject methods based on prior:predictive sampling. A number of recent developments aim to address this with extensions based on sequential Monte Carlo (SMC) strategies. We build on this here, introducing an ABC SMC method that uses data-based adaptive weights. This easily implemented and computationally trivial extension of ABC SMC can very substantially improve acceptance rates, as is demonstrated in a series of examples with simulated and real data sets, including a currently topical example from dynamic modelling in systems biology applications.
View details
Exchangeability and the law of maturity
Rafael B. Stern
Claudia M. Peixoto
Sergio Wechsler
Theory and Decision(2014), pp. 1-13
Preview abstract
The law of maturity is the belief that less-observed events are becoming mature and, therefore, more likely to occur in the future. Previous studies have shown that the assumption of infinite exchangeability contradicts the law of maturity. In particular, it has been shown that infinite exchangeability contradicts probabilistic descriptions of the law of maturity such as the gambler’s belief and the belief in maturity. We show that the weaker assumption of finite exchangeability is compatible with both the gambler’s belief and belief in maturity. We provide sufficient conditions under which these beliefs hold under finite exchangeability. These conditions are illustrated with commonly used parametric models.
View details
Bayesian Learning from Marginal Data in Bionetwork Models
Lingchong You
Mike West
Statistical Applications in Genetics and Molecular Biology, 10(2011)
In Defense of Randomization: a Subjectivist Bayesian Approach
Raphael Nishimura
Rafael Bassi Stern
AIP Conference Proceedings(2009), pp. 32-39
Preview abstract
In research situations usually approached by Decision Theory, it is only considered one researcher who collects a sample and makes a decision based on it. It can be shown that randomization of the sample does not improve the utility of the obtained results. Nevertheless, we present situations in which this approach is not satisfactory. First, we present a case in which randomization can be an important tool in order to achieve agreement between people with different opinions. Next, we present another situation in which there are two agents: the researcher—a person who collects the sample; and the decision‐maker—a person who makes decisions based on the sample collected. We show that problems emerge when the decision‐maker allows the researcher to arbitrarily choose a sample. We also show that the decision‐maker maximizes his expected utility requiring that the sample is collected randomly.
View details