Language
We advance the state of the art in natural language technologies and build systems that learn to understand and generate language in context.
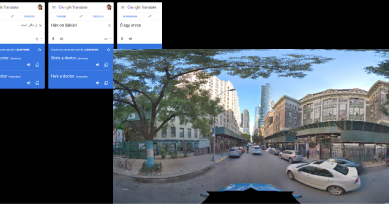
We advance the state of the art in natural language technologies and build systems that learn to understand and generate language in context.
About the team
Our team comprises multiple research groups working on a wide range of natural language understanding and generation projects. We pursue long-term research to develop novel capabilities that can address the needs of current and future Google products. We publish frequently and evaluate our methods on established scientific benchmarks (e.g., SQuAD, GLUE, SuperGlue) or develop new ones for measuring progress (e.g., Conceptual Captions, Natural Questions, TyDiQA). We collaborate with other teams across Google to deploy our research to the benefit of our users. Our product contributions often stretch the boundaries of what is technically possible. Applications of our research have resulted in better language capabilities across all major Google products.
Our researchers are experts in natural language processing and machine learning with varied backgrounds and a passion for language. Computer scientists and linguists work hand-in-hand to provide insight into ways to define language tasks, collect valuable data, and assist in enabling internationalization. Researchers and engineers work together to develop new neural network models that are sensitive to the nuances of language while taking advantage of the latest advances in specialized compute hardware (e.g., TPUs) to produce scalable solutions that can be used by billions of users.
Team focus summaries
Learn contextual language representations that capture meaning at various levels of granularity and are transferable across tasks.
Learn end-to-end models for real world question answering that requires complex reasoning about concepts, entities, relations, and causality in the world.
Learn document representations from geometric features and spatial relations, multi-modal content features, syntactic, semantic and pragmatic signals.
Advance next generation dialogue systems in human-machine and multi-human-machine interactions to achieve natural user interactions and enrich conversations between human users.
Produce natural and fluent output for spoken and written text for different domains and styles.
Learning high-quality models that scale to all languages and locales and are robust to multilingual inputs, transliterations, and regional variants.
Understand visual inputs (image & video) and express that understanding using fluent natural language (phrases, sentences, paragraphs).
Use state-of-the-art machine learning techniques and large-scale infrastructure to break language barriers and offer human quality translations across many languages to make it possible to easily explore the multilingual world.
Learn to summarize single and multiple documents into cohesive and concise summaries that accurately represent the documents.
Learn end-to-end models that classify the semantics of text, such as topic, sentiment or sensitive content (i.e., offensive, inappropriate, or controversial content).
Represent, combine, and optimize models for speech to text and text to speech.
Learn models that infer entities (people, places, things) from text and that can perform reasoning based on their relationships.
Use and learn representations that span language and other modalities, such as vision, space and time, and adapt and use them for problems requiring language-conditioned action in real or simulated environments (i.e., vision-and-language navigation).
Learn models for predicting executable logical forms given text in varying domains and languages, situated within diverse task contexts.
Learn models that can detect sentiment attribution and changes in narrative, conversation, and other text or spoken scenarios.
Learn models of language that are predictable and understandable, perform well across the broadest possible range of linguistic settings and applications, and adhere to our principles of responsible practices in AI.
Featured publications
Highlighted work
-
An NLU-Powered Tool to Explore COVID-19 Scientific LiteratureGoogle Research launches a NLU-powered tool that provides answers and evidence to complex scientific questions about COVID-19.
-
Using Neural Networks to Find Answers in TablesNeural networks enable people to use natural language to get questions answered from information stored in tables.
-
A Scalable Approach to Reducing Gender Bias in Google TranslateWe implemented an improved approach to reducing gender bias in Google Translate that uses a dramatically different paradigm to address gender bias by rewriting or post-editing the initial translation.
-
XTREME: A Massively Multilingual Multi-task Benchmark for Evaluating Cross-lingual GeneralizationTo encourage more research on multilingual learning, we introduce “XTREME: A Massively Multilingual Multi-task Benchmark for Evaluating Cross-lingual Generalization”, which covers 40 typologically diverse languages (spanning 12 language families) and includes nine tasks that collectively require reasoning about different levels of syntax or semantics.
-
Enhancing the Research Community's Access to Street View Panoramas for Language Grounding TasksWe add the Street View panoramas referenced in the Touchdown dataset to the existing StreetLearn dataset to support the broader community's ability to use Touchdown for researching vision and language navigation and spatial description resolution in Street view settings.
-
TyDi QA: A Multilingual Question Answering BenchmarkTo encourage research on multilingual question-answering, we released TyDi QA, a question answering corpus covering 11 Typologically Diverse languages
-
Encode, Tag and Realize: A Controllable and Efficient Approach for Text GenerationWe present a novel, open sourced method for text generation that is less error-prone and can be handled by easier to train and faster to execute model architectures.
-
ALBERT: A Lite BERT for Self-Supervised Learning of Language RepresentationsALBERT is an upgrade to BERT that advances the state-of-the-art performance on 12 NLP tasks, including the competitive Stanford Question Answering Dataset (SQuAD v2.0) and the SAT-style reading comprehension RACE benchmark.
-
Releasing PAWS and PAWS-X: Two New Datasets to Improve Natural Language UnderstandingWe are released two new datasets for use in the research community: Paraphrase Adversaries from Word Scrambling (PAWS) in English, and PAWS-X", an extension of the PAWS dataset to six "typologically distinct languages: French, Spanish, German, Chinese, Japanese, and Korean".
-
Robust Neural Machine TranslationIn "Robust Neural Machine Translation with Doubly Adversarial Inputs" (ACL 2019), we propose an approach that uses generated adversarial examples to improve the stability of machine translation models against small perturbations in the input.
-
Multilingual Universal Sentence Encoder for Semantic RetrievalWe released three new Universal Sentence Encoder multilingual modules with additional features and potential applications.
-
Natural Questions: a New Corpus and Challenge for Question Answering ResearchTo help spur research advances in question answering, we released Natural Questions, a new, large-scale corpus for training and evaluating open-domain question answering systems, and the first to replicate the end-to-end process in which people find answers to questions.
Some of our locations
Some of our people
-
Ankur Parikh
- Natural Language Processing
-
Bo Pang
- Natural Language Processing
-
Colin Cherry
- Machine Translation
- Natural Language Processing
-
Dipanjan Das
- Machine Intelligence
- Natural Language Processing
-
Fernando Pereira
- Machine Intelligence
- Natural Language Processing
-
Filip Radlinski
- Information Retrieval and the Web
- Machine Intelligence
- Natural Language Processing
-
Jacob Eisenstein
- Natural Language Processing
-
Jason Baldridge
- Natural Language Processing
-
Katja Filippova
- Machine Intelligence
- Natural Language Processing
-
Kellie Webster
- Machine Intelligence
- Machine Translation
- Natural Language Processing
-
Kenton Lee
- Machine Intelligence
- Natural Language Processing
-
Kristina N Toutanova
- Machine Intelligence
- Natural Language Processing
-
Luheng He
- Natural Language Processing
-
Massimiliano Ciaramita
- Data Mining and Modeling
- Information Retrieval and the Web
- Natural Language Processing
-
Melvin Johnson
- Machine Translation
- Natural Language Processing
-
Michael Collins
- Machine Learning
- Natural Language Processing
-
Orhan Firat
- Machine Intelligence
- Machine Translation
-
Radu Soricut
- General Science
- Machine Intelligence
- Machine Translation
-
Slav Petrov
- General Science
- Machine Intelligence
- Machine Translation
-
Srini Narayanan
- Machine Intelligence
- Natural Language Processing
-
Tania Bedrax-Weiss
- Machine Intelligence
- Machine Perception
- Natural Language Processing
-
William W. Cohen
- Information Retrieval and the Web
- Machine Intelligence
- Natural Language Processing
-
Wolfgang Macherey
- Machine Translation
- Natural Language Processing
-
Yasemin Altun
- Natural Language Processing
-
Yun-hsuan Sung
- Machine Intelligence
- Natural Language Processing
- Speech Processing