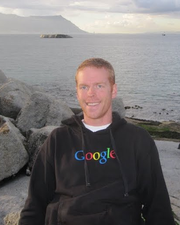
Tipp Moseley
Tipp Moseley has been at Google since 2009 working on warehouse-scale optimization, including profilers, compilers, architecture, and performance analysis. He received a Ph.D. in Computer Science from the University of Colorado at Boulder and a B.S. in Computer Science from Georgia Tech. He also served as a postdoctoral researcher at Université de Versailles. Outside work, Tipp has 3 hobbies, ages 2, 3, and 5 at the time of writing.
Research Areas
Authored Publications
Sort By
Characterizing a Memory Allocator at Warehouse Scale
Zhuangzhuang Zhou
Nilay Vaish
Patrick Xia
Christina Delimitrou
Proceedings of the 29th ACM International Conference on Architectural Support for Programming Languages and Operating Systems, Volume 3, Association for Computing Machinery, La Jolla, CA, USA(2024), 192–206
Preview abstract
Memory allocation constitutes a substantial component of warehouse-scale computation. Optimizing the memory allocator not only reduces the datacenter tax, but also improves application performance, leading to significant cost savings.
We present the first comprehensive characterization study of TCMalloc, a warehouse-scale memory allocator used in our production fleet. Our characterization reveals a profound diversity in the memory allocation patterns, allocated object sizes and lifetimes, for large-scale datacenter workloads, as well as in their performance on heterogeneous hardware platforms. Based on these insights, we redesign TCMalloc for warehouse-scale environments. Specifically, we propose optimizations for each level of its cache hierarchy that include usage-based dynamic sizing of allocator caches, leveraging hardware topology to mitigate inter-core communication overhead, and improving allocation packing algorithms based on statistical data. We evaluate these design choices using benchmarks and fleet-wide A/B experiments in our production fleet, resulting in a 1.4% improvement in throughput and a 3.4% reduction in RAM usage for the entire fleet. At our scale, even a single percent CPU or memory improvement translates to significant savings in server costs.
View details
EMISSARY: Enhanced Miss Awareness Replacement Policy for L2 Instruction Caching
Nayana Prasad Nagendra
Bhargav Reddy Godala
Ishita Chaturvedi
Atmn Patel
Jared Stark
Gilles A. Pokam
Simone Campanoni
David I. August
Proceedings of the 50th Annual International Symposium on Computer Architecture (ISCA)(2023)
Preview abstract
For decades, architects have designed cache replacement policies to reduce cache misses. Since not all cache misses affect processor performance equally, researchers have also proposed cache replacement policies focused on reducing the total miss cost rather than the total miss count. However, all prior cost-aware replacement policies have been proposed specifically for data caching and are either inappropriate or unnecessarily complex for instruction caching. This paper presents EMISSARY, the first cost-aware cache replacement family of policies specifically designed for instruction caching. Observing that modern architectures entirely tolerate many instruction cache misses, EMISSARY resists evicting those cache lines whose misses cause costly decode starvations. In the context of a modern processor with fetch-directed instruction prefetching and other aggressive front-end features, EMISSARY applied to L2 cache instructions delivers an impressive 3.24% geomean speedup (up to 23.7%) and a geomean energy savings of 2.1% (up to 17.7%) when evaluated on widely used server applications with large code footprints. This speedup is 21.6% of the total speedup obtained by an unrealizable L2 cache with a zero-cycle miss latency for all capacity and conflict instruction misses.
View details
Beyond malloc efficiency to fleet efficiency: a hugepage-aware memory allocator
Andrew Hamilton Hunter
15th USENIX Symposium on Operating Systems Design and Implementation (OSDI 21)(2021) (to appear)
Preview abstract
Memory allocation represents significant compute cost at the warehouse scale and its optimization can yield considerable cost savings. One classical approach is to increase the efficiency of an allocator to minimize the cycles spent in the allocator code. However, memory allocation decisions also impact overall application performance via data placement, offering opportunities to improve fleetwide productivity by completing more units of application work using fewer hardware resources. Here, we focus on hugepage coverage. We present TEMERAIRE, a hugepage-aware enhancement of TCMALLOC to reduce CPU overheads in the application’s code. We discuss the design and implementation of TEMERAIRE including strategies for hugepage-aware memory layouts to maximize hugepage coverage and to minimize fragmentation overheads. We present application studies for 8 applications, improving requests-per-second (RPS) by 7.7% and reducing RAM usage 2.4%. We present the results of a 1% experiment at fleet scale as well as the longitudinal rollout in Google’s warehouse scale computers. This yielded 6% fewer TLB miss stalls, and 26% reduction in memory wasted due to fragmentation. We conclude with a discussion of additional techniques for improving the allocator development process and potential optimization strategies for future memory allocators.
View details
Break Dancing: low overhead, architecture agnostic software branch tracing
22nd ACM SIGPLAN/SIGBED International Conference on Languages, Compilers, and Tools for Embedded Systems (LCTES ’21)(2021)
Preview abstract
Sampling-based Feedback Directed Optimization (FDO) methods like AutoFDO and BOLT that employ profiles continuously collected in live production environments, are commonly used in datacenter applications to attain significant performance benefits without the toil of maintaining representative load tests. Sampled profiles rely on hardware facilities like Intel’s Last Branch Record (LBR) which are not currently available even on popular CPUs from ARM or AMD. Since not all architectures include a hardware LBR feature, we present an architecture agnostic approach to collect LBR-like data. We use sampling and limited program tracing to capture LBR like data from optimized and unmodified applications binaries. Since the implementation is in user space, we can collect arbitrarily long LBR buffers, and by varying the sampling rate, we can adjust the runtime overhead to arbitrarily low values. We target runtime overheads of <2% when the profiler is on and zero when it’s off. This amortizes to negligible fleet-wide collection cost given the size of a modern production fleet.
We implemented a profiler that uses this method of software branch tracing. We also analyzed its overhead and the similarity of the data it collects to the Intel LBR hardware using the SPEC2006 benchmarks. Results demonstrate profile quality and optimization efficacy at parity with LBR-based AutoFDO and the target profiling overhead being achievable even without implementing any advanced tuning.
View details
AsmDB: Understanding and Mitigating Front-End Stalls in Warehouse-Scale Computers
Nayana Prasad Nagendra
David I. August
Christos Kozyrakis
Trivikram Krishnamurthy
Heiner Litz
International Symposium on Computer Architecture (ISCA)(2019)
Preview abstract
The large instruction working sets of private and public cloud workloads lead to frequent instruction cache misses and costs in the millions of dollars. While prior work has identified the growing importance of this problem, to date, there has been little analysis of where the misses come from, and what the opportunities are to improve them. To address this challenge, this paper makes three contributions. First, we present the design and deployment of a new, always-on, fleet-wide monitoring system, AsmDB, that tracks front-end bottlenecks. AsmDB uses hardware support to collect bursty execution traces, fleet-wide temporal and spatial sampling, and sophisticated offline post-processing to construct full-program dynamic control-flow graphs. Second, based on a longitudinal analysis of AsmDB data from real-world online services, we present two detailed insights on the sources of front-end stalls: (1) cold code that is brought in along with hot code leads to significant cache fragmentation and a corresponding large number of instruction cache misses; (2) distant branches and calls that are not amenable to traditional cache locality or next-line prefetching strategies account for a large fraction of cache misses. Third, we prototype two optimizations that target these insights. For misses caused by fragmentation, we focus on memcmp, one of the hottest functions contributing to cache misses, and show how fine-grained layout optimizations lead to significant benefits. For misses at the targets of distant jumps, we propose new hardware support for software code prefetching and prototype a new feedback-directed compiler optimization that combines static program flow analysis with dynamic miss profiles to demonstrate significant benefits for several large warehouse-scale workloads. Improving upon prior work, our proposal avoids invasive hardware modifications by prefetching via software in an efficient and scalable way. Simulation results show that such an approach can eliminate up to 96% of instruction cache misses with negligible overheads.
View details
AutoFDO: Automatic Feedback-Directed Optimization for Warehouse-Scale Applications
Dehao Chen
CGO 2016 Proceedings of the 2016 International Symposium on Code Generation and Optimization, ACM, New York, NY, USA, pp. 12-23
Preview abstract
AutoFDO is a system to simplify real-world deployment of feedback-directed optimization (FDO). The system works by sampling hardware performance monitors on production machines and using those profiles to guide optimization. Profile data is stale by design, and we have implemented compiler features to deliver stable speedup across releases. The resulting performance is a geomean of 10.5% improvement on our benchmarks. AutoFDO achieves 85% of the gains of traditional FDO, despite imprecision due to sampling and information lost in the compilation pipeline. The system is deployed to hundreds of binaries at Google, and it is extremely easy to enable; users need only to add some flags to their release build. To date, AutoFDO has increased the number of FDO users at Google by 8X and has doubled the number of cycles spent in FDO-optimized binaries. Over half of CPU cycles used are now spent in some flavor of FDO-optimized binaries.
View details
Profiling a warehouse-scale computer
Juan Darago
Kim Hazelwood
Gu-Yeon Wei
David Brooks
ISCA '15 Proceedings of the 42nd Annual International Symposium on Computer Architecture, ACM(2014), pp. 158-169
Preview abstract
With the increasing prevalence of warehouse-scale (WSC) and cloud computing, understanding the interactions of server applications with the underlying microarchitecture becomes ever more important in order to extract maximum performance out of server hardware. To aid such understanding, this paper presents a detailed microarchitectural analysis of live datacenter jobs, measured on more than 20,000 Google machines over a three year period, and comprising thousands of different applications.
We first find that WSC workloads are extremely diverse, breeding the need for architectures that can tolerate application variability without performance loss. However, some patterns emerge, offering opportunities for co-optimization of hardware and software. For example, we identify common building blocks in the lower levels of the software stack. This "datacenter tax" can comprise nearly 30% of cycles across jobs running in the fleet, which makes its constituents prime candidates for hardware specialization in future server systems-on-chips. We also uncover opportunities for classic microarchitectural optimizations for server processors, especially in the cache hierarchy. Typical workloads place significant stress on instruction caches and prefer memory latency over bandwidth. They also stall cores often, but compute heavily in bursts. These observations motivate several interesting directions for future warehouse-scale computers.
View details
Instant Profiling: Instrumentation Sampling for Profiling Datacenter Applications
Preview
Hyoun Kyu Cho
Rick Hank
Scott A. Mahlke
Proceedings of the 2013 IEEE/ACM International Symposium on Code Generation and Optimization (CGO), IEEE Computer Society, Washington, DC, USA
Preview abstract
Google-Wide Profiling (GWP), a continuous profiling infrastructure for data centers, provides performance insights for cloud applications. With negligible overhead, GWP provides stable, accurate profiles and a datacenter-scale tool for traditional performance analyses. Furthermore, GWP introduces novel applications of its profiles, such as application- platform affinity measurements and identification of platform-specific, microarchitectural peculiarities.
View details