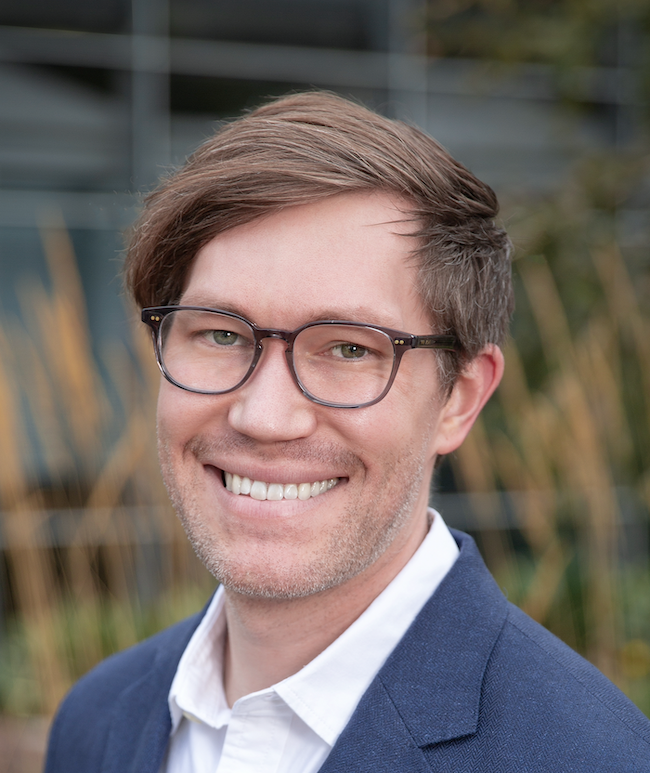
Drew Bryant
Research Areas
Authored Publications
Sort By
Longitudinal fundus imaging and its genome-wide association analysis provides evidence for a human retinal aging clock
Sara Ahadi
Kenneth A Wilson Jr,
Orion Pritchard
Ajay Kumar
Enrique M Carrera
Ricardo Lamy
Jay M Stewart
Avinash Varadarajan
Pankaj Kapahi
Ali Bashir
eLife(2023)
Preview abstract
Background
Biological age, distinct from an individual’s chronological age, has been studied extensively through predictive aging clocks. However, these clocks have limited accuracy in short time-scales. Deep learning approaches on imaging datasets of the eye have proven powerful for a variety of quantitative phenotype inference and provide an opportunity to explore organismal aging and tissue health.
Methods
Here we trained deep learning models on fundus images from the EyePacs dataset to predict individuals’ chronological age. These predictions lead to the concept of a retinal aging clock which we then employed for a series of downstream longitudinal analyses. The retinal aging clock was used to assess the predictive power of aging inference, termed eyeAge, on short time-scales using longitudinal fundus imaging data from a subset of patients. Additionally, the model was applied to a separate cohort from the UK Biobank to validate the model and perform a GWAS. The top candidate gene was then tested in a fly model of eye aging.
Findings
EyeAge was able to predict the age with a mean absolute error of 3.26 years, which is much less than other aging clocks. Additionally, eyeAge was highly independent of blood marker-based measures of biological age (e.g. “phenotypic age”), maintaining a hazard ratio of 1.026 even in the presence of phenotypic age. Longitudinal studies showed that the resulting models were able to predict individuals’ aging, in time-scales less than a year with 71% accuracy. Notably, we observed a significant individual-specific component to the prediction. This observation was confirmed with the identification of multiple GWAS hits in the independent UK Biobank cohort. The knockdown of the top hit, ALKAL2, which was previously shown to extend lifespan in flies, also slowed age-related decline in vision in flies.
Interpretation
In conclusion, predicted age from retinal images can be used as a biomarker of biological aging in a given individual independently from phenotypic age. This study demonstrates the utility of retinal aging clock for studying aging and age-related diseases and quantitatively measuring aging on very short time-scales, potentially opening avenues for quick and actionable evaluation of gero-protective therapeutics.
View details
Deep diversification of an AAV capsid protein by machine learning
Ali Bashir
Sam Sinai
Nina K. Jain
Pierce J. Ogden
Patrick F. Riley
George M. Church
Eric D. Kelsic
Nature Biotechnology(2021)
Preview abstract
Modern experimental technologies can assay large numbers of biological sequences, but engineered protein libraries rarely exceed the sequence diversity of natural protein families. Machine learning (ML) models trained directly on experimental data without biophysical modeling provide one
route to accessing the full potential diversity of engineered proteins. Here we apply deep learning to design highly diverse adeno-associated virus 2 (AAV2) capsid protein variants that remain viable for packaging of a DNA payload. Focusing on a 28-amino acid segment, we generated 201,426 variants of the AAV2 wild-type (WT) sequence yielding 110,689 viable engineered capsids, 57,348 of which surpass the average diversity of natural AAV serotype sequences, with 12–29 mutations across this region. Even when trained on limited data, deep neural network models accurately predict capsid viability across diverse variants. This approach unlocks vast areas of functional but previously unreachable sequence space, with many potential applications for the generation of improved
viral vectors and protein therapeutics.
View details
Critiquing Protein Family Classification Models Using Sufficient Input Subsets
Brandon Michael Carter
Jamie Alexander Smith
Theo Sanderson
ACM SIGKDD Conference on Knowledge Discovery and Data Mining(2019) (to appear)
Preview abstract
In many application domains, neural networks are highly accurate and have been deployed at large scale. However, users often do not have good tools for understanding how these models arrive at their predictions. This has hindered adoption in fields such as the life and medical sciences, where researchers require that models base their decisions on underlying biological phenomena rather than peculiarities of the dataset introduced, e.g., as a function of when and how the data were collected. In response, we propose a set of methods for critiquing deep learning models, and demonstrate their application for protein family classification, a task for which high- accuracy models have considerable potential impact. Our methods extend the recently-introduced sufficient input subsets technique (SIS), which we use to identify the subset of locations (SIS) in each protein sequence that is sufficient for classification. Our suite of tools analyzes these SIS to shed light on the decision making criteria employed by models trained on this task. These tools expose that while these deep models may perform classification for biologically-relevant reasons, their behavior varies considerably across choice of network architecture and parameter initialization. While the techniques that we develop are specific to the protein sequence classification task, the approach taken generalizes to a broad set of scientific contexts in which model interpretability is essential. We encourage further application of our techniques for interrogating machine learning models trained on other scientifically relevant tasks.
View details
Deep Learning Classifies the Protein Universe
Theo Sanderson
Brandon Carter
Mark DePristo
Nature Biotechnology(2019)
Preview abstract
Understanding the relationship between amino acid sequence and protein function is a long-standing problem in molecular biology with far-reaching scientific implications. Despite six decades of progress, state-of-the-art techniques cannot annotate $\sim1/3$ of microbial protein sequences, hampering our ability to exploit sequences collected from diverse organisms. To address this, we report a deep learning model that learns the relationship between unaligned amino acid sequences and their functional classification across all 17929 families of the PFam database. Using the Pfam seed sequences we establish a rigorous benchmark assessment and find that a dilated convolutional model reduces the error of state of the art BLASTp and pHMM models by a factor of nine. With 80\% of the full Pfam database we train a protein family predictor that is more accurate and over 200 times faster than BLASTp, while learning sequence features such as structural disorder and transmembrane helices. Our model co-locates sequences from unseen families in embedding space far from existing families, allowing sequences from novel families to be classified. We anticipate that deep learning models will be a core component of future general-purpose protein function prediction tools.
View details
Preview abstract
Low-carbon electricity technologies are often evaluated by their Levelized
Cost of Energy (LCOE). However, LCOE cannot model the impact of one
electricity source on the value of others. In previous work, System LCOE
was proposed to estimate the costs of integrating an intermittent source
into a grid consisting of multiple dispatchable electricity sources.
Using a new DOSCOE (Dispatch-optimized system cost of electricity) model,
we generalize System LCOE. DOSCOE can handle any mixture of dispatchable
and non-dispatchable sources. It can analyze systems which contain storage,
have legacy infrastructure, or have imposed policies. DOSCOE thus updates
System LCOE to be applicable to more realistic electricity grid models.
DOSCOE uses a linear program to find the capacity and generation mix which
yields minimum LCOE. Running this linear program multiple times yields
System LCOE curves.
DOSCOE shows that to cost-effectively remove the last 10-20% of fossil
fuels requires a moderate price on carbon and either low-cost nuclear power
or carbon capture and sequestration. Alternatively, a hypothetical zero-carbon
source needs to have a net present cost less than $2200/kW to displace existing
fossil-fuel plants.
View details