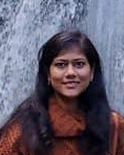
Priyanka Agrawal
Priyanka is a Research Scientist at Google Research, London. She is a part of natural language generation team where she is working on scaling generation models to under-represented languages under low-resource settings. Prior to Google, she was an ML scientist with Booking.com and a researcher at IBM Research Lab. More info on her homepage.
Research Areas
Authored Publications
Google Publications
Other Publications
Sort By
QAmeleon: Multilingual QA with Only 5 Examples
Fantine Huot
Sebastian Ruder
Mirella Lapata
Arxiv (2022)
Preview abstract
The availability of large, high-quality datasets has been one of the main drivers of recent progress in question answering (QA). Such annotated datasets however are difficult and costly to collect, and rarely exist in languages other than English, rendering QA technology inaccessible to underrepresented languages. An alternative to building large monolingual training datasets is to leverage pre-trained language models (PLMs) under a few-shot learning setting. Our approach, QAmeleon, uses a PLM to automatically generate multilingual data upon which QA models are trained, thus avoiding costly annotation. Prompt tuning the PLM for data synthesis with only five examples per language delivers accuracy superior to translation-based baselines, bridges nearly 60% of the gap between an English-only baseline and a fully supervised upper bound trained on almost 50,000 hand labeled examples, and always leads to substantial improvements compared to fine-tuning a QA model directly on labeled examples in low resource settings. Experiments on the TyDiQA-GoldP and MLQA benchmarks show that few-shot prompt tuning for data synthesis scales across languages and is a viable alternative to large-scale annotation.
View details
No Results Found