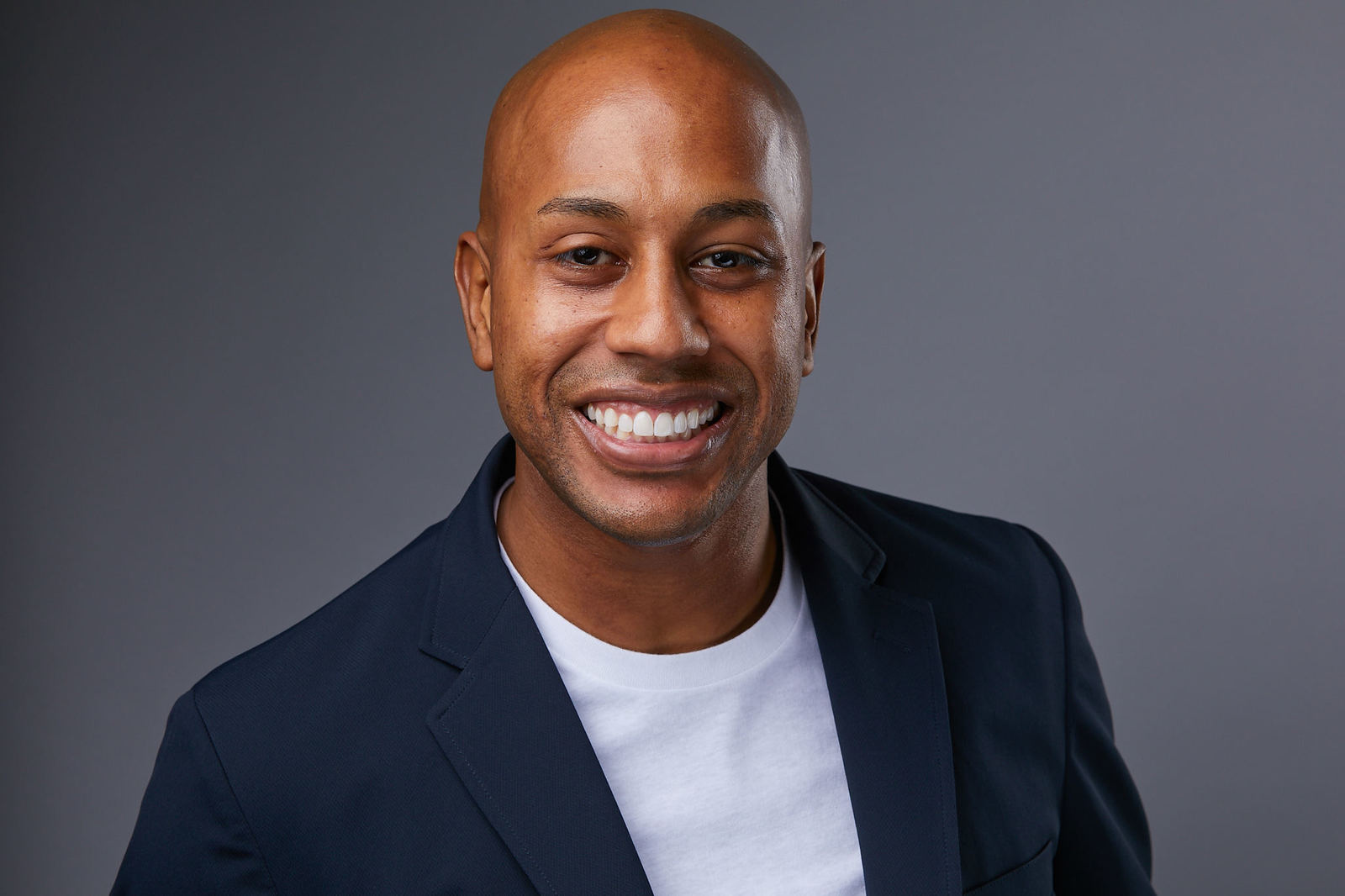
Andrew Zaldivar
Andrew Zaldivar is a Senior Developer Advocate for Google. His job is to help to bring the benefits of AI to everyone. Andrew develops, evaluates, and promotes tools and techniques that can help communities build socially responsible AI systems, writing posts for the Google Developers blog and speaking at a variety of conferences.
Before joining Google AI, Andrew was a Senior Strategist in Google’s Trust & Safety group. He worked on protecting the integrity of some of Google’s key products by using machine learning to scale, optimize and automate abuse-fighting efforts.
Prior to joining Google, Andrew completed his Ph.D. in Cognitive Neuroscience from the University of California, Irvine and was an Insight Data Science fellow.
Authored Publications
Sort By
Data Cards: Purposeful and Transparent Dataset Documentation for Responsible AI
Association for Computing MachineryNew YorkNYUnited States(2022)
Preview abstract
As we move towards large-scale models capable of numerous downstream tasks, the complexity of understanding multi-modal datasets that give nuance to models rapidly increases. A clear and thorough understanding of a dataset's origins, development, intent, ethical considerations and evolution becomes a necessary step for the responsible and informed deployment of models, especially those in people-facing contexts and high-risk domains. However, the burden of this understanding often falls on the intelligibility, conciseness, and comprehensiveness of its documentation. However, the burden of this understanding often falls on the intelligibility, conciseness, and comprehensiveness of the documentation, and consistency and comparability across the documentation of all datasets involved, and as such documentation must be treated as a user-centric product in and of itself. In this paper, we propose Data Cards for fostering transparent, purposeful and human-centered documentation of datasets within the practical contexts of industry and research. Data Cards are structured summaries of essential facts about various aspects of ML datasets needed by stakeholders across a dataset's lifecycle for responsible AI development. These summaries provide explanations of processes and rationales that shape the data and consequently the models—such as upstream sources, data collection and annotation methods; training and evaluation methods, intended use, or decisions affecting model performance. We also present evaluative frameworks that ground Data Cards in real-world utility and human-centricity. Using two case studies, we report on desirable characteristics that support adoption across domains, organizational structures, and audience groups. Finally, we present lessons learned from deploying over twenty Data Cards.
View details
Model Cards for Model Reporting
Elena Spitzer
Inioluwa Deborah Raji
M. Mitchell
Simone Sanoian McCloskey Wu
Timnit Gebru
(2019)
Preview abstract
Trained machine learning models are increasingly used to perform high impact tasks such as determining crime recidivism rates and predicting health risks. In order to clarify the intended use cases of machine learning models and minimize their usage in contexts they are not well-suited for, we recommend that released models be accompanied by documentation detailing their performance characteristics. In this paper, we propose a framework that we call model cards (or M-cards) to encourage such transparent model reporting. Model cards are short documents accompanying trained machine learning models that provide benchmarked evaluation in a variety of conditions, such as across different cultural, demographic, or phenotypic subgroups (e.g., race, geographic location, sex, Fitzpatrick skin tone) and intersectional subgroups (e.g., age and race, or sex and Fitzpatrick skin tone) that are relevant to the intended application domains. Model cards also disclose the context under which models are intended to be used, details of the performance evaluation procedures, and other relevant information. While we focus primarily on human-centered machine learning models in the application fields of computer vision and natural language processing, this framework can be used to document any trained machine learning model. To solidify the concept, we provide cards for models trained to detect smiling faces on the CelebA dataset (Liu et al., 2015) and models trained to detect toxicity in the Conversation AI dataset (Dixon et al., 2018). We propose this work as a step towards the responsible democratization of machine learning and related AI technology, providing context around machine learning models and increasing the transparency into how well such models work. We hope this work encourages those releasing trained machine learning models to accompany model releases with similar detailed documentation.
View details