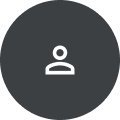
Shen Yan
Research Areas
Authored Publications
Google Publications
Other Publications
Sort By
UnLoc: a unified framework for video localization tasks
Xuehan Xiong
Anurag Arnab
Zhonghao Wang
Weina Ge
International Conference on Computer Vision (2023)
Preview abstract
We adapt large-scale image-text pretrained models such as CLIP for temporal localization tasks in untrimmed videos, which is still a relatively unexplored task. We do so by designing a new approach called UnLoc, which uses a pretrained image and text tower, and feeds tokens to a video-text fusion model. The output of the fusion module are then used to construct a feature pyramid in which each level connects to a head to predict a per-frame relevancy score and start/end time displacements. Unlike previous works, our architecture enables zero-shot Moment Retrieval, TAL and action segmentation with a single stage model, without the need for action proposals or representation masking. Unlike specialised models, we achieve state of the art results on three different localization tasks with a unified approach - in some cases outperforming previous works by large margins.
View details
VideoCoCa: Video-Text Modeling with Zero-Shot Transfer from Contrastive Captioners
Tao Zhu
Zirui Wang
Mi Zhang
Soham Ghosh
Jiahui Yu
arxiv.org, Cornell University (2023)
Preview abstract
We explore an efficient approach to establish a foundational video-text model. We present VideoCoCa that maximally reuses a pretrained image-text contrastive captioner (CoCa) model and adapt it to video-text tasks with minimal extra training. While previous works adapt image-text models with various cross-frame fusion modules, we find that the generative attentional pooling and contrastive attentional pooling layers in CoCa are instantly adaptable to flattened frame embeddings, yielding state-of-the-art results on zero-shot video classification and zero-shot text-to-video retrieval. Furthermore, we explore lightweight finetuning on top of VideoCoCa, and achieve strong results on video question-answering and video captioning.
View details
Multiview Transformers for Video Recognition
Xuehan Xiong
Anurag Arnab
Zhichao Lu
Mi Zhang
The IEEE / CVF Computer Vision and Pattern Recognition Conference (CVPR) (2022)
Preview abstract
Video understanding often requires reasoning at multiple spatiotemporal resolutions.
To this end, we present Multiview Transformers for Video Recognition (MTV).
Our model consists of separate encoders to represent different views of the input video with lateral connections to fuse information across views.
MTV consistently performs better than single-view counterparts in terms of accuracy and computational cost across a range of model sizes, and can effectively leverage different transformer encoder architectures.
We present thorough ablation studies of our model and achieve state-of-the-art results on five standard datasets.
We will release code and pretrained checkpoints to facilitate further research.
View details
No Results Found